Functional feature selection reveals the inner cell mass in human pre-implantation embryo single cell RNA sequencing data
biorxiv(2022)
摘要
A major challenge with single cell gene expression data is to discern meaningful cellular heterogeneity from technical or biological noise. To address this challenge, we present Entropy Sorting, a mathematical framework that distinguishes genes indicative of cell identity. On synthetic data we demonstrate the removal of noisy signals to reveal a higher resolution of gene expression patterns than commonly used feature selection methods. We then apply Entropy Sorting to human pre-implantation embryo scRNA-seq data. Previous studies failed to identify a distinct signature of the inner cell mass (ICM), suggesting that lineage segregation in the human embryo does not follow the paradigm established in mouse. However, Entropy Sorting identifies a set of highly informative genes that clearly separate the ICM, Trophectoderm, Epiblast and Hypoblast and reveals sequential lineage bifurcations as in mouse. Entropy sorting thus provides a powerful approach for maximising information extraction from high dimensional datasets such as scRNA-seq data.
### Competing Interest Statement
The authors have declared no competing interest.
更多查看译文
AI 理解论文
溯源树
样例
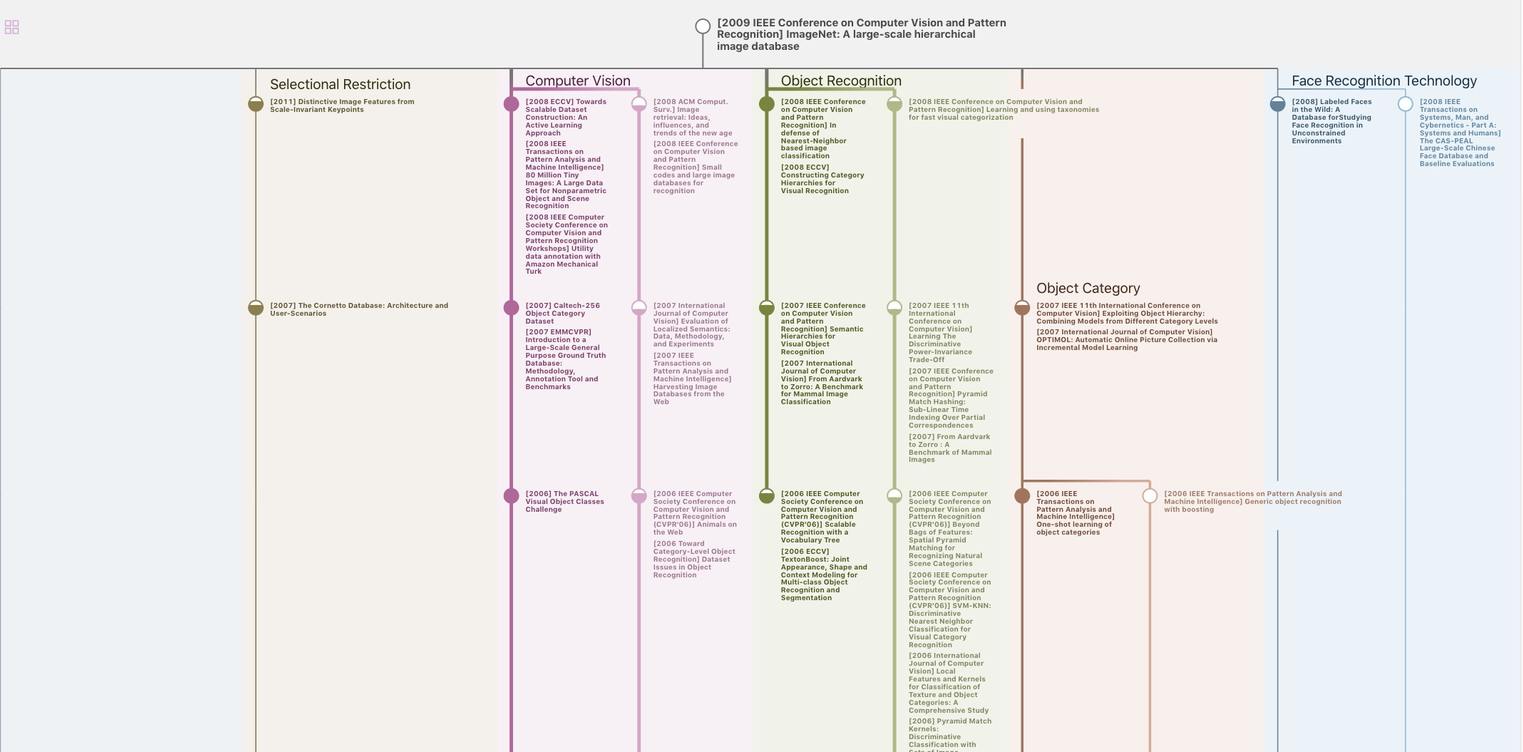
生成溯源树,研究论文发展脉络
Chat Paper
正在生成论文摘要