ES6D: A Computation Efficient and Symmetry-Aware 6D Pose Regression Framework.
IEEE Conference on Computer Vision and Pattern Recognition(2022)
摘要
In this paper, a computation efficient regression framework is presented for estimating the 6D pose of rigid objects from a single RGB-D image, which is applicable to handling symmetric objects. This framework is designed in a simple architecture that efficiently extracts point-wise features from RGB-D data using a fully convolutional network, called XYZNet, and directly regresses the 6D pose without any post refinement. In the case of symmetric object, one object has multiple ground-truth poses, and this one-to-many relationship may lead to estimation ambiguity. In order to solve this ambiguity problem, we design a symmetry-invariant pose distance metric, called average (maximum) grouped primitives distance or A(M)GPD. The proposed A(M)GPD loss can make the regression network converge to the correct state, i.e., all minima in the A(M)GPD loss surface are mapped to the correct poses. Extensive experiments on YCB-Video and T-LESS datasets demonstrate the proposed framework's substantially superior performance in top accuracy and low computational cost.
更多查看译文
关键词
computation efficient,symmetry-aware
AI 理解论文
溯源树
样例
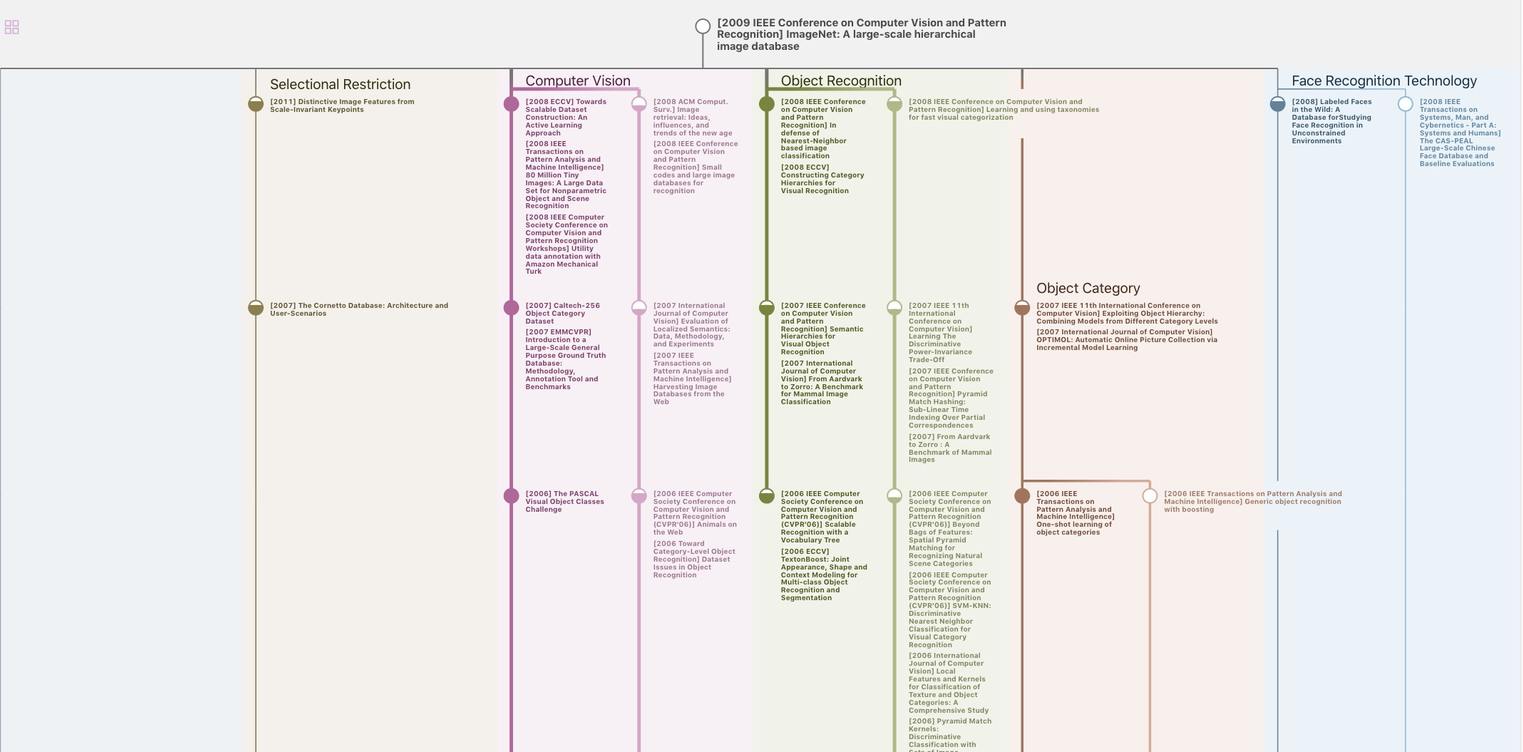
生成溯源树,研究论文发展脉络
Chat Paper
正在生成论文摘要