RISP: Rendering-Invariant State Predictor with Differentiable Simulation and Rendering for Cross-Domain Parameter Estimation
International Conference on Learning Representations (ICLR)(2022)
摘要
This work considers identifying parameters characterizing a physical system's dynamic motion directly from a video whose rendering configurations are inaccessible. Existing solutions require massive training data or lack generalizability to unknown rendering configurations. We propose a novel approach that marries domain randomization and differentiable rendering gradients to address this problem. Our core idea is to train a rendering-invariant state-prediction (RISP) network that transforms image differences into state differences independent of rendering configurations, e.g., lighting, shadows, or material reflectance. To train this predictor, we formulate a new loss on rendering variances using gradients from differentiable rendering. Moreover, we present an efficient, second-order method to compute the gradients of this loss, allowing it to be integrated seamlessly into modern deep learning frameworks. We evaluate our method in rigid-body and deformable-body simulation environments using four tasks: state estimation, system identification, imitation learning, and visuomotor control. We further demonstrate the efficacy of our approach on a real-world example: inferring the state and action sequences of a quadrotor from a video of its motion sequences. Compared with existing methods, our approach achieves significantly lower reconstruction errors and has better generalizability among unknown rendering configurations.
更多查看译文
关键词
differentiable rendering,differentiable simulation,system identification
AI 理解论文
溯源树
样例
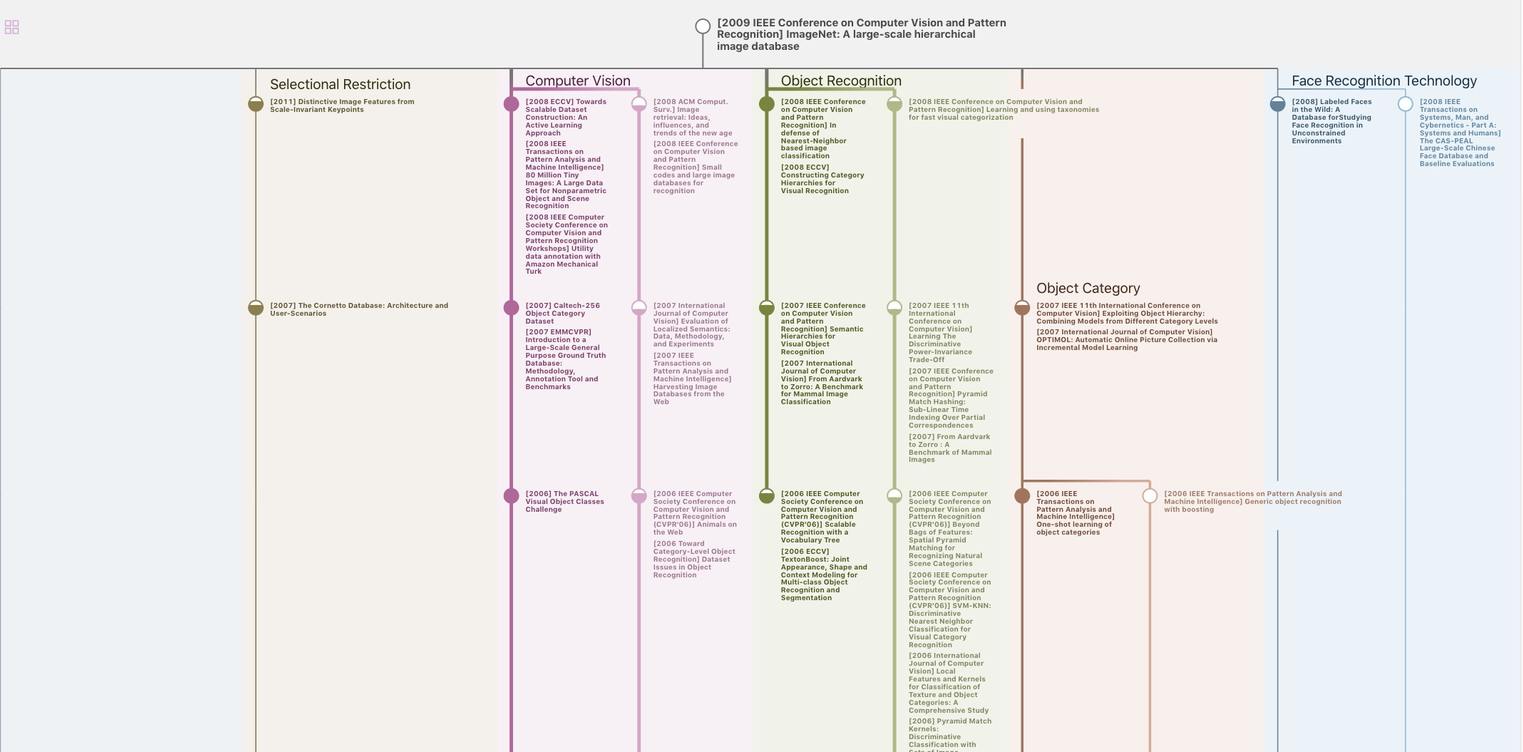
生成溯源树,研究论文发展脉络
Chat Paper
正在生成论文摘要