How Stable Are Transferability Metrics Evaluations?
European Conference on Computer Vision(2022)
摘要
Transferability metrics is a maturing field with increasing interest, which aims at providing heuristics for selecting the most suitable source models to transfer to a given target dataset, without fine-tuning them all. However, existing works rely on custom experimental setups which differ across papers, leading to inconsistent conclusions about which transferability metrics work best. In this paper we conduct a large-scale study by systematically constructing a broad range of 715k experimental setup variations. We discover that even small variations to an experimental setup lead to different conclusions about the superiority of a transferability metric over another. Then we propose better evaluations by aggregating across many experiments, enabling to reach more stable conclusions. As a result, we reveal the superiority of LogME at selecting good source datasets to transfer from in a semantic segmentation scenario, NLEEP at selecting good source architectures in an image classification scenario, and GBC at determining which target task benefits most from a given source model. Yet, no single transferability metric works best in all scenarios.
更多查看译文
关键词
transferability metrics evaluations
AI 理解论文
溯源树
样例
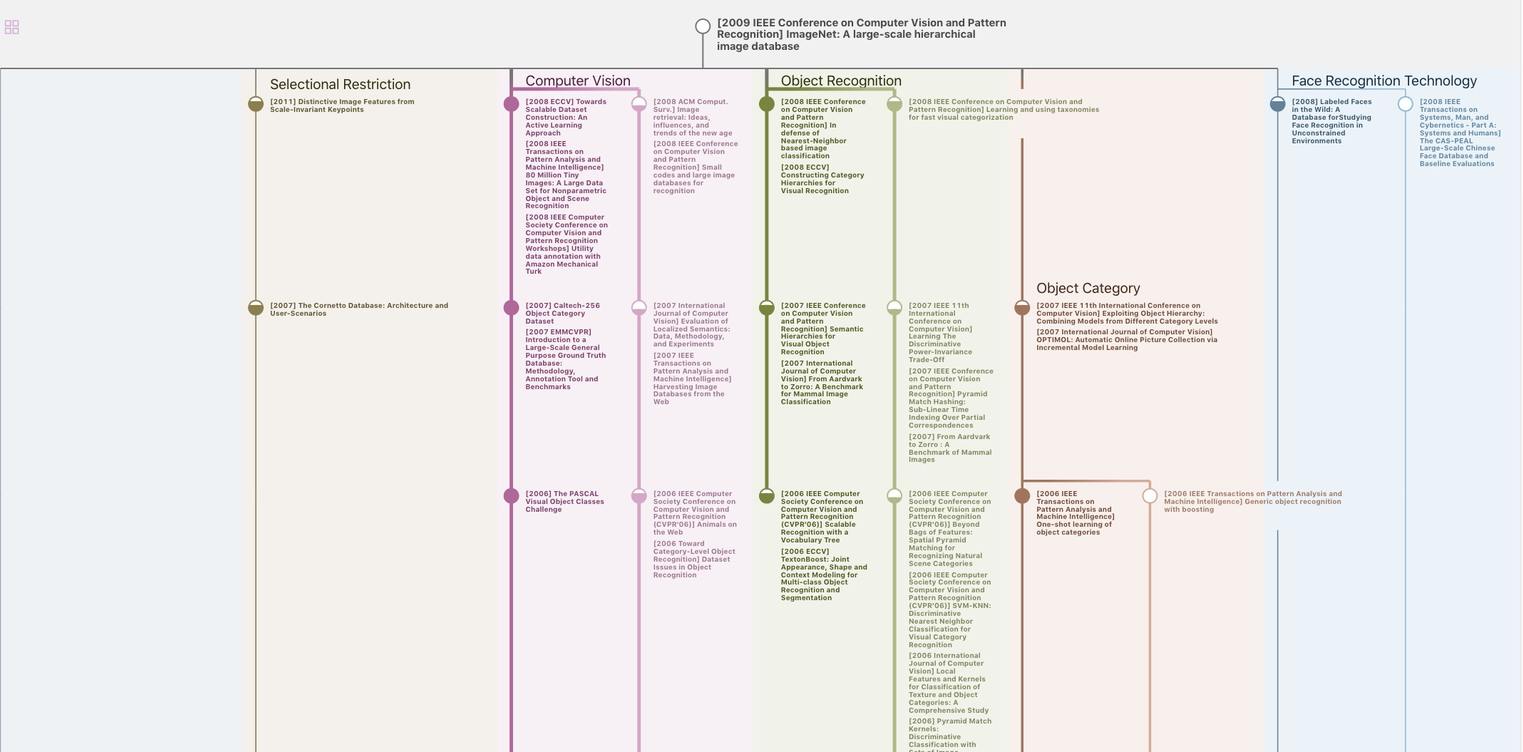
生成溯源树,研究论文发展脉络
Chat Paper
正在生成论文摘要