DictFormer: Tiny Transformer with Shared Dictionary
International Conference on Learning Representations (ICLR)(2022)
摘要
We introduce DictFormer with the efficient shared dictionary to provide a compact, fast, and accurate transformer model. DictFormer significantly reduces the redundancy in the transformer's parameters by replacing the prior transformer's parameters with a compact, shared dictionary, few unshared coefficients, and indices. Also, DictFormer enables faster computations since expensive weights multiplications are converted into cheap shared look-ups on dictionary and few linear projections. Training dictionary and coefficients are not trivial since indices used for looking up dictionary are not differentiable. We adopt a sparse-constraint training with $l_1\,\,norm$ relaxation to learn coefficients and indices in DictFormer. DictFormer is flexible to support different model sizes by dynamically changing dictionary size. Compared to existing lightweight Transformers, DictFormer consistently reduces model size over Transformer on multiple tasks, e.g., machine translation, abstractive summarization, and language modeling. Extensive experiments show that DictFormer reduces $3.6\times$ to $8.9\times$ model size with similar accuracy over multiple tasks, compared to Transformer.
更多查看译文
关键词
Transformer,Parameters Sharing,Tiny,On-device Transformer,Machine Translation,Attention,Dictionary Sharing
AI 理解论文
溯源树
样例
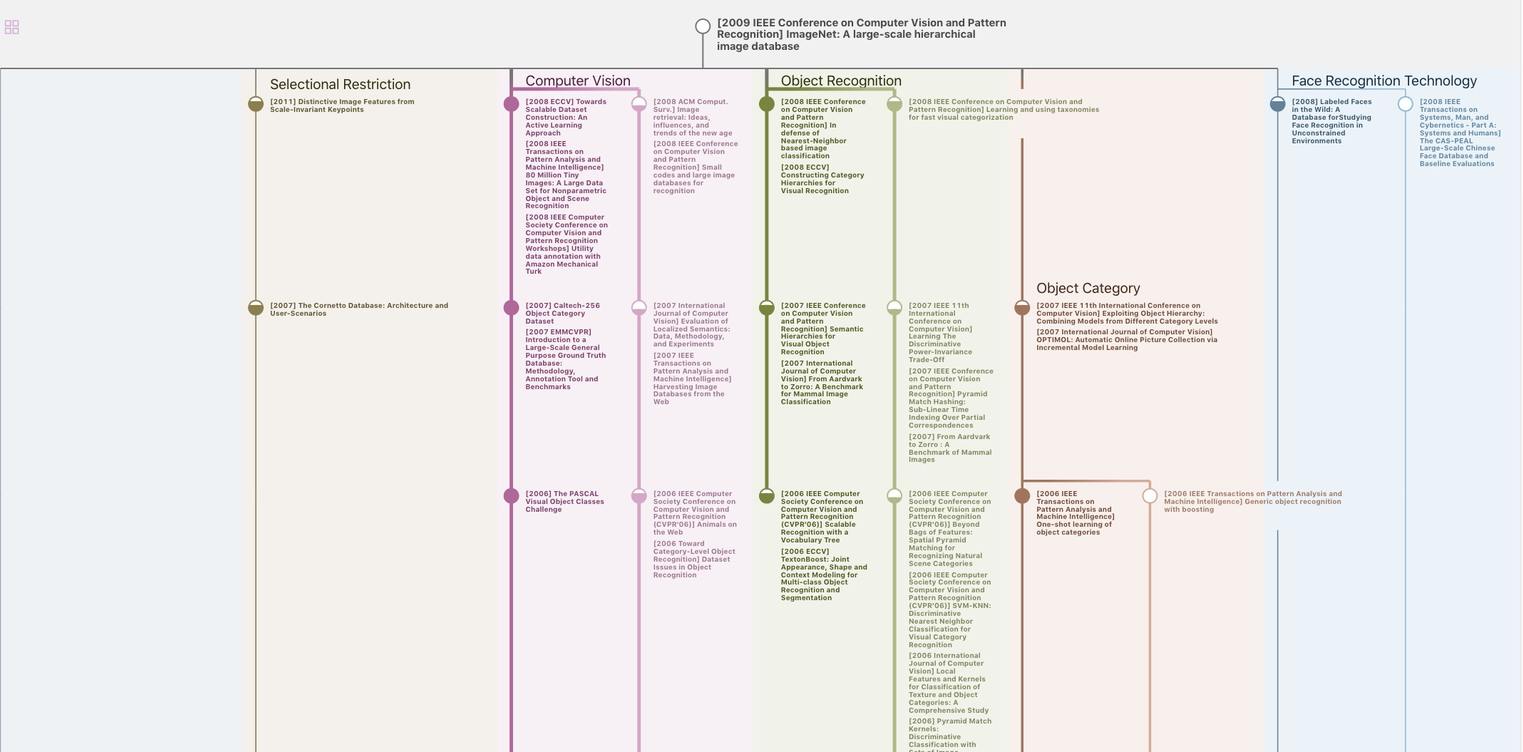
生成溯源树,研究论文发展脉络
Chat Paper
正在生成论文摘要