Graph Learning on Millions of Data in Seconds: Label Propagation Acceleration on Graph Using Data Distribution
IEEE Transactions on Pattern Analysis and Machine Intelligence(2023)
摘要
Graph-based semi-supervised learning methods have been used in a wide range of real-world applications, e.g., from social relationship mining to multimedia classification and retrieval. However, existing methods are limited along with high computational complexity or not facilitating incremental learning, which may not be powerful to deal with large-scale data, whose scale may continuously increase, in real world. This paper proposes a new method called Data Distribution Based Graph Learning (DDGL) for semi-supervised learning on large-scale data. This method can achieve a fast and effective label propagation and supports incremental learning. The key motivation is to propagate the labels along smaller-scale data distribution model parameters, rather than directly dealing with the raw data as previous methods, which accelerate the data propagation significantly. It also improves the prediction accuracy since the loss of structure information can be alleviated in this way. To enable incremental learning, we propose an adaptive graph updating strategy which can update the model when there is distribution bias between new data and the already seen data. We have conducted comprehensive experiments on multiple datasets with sample sizes increasing from seven thousand to five million. Experimental results on the classification task on large-scale data demonstrate that our proposed DDGL method improves the classification accuracy by a large margin while consuming much less time compared to state-of-the-art methods.
更多查看译文
关键词
Large-scale graph learning,data distribution,propagation acceleration
AI 理解论文
溯源树
样例
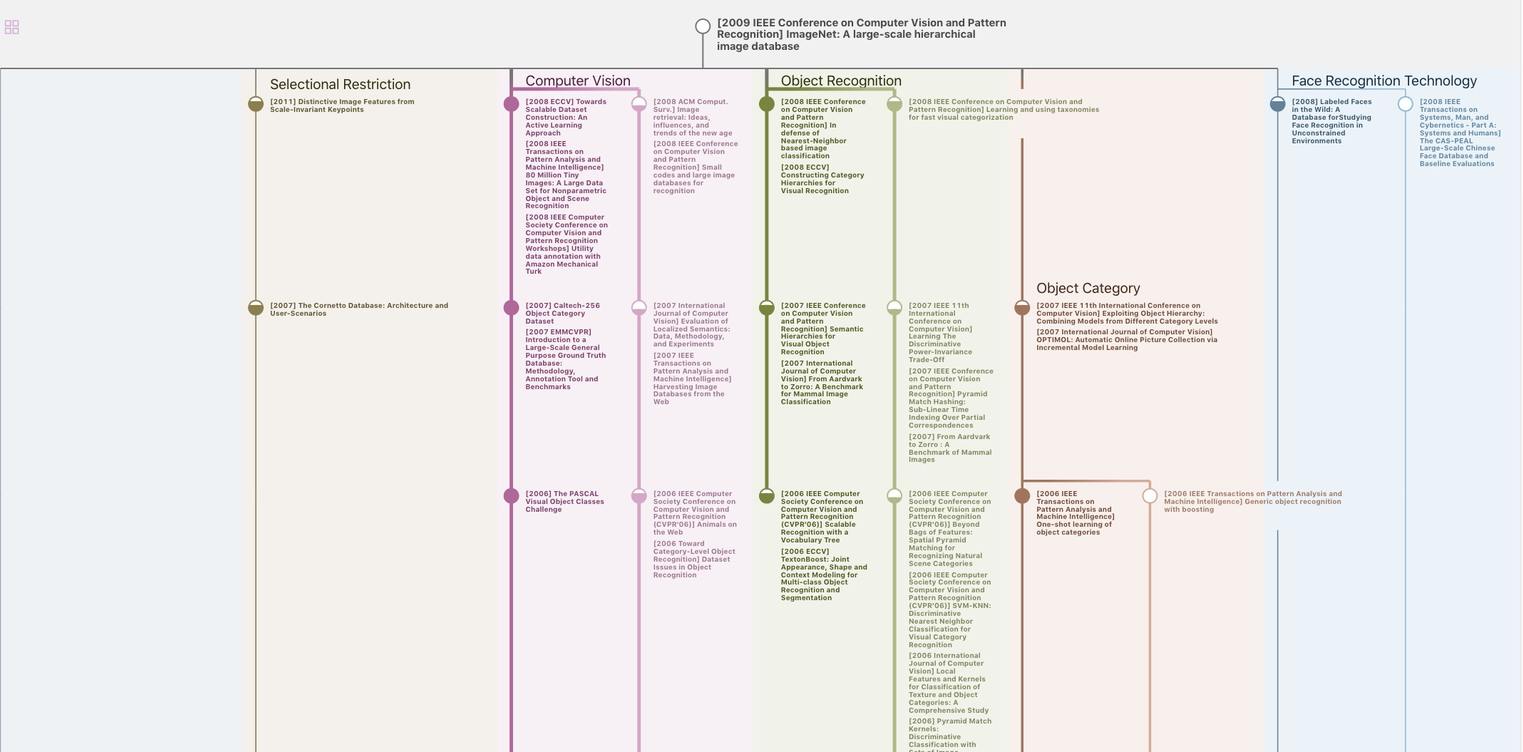
生成溯源树,研究论文发展脉络
Chat Paper
正在生成论文摘要