Stein Latent Optimization for Generative Adversarial Networks
International Conference on Learning Representations (ICLR)(2022)
摘要
Generative adversarial networks (GANs) with clustered latent spaces can perform conditional generation in a completely unsupervised manner. In the real world, the salient attributes of unlabeled data can be imbalanced. However, most of existing unsupervised conditional GANs cannot cluster attributes of these data in their latent spaces properly because they assume uniform distributions of the attributes. To address this problem, we theoretically derive Stein latent optimization that provides reparameterizable gradient estimations of the latent distribution parameters assuming a Gaussian mixture prior in a continuous latent space. Structurally, we introduce an encoder network and novel unsupervised conditional contrastive loss to ensure that data generated from a single mixture component represent a single attribute. We confirm that the proposed method, named Stein Latent Optimization for GANs (SLOGAN), successfully learns balanced or imbalanced attributes and achieves state-of-the-art unsupervised conditional generation performance even in the absence of attribute information (e.g., the imbalance ratio). Moreover, we demonstrate that the attributes to be learned can be manipulated using a small amount of probe data.
更多查看译文
关键词
Generative Adversarial Networks,Unsupervised Conditional GANs
AI 理解论文
溯源树
样例
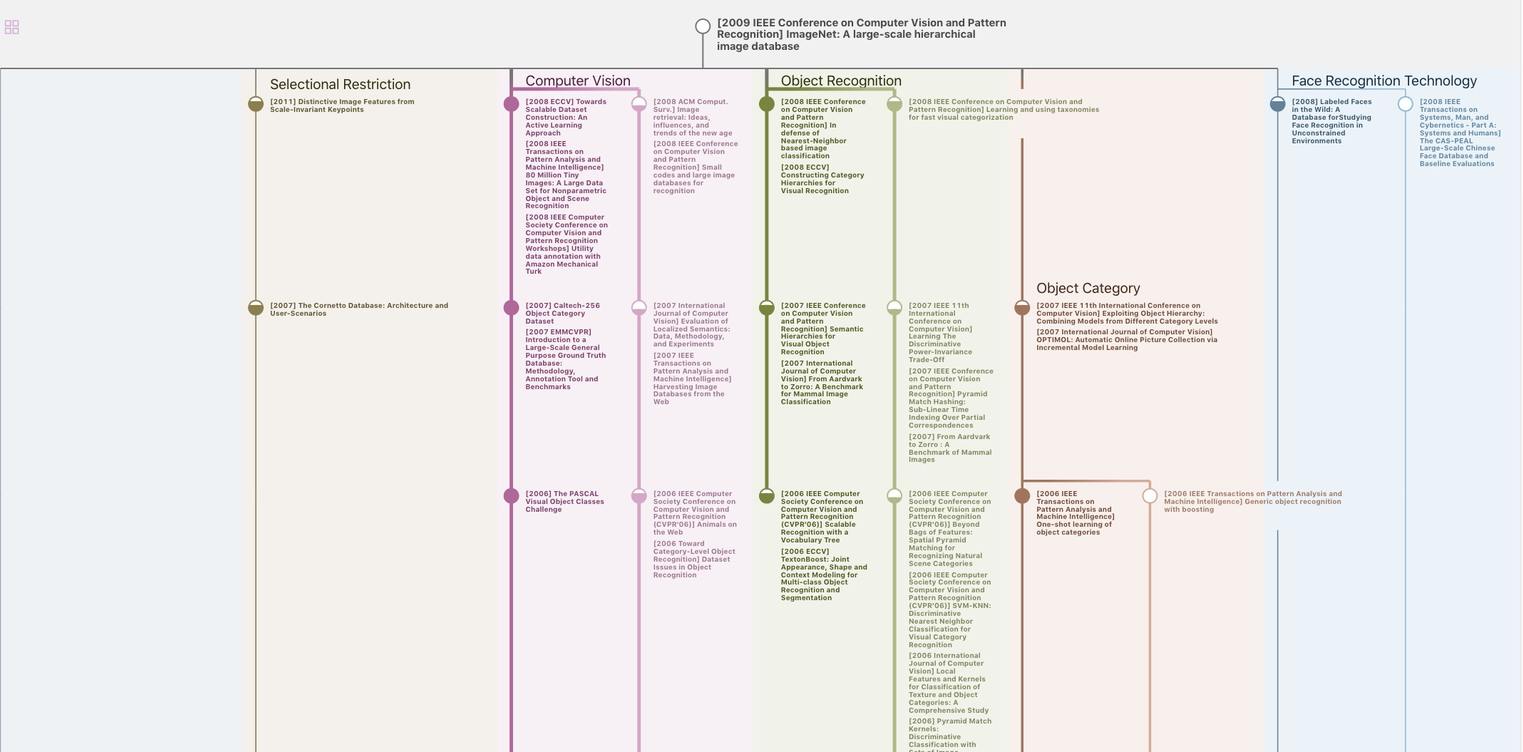
生成溯源树,研究论文发展脉络
Chat Paper
正在生成论文摘要