Towards reliable and explainable AI model for pulmonary nodule diagnosis
BIOMEDICAL SIGNAL PROCESSING AND CONTROL(2024)
摘要
Background and Objective: Lung cancer has the highest mortality rate of all deadly cancers in the world. Early detection is essential to the treatment of lung cancer. However, detection and accurate diagnosis of pulmonary nodules depend heavily on the experience of radiologists and can be a heavy workload for them. Computer-aided diagnosis (CAD) systems have been developed to assist radiologists in nodule detection and diagnosis, greatly easing the workload while increasing diagnosis accuracy. Recent developments in deep learning have greatly improved the performance of CAD systems. However, the lack of model reliability and interpretability remains a major obstacle for its large-scale clinical application.Methods: We proposed a multi-task explainable deep-learning model for pulmonary nodule diagnosis, named "ExPN-Net". The proposed ExPN-Net can not only predict lesion malignancy but also identify nodule characteristics. Further, the location of each characteristic can also be visualized for visual interpretability. We first used 3D nnU-Net to obtain the probability maps of lung nodules, which were used as attention for the following classification task. Then, we used an anatomical attention gate (AAG) mechanism to introduce the spatial attention information into the classification network. Finally, the soft activation map (SAM) module is used to generate fine-grained activation maps to indicate the nodule characteristics. To evaluate the performance, we validated ExPN-Net on both public and in-house datasets. 624 and 807 participants were enrolled from the public LIDC and our-inhouse datasets, respectively. The roles of different modules in ExPN-Net are analyzed by ablation experiments.Results: ExPN-Net achieved a test AUC of 0.992 and 0.923 on the LIDC public and in-house datasets, respectively. Moreover, experimental results proved that by incorporating characteristic identification tasks into the multitask model, the accuracy of the malignancy classification can also be improved.Conclusions: This work successfully updates the state-of-the-art diagnosis accuracy of pulmonary nodules by incorporating characteristics and anatomical attention mechanism. Furthermore, our multi-task explainable model provides a solution for AI models to better interact with radiologists in a clinical environment. Source code is available at: https://github.com/KumoLiu/explainablePN.
更多查看译文
关键词
Deep learning,CAD,Interpretability,Pulmonary Nodule,Nodule characteristics
AI 理解论文
溯源树
样例
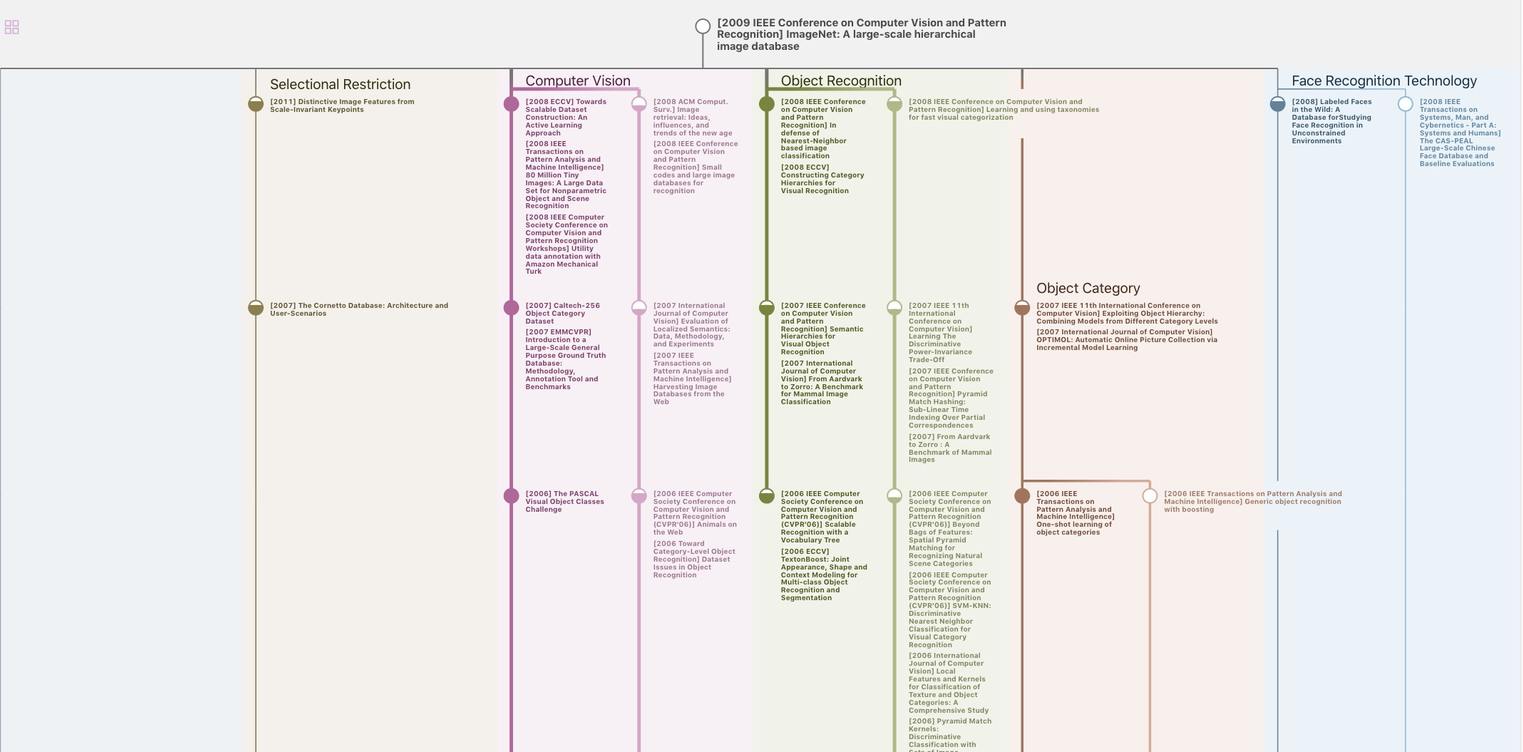
生成溯源树,研究论文发展脉络
Chat Paper
正在生成论文摘要