Automated Atomic Silicon Quantum Dot Circuit Design via Deep Reinforcement Learning
arxiv(2022)
摘要
Robust automated design tools are crucial for the proliferation of any computing technology. We introduce the first automated design tool for the silicon dangling bond quantum dot computing technology, which is an extremely versatile and flexible single-atom computing circuitry framework. The automated designer is capable of navigating the complex, hyperdimensional design spaces of arbitrarily sized design areas and truth tables by employing a tabula rasa double-deep Q-learning reinforcement learning algorithm. Robust policy convergence is demonstrated for a wide range of two-input, one-output logic circuits and a two-input, two-output half-adder, designed with an order of magnitude fewer SiDBs in several orders of magnitude less time than the only other half-adder demonstrated in the literature. We anticipate that reinforcement learning-based automated design tools will accelerate the development of the SiDB quantum dot computing technology, leading to its eventual adoption in specialized computing hardware.
更多查看译文
关键词
reinforcement learning,quantum,silicon
AI 理解论文
溯源树
样例
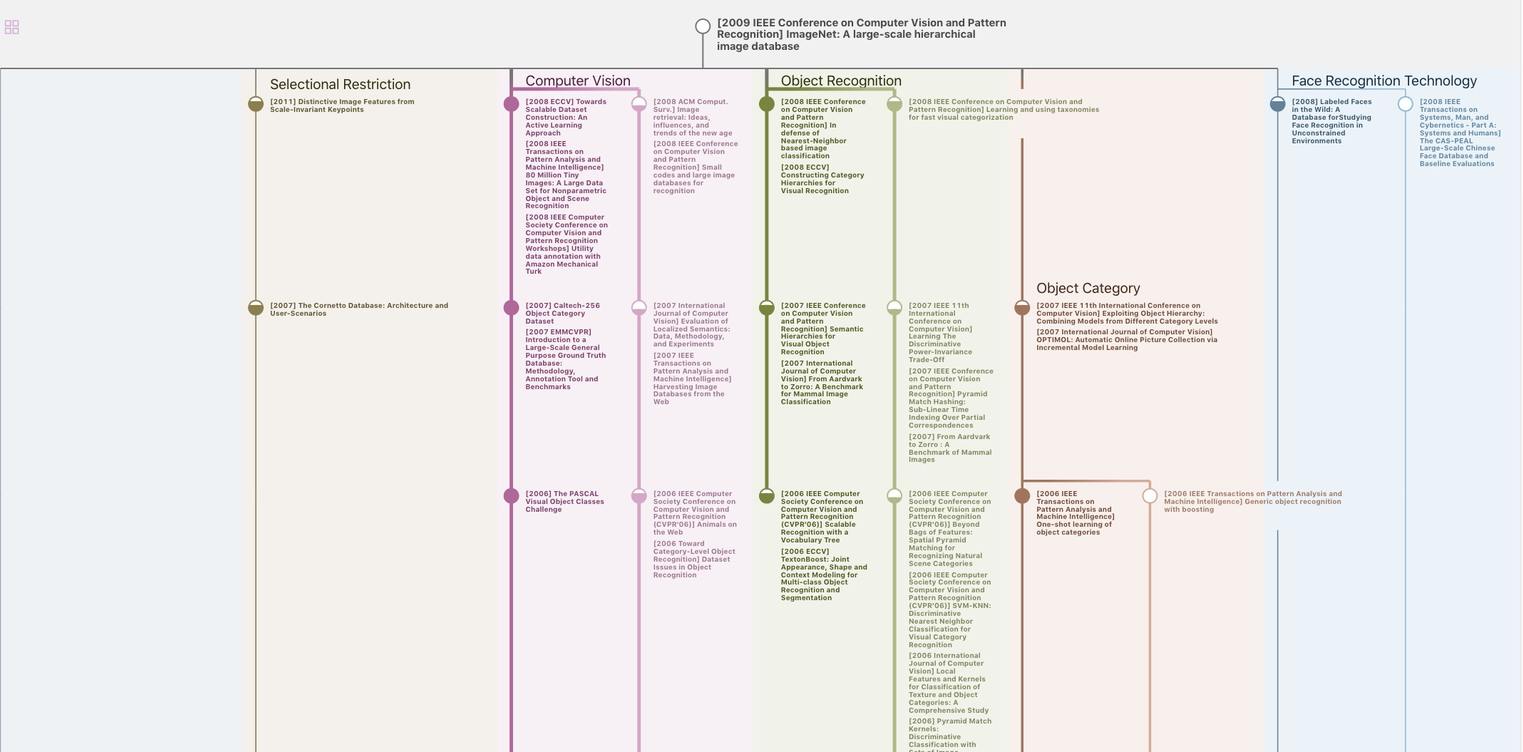
生成溯源树,研究论文发展脉络
Chat Paper
正在生成论文摘要