From CNNs to Vision Transformers -- A Comprehensive Evaluation of Deep Learning Models for Histopathology
arxiv(2022)
摘要
While machine learning is currently transforming the field of histopathology, the domain lacks a comprehensive evaluation of state-of-the-art models based on essential but complementary quality requirements beyond a mere classification accuracy. In order to fill this gap, we conducted an extensive evaluation by benchmarking a wide range of classification models, including recent vision transformers, convolutional neural networks and hybrid models comprising transformer and convolutional models. We thoroughly tested the models on five widely used histopathology datasets containing whole slide images of breast, gastric, and colorectal cancer and developed a novel approach using an image-to-image translation model to assess the robustness of a cancer classification model against stain variations. Further, we extended existing interpretability methods to previously unstudied models and systematically reveal insights of the models' classification strategies that allow for plausibility checks and systematic comparisons. The study resulted in specific model recommendations for practitioners as well as putting forward a general methodology to quantify a model's quality according to complementary requirements that can be transferred to future model architectures.
更多查看译文
关键词
histopathology,cnns,deep
AI 理解论文
溯源树
样例
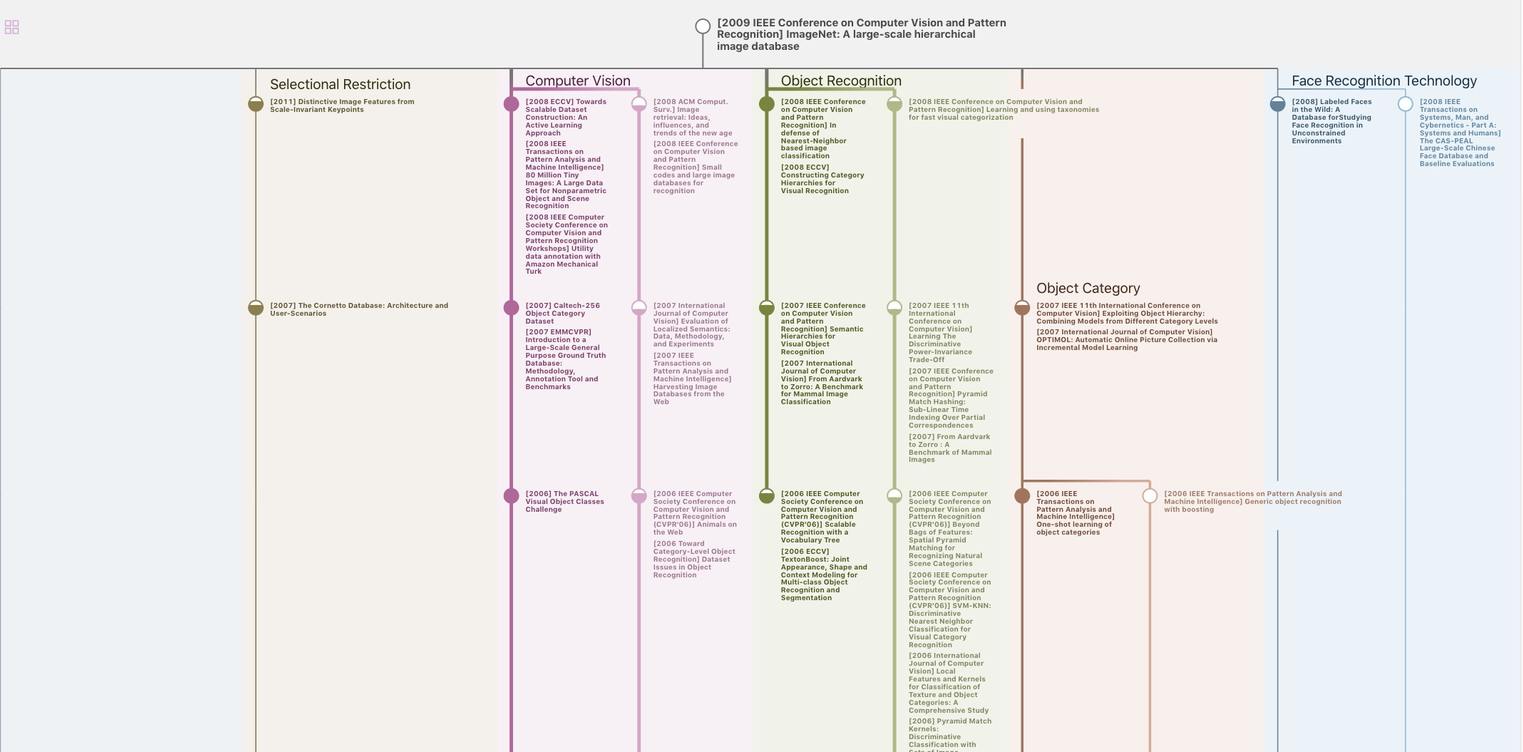
生成溯源树,研究论文发展脉络
Chat Paper
正在生成论文摘要