SwapMix: Diagnosing and Regularizing the Over-Reliance on Visual Context in Visual Question Answering
IEEE Conference on Computer Vision and Pattern Recognition(2022)
摘要
While Visual Question Answering (VQA) has progressed rapidly, previous works raise concerns about robustness of current VQA models. In this work, we study the robustness of VQA models from a novel perspective: visual context. We suggest that the models over-rely on the visual context, i.e., irrelevant objects in the image, to make predictions. To diagnose the models' reliance on visual context and measure their robustness, we propose a simple yet effective perturbation technique, SwapMix. SwapMix perturbs the visual context by swapping features of irrelevant context objects with features from other objects in the dataset. Using SwapMix we are able to change answers to more than 45% of the questions for a representative VQA model. Additionally, we train the models with perfect sight and find that the context over-reliance highly depends on the quality of visual representations. In addition to diagnosing, SwapMix can also be applied as a data augmentation strategy during training in order to regularize the context over-reliance. By swapping the context object features, the model reliance on context can be suppressed effectively. Two representative VQA models are studied using SwapMix: a co-attention model MCAN and a large-scale pretrained model LXMERT. Our experiments on the popular GQA dataset show the effectiveness of SwapMix for both diagnosing model robustness, and regularizing the over-reliance on visual context. The code for our method is available at https://github.com/vipulgupta1011/swapmix
更多查看译文
关键词
Vision + language, Visual reasoning
AI 理解论文
溯源树
样例
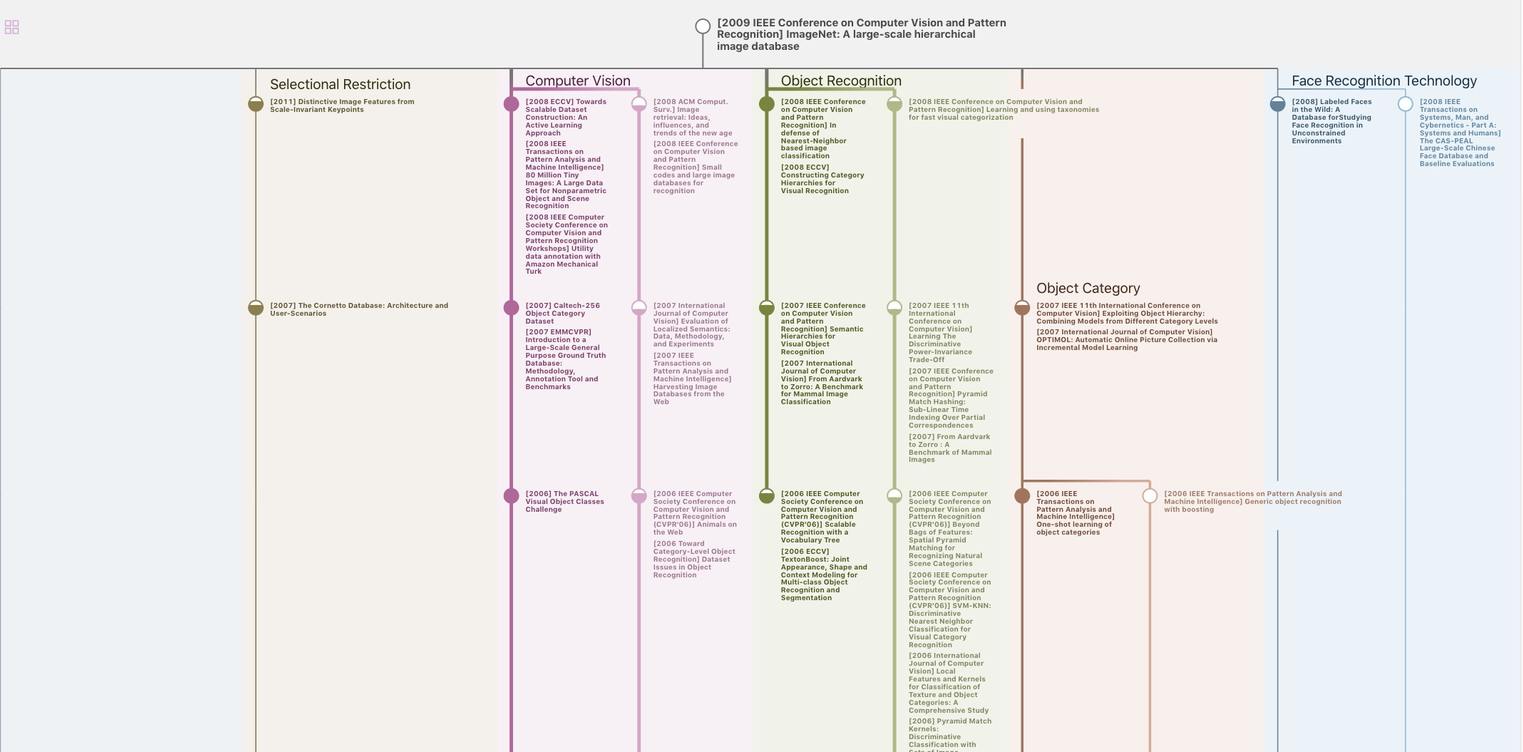
生成溯源树,研究论文发展脉络
Chat Paper
正在生成论文摘要