Model-free Learning for Risk-constrained Linear Quadratic Regulator with Structured Feedback in Networked Systems
arxiv(2022)
摘要
We develop a model-free learning algorithm for the infinite-horizon linear quadratic regulator (LQR) problem. Specifically, (risk) constraints and structured feedback are considered, in order to reduce the state deviation while allowing for a sparse communication graph in practice. By reformulating the dual problem as a nonconvex-concave minimax problem, we adopt the gradient descent max-oracle (GDmax), and for model-free setting, the stochastic (S)GDmax using zero-order policy gradient. By bounding the Lipschitz and smoothness constants of the LQR cost using specifically defined sublevel sets, we can design the stepsize and related parameters to establish convergence to a stationary point (at with high probability). Numerical tests in a networked microgrid control problem have validated the convergence of our proposed SGDmax algorithm while demonstrating the effectiveness of risk constraints. The SGDmax algorithm has attained a satisfactory optimality gap compared to the classical LQR control, especially for the full feedback case.
更多查看译文
关键词
linear quadratic regulator,structured feedback,networked systems,learning,model-free,risk-constrained
AI 理解论文
溯源树
样例
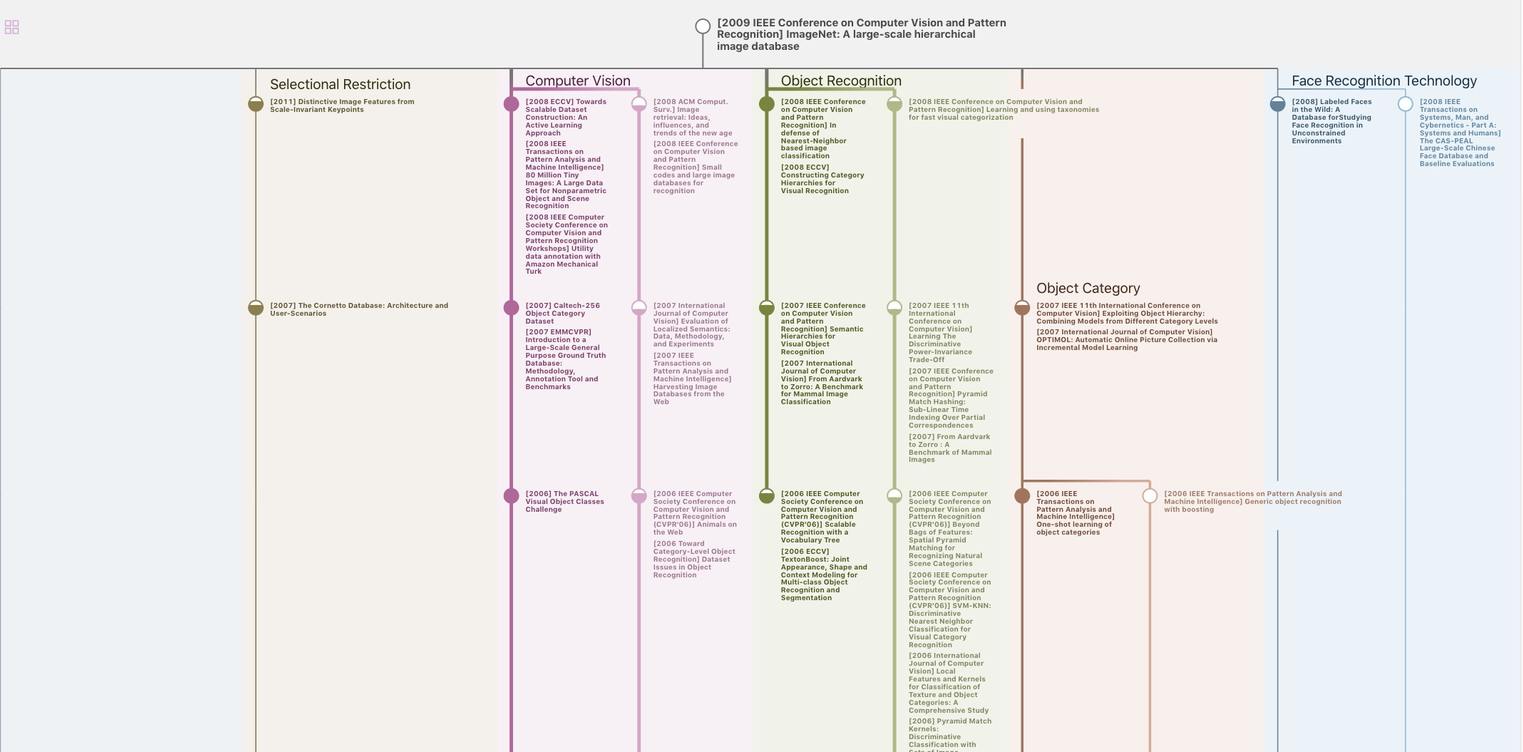
生成溯源树,研究论文发展脉络
Chat Paper
正在生成论文摘要