Divide & Conquer Imitation Learning
IEEE/RJS International Conference on Intelligent RObots and Systems (IROS)(2022)
摘要
When cast into the Deep Reinforcement Learning framework, many robotics tasks require solving a long horizon and sparse reward problem, where learning algorithms struggle. In such context, Imitation Learning (IL) can be a powerful approach to bootstrap the learning process. However, most IL methods require several expert demonstrations which can be prohibitively difficult to acquire. Only a handful of IL algorithms have shown efficiency in the context of an extreme low expert data regime where a single expert demonstration is available. In this paper, we present a novel algorithm designed to imitate complex robotic tasks from the states of an expert trajectory. Based on a sequential inductive bias, our method divides the complex task into smaller skills. The skills are learned into a goal-conditioned policy that is able to solve each skill individually and chain skills to solve the entire task. We show that our method imitates a non-holonomic navigation task and scales to a complex simulated robotic manipulation task with very high sample efficiency.
更多查看译文
关键词
learning,conquer
AI 理解论文
溯源树
样例
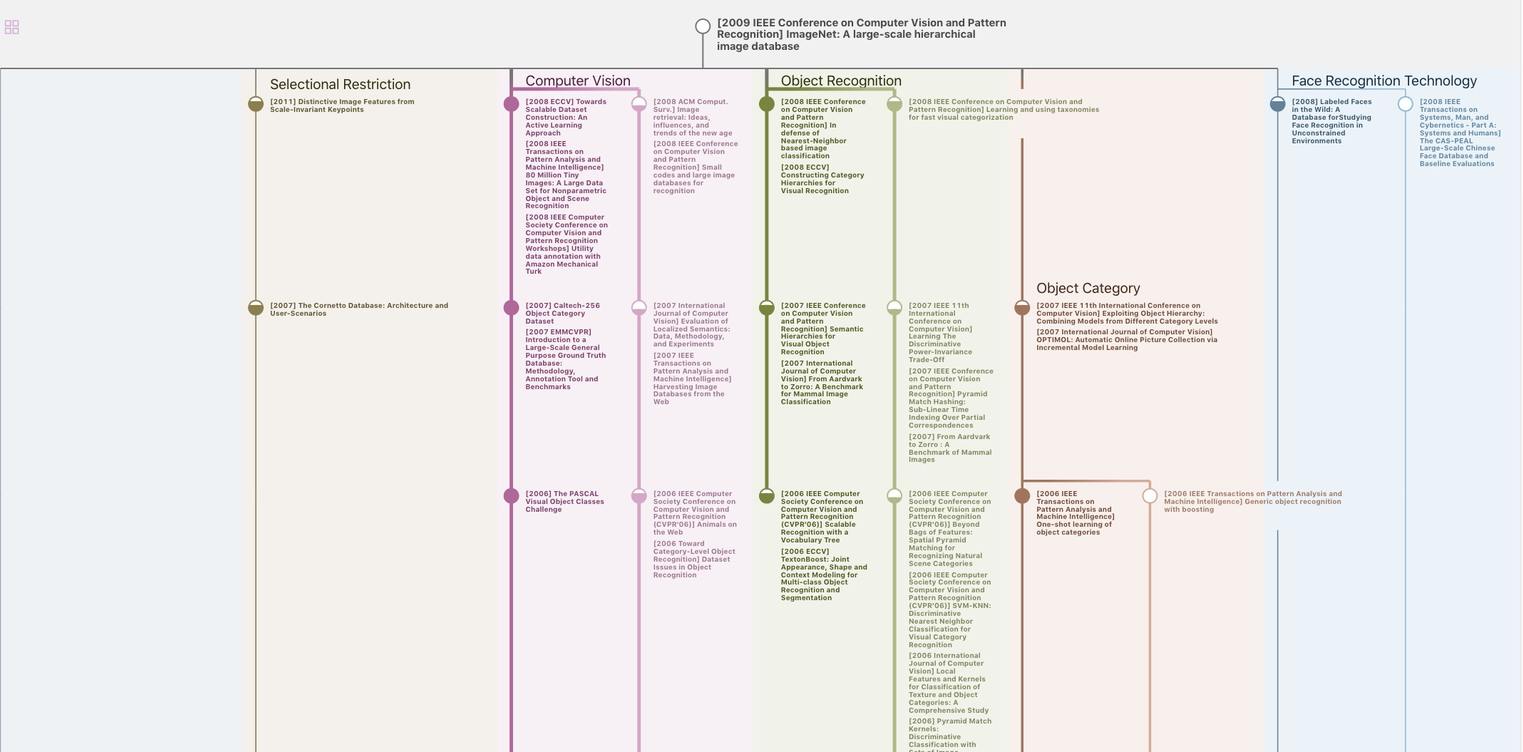
生成溯源树,研究论文发展脉络
Chat Paper
正在生成论文摘要