FederatedScope: A Flexible Federated Learning Platform for Heterogeneity
arxiv(2022)
摘要
Although remarkable progress has been made by existing federated learning (FL) platforms to provide infrastructures for development, these platforms may not well tackle the challenges brought by various types of heterogeneity, including the heterogeneity in participants' local data, resources, behaviors and learning goals. To fill this gap, in this paper, we propose a novel FL platform, named FederatedScope, which employs an event-driven architecture to provide users with great flexibility to independently describe the behaviors of different participants. Such a design makes it easy for users to describe participants with various local training processes, learning goals and backends, and coordinate them into an FL course with synchronous or asynchronous training strategies. Towards an easy-to-use and flexible platform, FederatedScope enables rich types of plug-in operations and components for efficient further development, and we have implemented several important components to better help users with privacy protection, attack simulation and auto-tuning. We have released FederatedScope at https://github.com/alibaba/FederatedScope to promote academic research and industrial deployment of federated learning in a wide range of scenarios.
更多查看译文
AI 理解论文
溯源树
样例
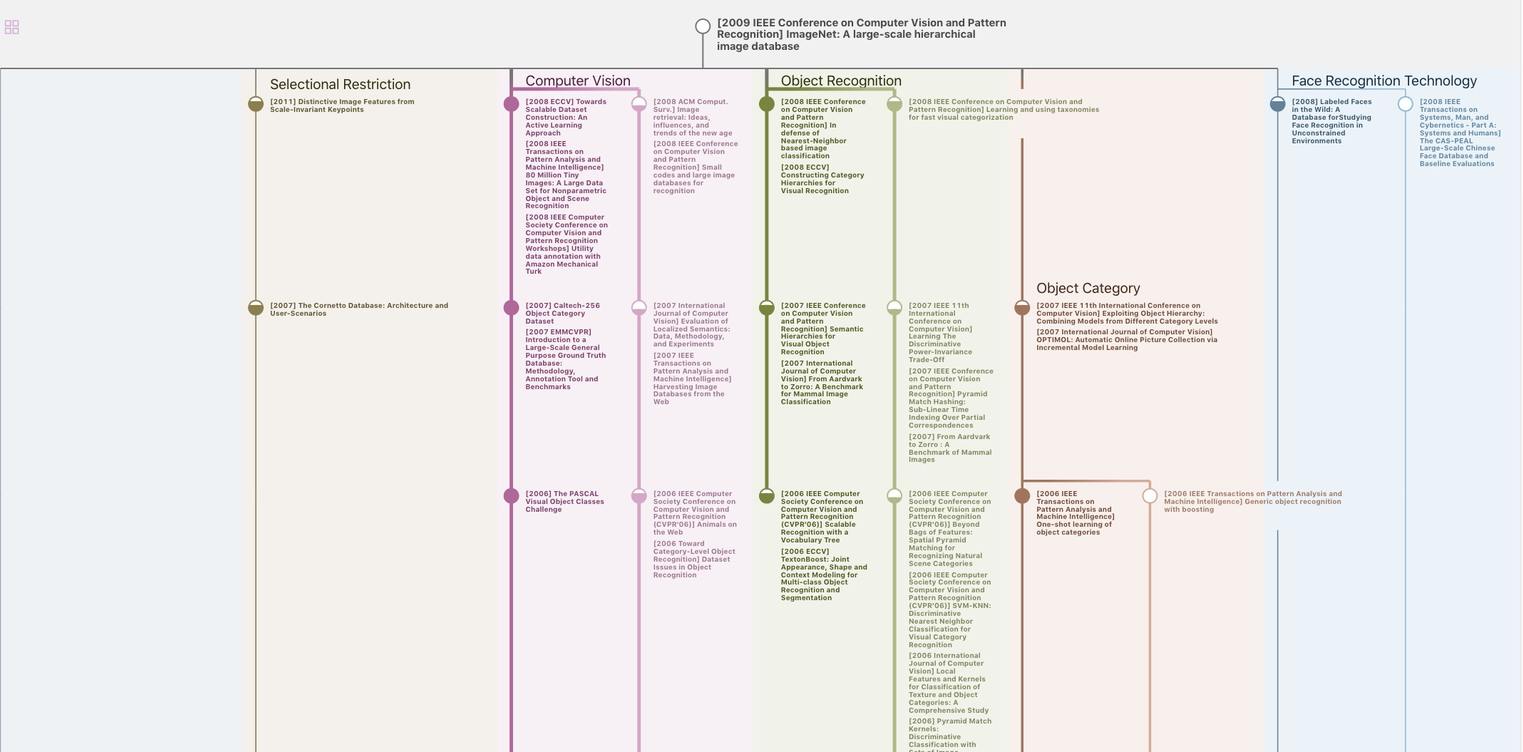
生成溯源树,研究论文发展脉络
Chat Paper
正在生成论文摘要