Masked Siamese Networks for Label-Efficient Learning.
European Conference on Computer Vision(2022)
摘要
We propose Masked Siamese Networks (MSN), a self-supervised learning framework for learning image representations. Our approach matches the representation of an image view containing randomly masked patches to the representation of the original unmasked image. This self-supervised pre-training strategy is particularly scalable when applied to Vision Transformers since only the unmasked patches are processed by the network. As a result, MSNs improve the scalability of joint-embedding architectures, while producing representations of a high semantic level that perform competitively on low-shot image classification. For instance, on ImageNet-1K, with only 5,000 annotated images, our base MSN model achieves 72.4% top-1 accuracy, and with 1% of ImageNet-1K labels, we achieve 75.7% top-1 accuracy, setting a new state-of-the-art for self-supervised learning on this benchmark. Our code is publicly available.
更多查看译文
关键词
siamese networks,learning,label-efficient
AI 理解论文
溯源树
样例
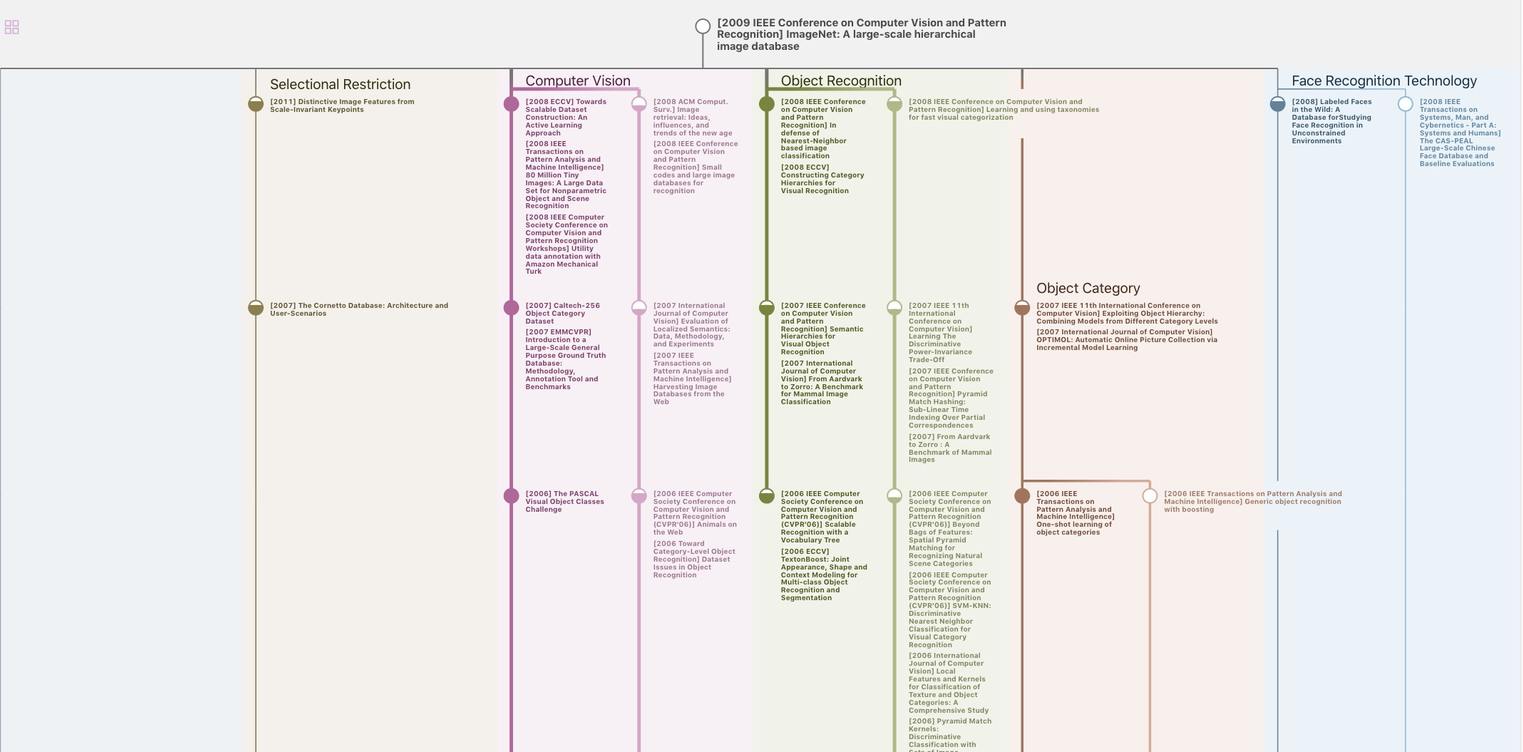
生成溯源树,研究论文发展脉络
Chat Paper
正在生成论文摘要