Multi-Label Clinical Time-Series Generation via Conditional GAN
IEEE TRANSACTIONS ON KNOWLEDGE AND DATA ENGINEERING(2024)
摘要
In recent years, deep learning has been successfully adopted in a wide range of applications related to electronic health records (EHRs) such as representation learning and clinical event prediction. However, due to privacy constraints, limited access to EHR becomes a bottleneck for deep learning research. To mitigate these concerns, generative adversarial networks (GANs) have been successfully used for generating EHR data. However, there are still challenges in high-quality EHR generation, including generating time-series EHR data and imbalanced uncommon diseases. In this work, we propose a Multi-label Time-series GAN (MTGAN) to generate EHR and simultaneously improve the quality of uncommon disease generation. The generator of MTGAN uses a gated recurrent unit (GRU) with a smooth conditional matrix to generate sequences and uncommon diseases. The critic gives scores using Wasserstein distance to recognize real samples from synthetic samples by considering both data and temporal features. We also propose a training strategy to calculate temporal features for real data and stabilize GAN training. Furthermore, we design multiple statistical metrics and prediction tasks to evaluate the generated data. Experimental results demonstrate the quality of the synthetic data and the effectiveness of MTGAN in generating realistic sequential EHR data, especially for uncommon diseases.
更多查看译文
关键词
Diseases,Generative adversarial networks,Generators,Training,Task analysis,Synthetic data,Measurement,Electronic health records,generative adversarial network (GAN),time-series generation,imbalanced data
AI 理解论文
溯源树
样例
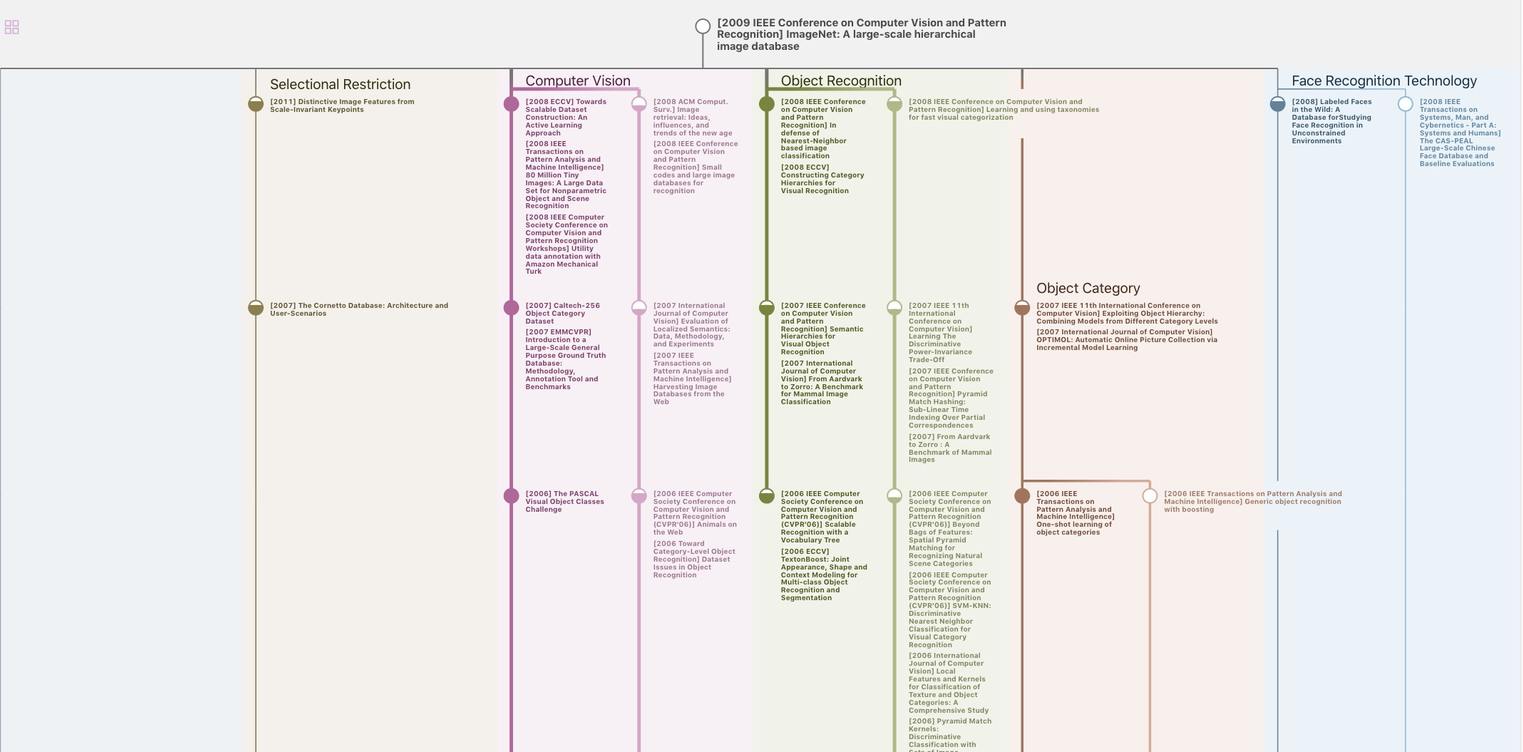
生成溯源树,研究论文发展脉络
Chat Paper
正在生成论文摘要