Does Recommend-Revise Produce Reliable Annotations? An Analysis on Missing Instances in DocRED
PROCEEDINGS OF THE 60TH ANNUAL MEETING OF THE ASSOCIATION FOR COMPUTATIONAL LINGUISTICS (ACL 2022), VOL 1: (LONG PAPERS)(2022)
摘要
DocRED is a widely used dataset for document-level relation extraction. In the large-scale annotation, a recommend-revise scheme is adopted to reduce the workload. Within this scheme, annotators are provided with candidate relation instances from distant supervision, and they then manually supplement and remove relational facts based on the recommendations. However, when comparing DocRED with a subset relabeled from scratch, we find that this scheme results in a considerable amount of false negative samples and an obvious bias towards popular entities and relations. Furthermore, we observe that the models trained on DocRED have low recall on our relabeled dataset and inherit the same bias in the training data. Through the analysis of annotators' behaviors, we figure out the underlying reason for the problems above: the scheme actually discourages annotators from supplementing adequate instances in the revision phase. We appeal to future research to take into consideration the issues with the recommend-revise scheme when designing new models and annotation schemes. The relabeled dataset is released at https://github.com/AndrewZhe/Revisit-DocRED, to serve as a more reliable test set of document RE models.
更多查看译文
关键词
missing instances,recommend-revise
AI 理解论文
溯源树
样例
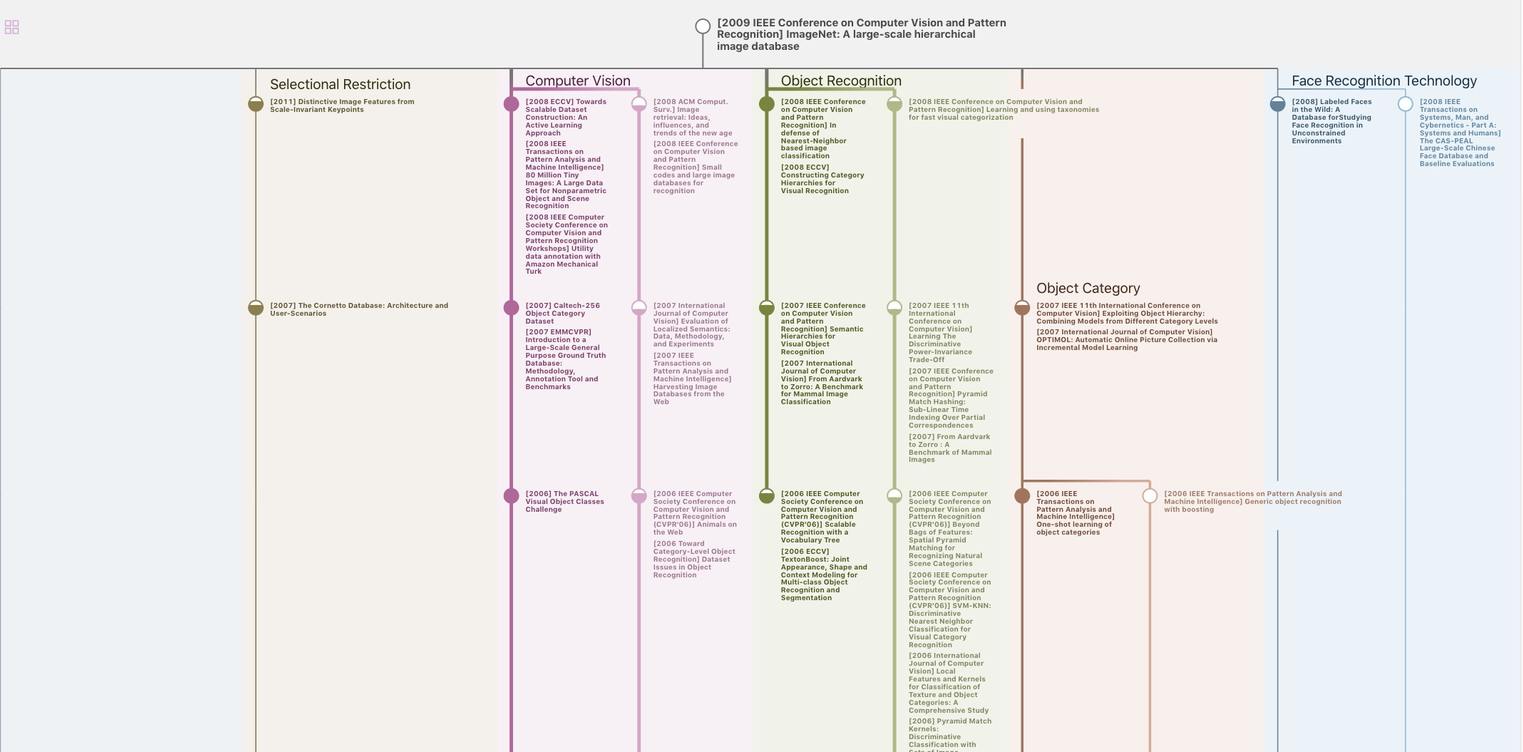
生成溯源树,研究论文发展脉络
Chat Paper
正在生成论文摘要