Stretching Sentence-pair NLI Models to Reason over Long Documents and Clusters
arxiv(2022)
摘要
Natural Language Inference (NLI) has been extensively studied by the NLP community as a framework for estimating the semantic relation between sentence pairs. While early work identified certain biases in NLI models, recent advancements in modeling and datasets demonstrated promising performance. In this work, we further explore the direct zero-shot applicability of NLI models to real applications, beyond the sentence-pair setting they were trained on. First, we analyze the robustness of these models to longer and out-of-domain inputs. Then, we develop new aggregation methods to allow operating over full documents, reaching state-of-the-art performance on the ContractNLI dataset. Interestingly, we find NLI scores to provide strong retrieval signals, leading to more relevant evidence extractions compared to common similarity-based methods. Finally, we go further and investigate whole document clusters to identify both discrepancies and consensus among sources. In a test case, we find real inconsistencies between Wikipedia pages in different languages about the same topic.
更多查看译文
关键词
long documents,clusters,sentence-pair
AI 理解论文
溯源树
样例
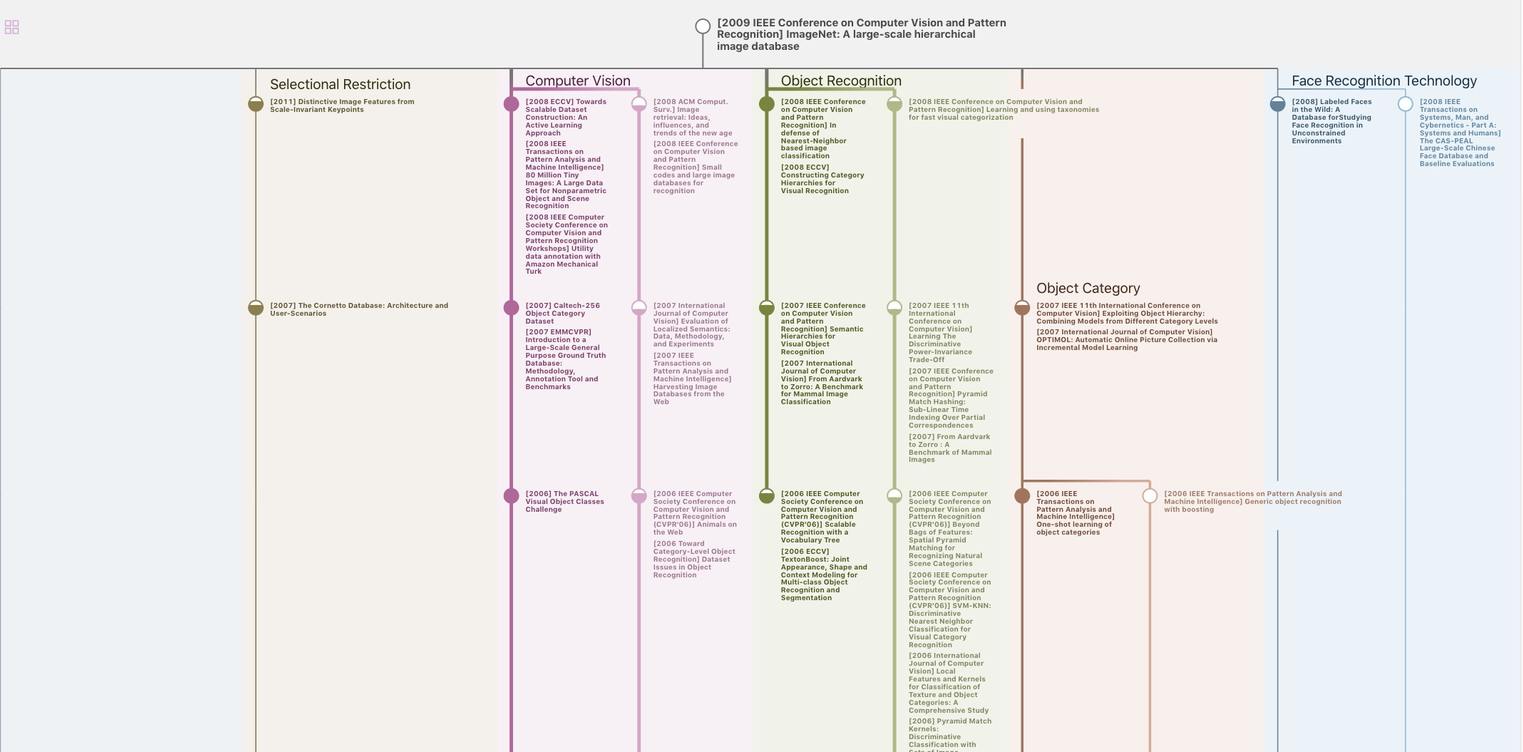
生成溯源树,研究论文发展脉络
Chat Paper
正在生成论文摘要