Label Semantic Aware Pre-training for Few-shot Text Classification
PROCEEDINGS OF THE 60TH ANNUAL MEETING OF THE ASSOCIATION FOR COMPUTATIONAL LINGUISTICS (ACL 2022), VOL 1: (LONG PAPERS)(2022)
摘要
In text classification tasks, useful information is encoded in the label names. Label semantic aware systems have leveraged this information for improved text classification performance during fine-tuning and prediction. However, use of label-semantics during pre-training has not been extensively explored. We therefore propose Label Semantic Aware Pre-training (LSAP) to improve the generalization and data efficiency of text classification systems. LSAP incorporates label semantics into pre-trained generative models (T5 in our case) by performing secondary pre-training on labeled sentences from a variety of domains. As domain-general pre-training requires large amounts of data, we develop a filtering and labeling pipeline to automatically create sentence-label pairs from unlabeled text. We perform experiments on intent (ATIS, Snips, TOPv2) and topic classification (AG News, Yahoo! Answers). LSAP obtains significant accuracy improvements over state-of-the-art models for few-shot text classification while maintaining performance comparable to state of the art in high-resource settings.
更多查看译文
关键词
classification,label,text,pre-training,few-shot
AI 理解论文
溯源树
样例
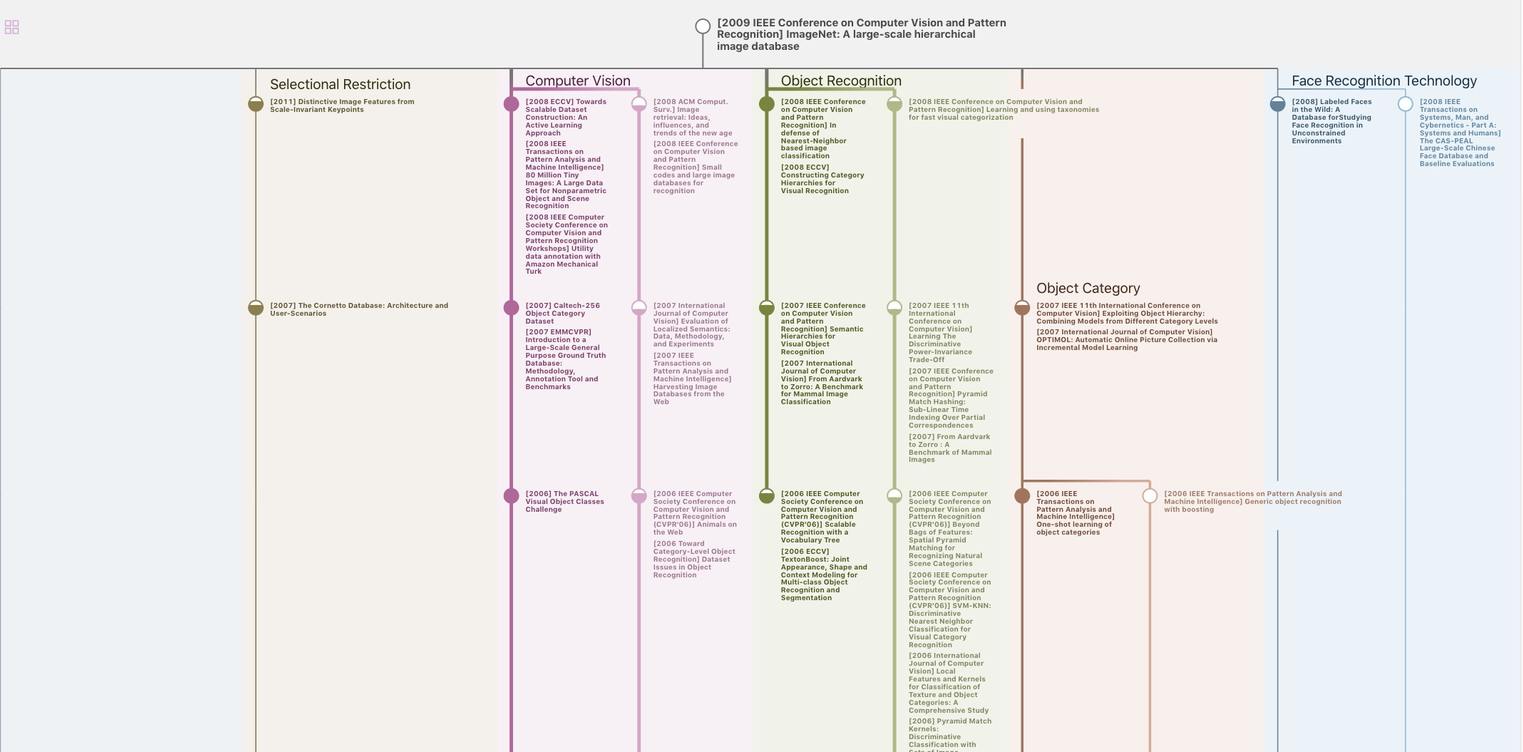
生成溯源树,研究论文发展脉络
Chat Paper
正在生成论文摘要