AutoProtoNet: Interpretability for Prototypical Networks
arxiv(2022)
摘要
In meta-learning approaches, it is difficult for a practitioner to make sense of what kind of representations the model employs. Without this ability, it can be difficult to both understand what the model knows as well as to make meaningful corrections. To address these challenges, we introduce AutoProtoNet, which builds interpretability into Prototypical Networks by training an embedding space suitable for reconstructing inputs, while remaining convenient for few-shot learning. We demonstrate how points in this embedding space can be visualized and used to understand class representations. We also devise a prototype refinement method, which allows a human to debug inadequate classification parameters. We use this debugging technique on a custom classification task and find that it leads to accuracy improvements on a validation set consisting of in-the-wild images. We advocate for interpretability in meta-learning approaches and show that there are interactive ways for a human to enhance meta-learning algorithms.
更多查看译文
关键词
prototypical networks,interpretability
AI 理解论文
溯源树
样例
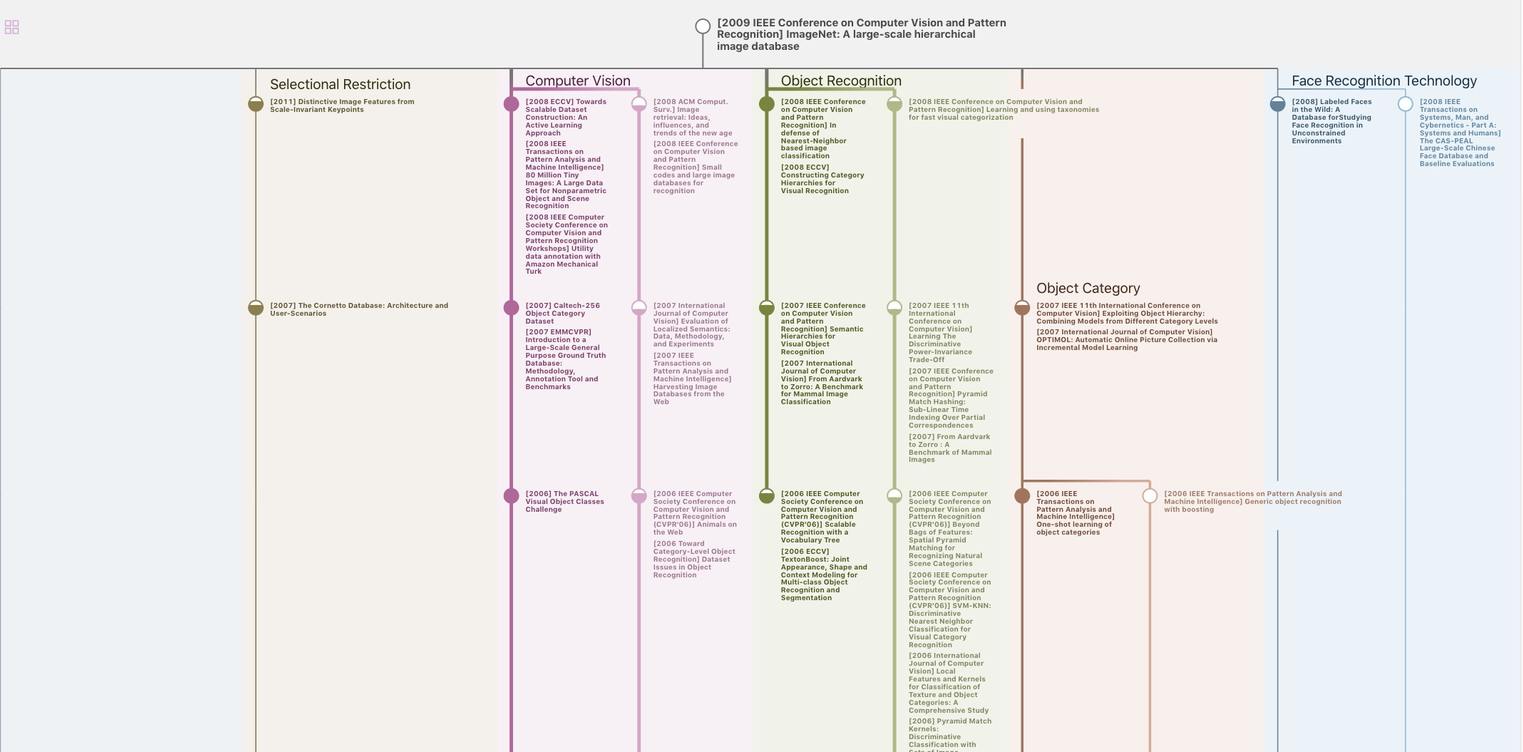
生成溯源树,研究论文发展脉络
Chat Paper
正在生成论文摘要