Mechanisms of human dynamic object recognition revealed by sequential deep neural networks
biorxiv(2023)
摘要
Author summaryOur visual world is both stable and dynamic: even within a single glance, a scene may change dramatically. Brains thus need to balance integration of information over time to create stable percepts with sensitivity to changes in sensory input, e.g., to rapidly recognize new objects. How do brains and, in particular, visual systems achieve this? Here, we addressed this question by having humans and different neural network models perform the same object recognition task in which sequences of images were shown in rapid or slow succession. We observed that models treating images as a continuous sequence by integrating its processing over time reproduced human performance patterns better than models processing every single image at a time. Furthermore, models equipped with sensory adaptation, a form of stimulus habituation, better recognized objects in faster sequences and more efficiently captured human behaviour. These findings show that lateral recurrence and adaptation jointly enable object recognition across a wide variety of time scales, suggesting a critical role for these mechanisms in dynamic vision. Humans can quickly recognize objects in a dynamically changing world. This ability is showcased by the fact that observers succeed at recognizing objects in rapidly changing image sequences, at up to 13 ms/image. To date, the mechanisms that govern dynamic object recognition remain poorly understood. Here, we developed deep learning models for dynamic recognition and compared different computational mechanisms, contrasting feedforward and recurrent, single-image and sequential processing as well as different forms of adaptation. We found that only models that integrate images sequentially via lateral recurrence mirrored human performance (N = 36) and were predictive of trial-by-trial responses across image durations (13-80 ms/image). Importantly, models with sequential lateral-recurrent integration also captured how human performance changes as a function of image presentation durations, with models processing images for a few time steps capturing human object recognition at shorter presentation durations and models processing images for more time steps capturing human object recognition at longer presentation durations. Furthermore, augmenting such a recurrent model with adaptation markedly improved dynamic recognition performance and accelerated its representational dynamics, thereby predicting human trial-by-trial responses using fewer processing resources. Together, these findings provide new insights into the mechanisms rendering object recognition so fast and effective in a dynamic visual world.
更多查看译文
关键词
human dynamic object recognition,sequential deep neural networks,neural networks
AI 理解论文
溯源树
样例
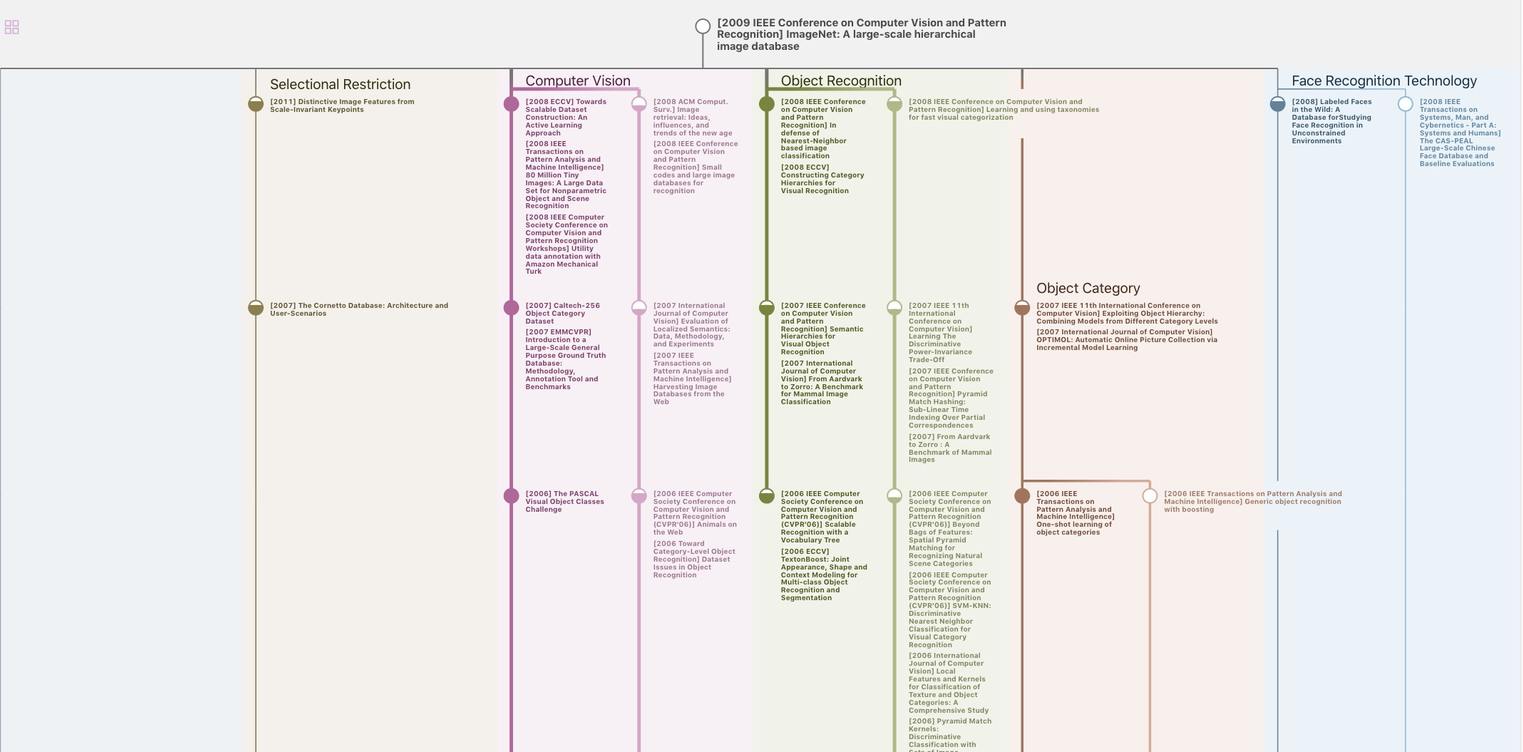
生成溯源树,研究论文发展脉络
Chat Paper
正在生成论文摘要