FaiRIR: Mitigating Exposure Bias from Related Item Recommendations in Two-Sided Platforms
arxiv(2022)
摘要
Related Item Recommendations (RIRs) are ubiquitous in most online platforms today, including e-commerce and content streaming sites. These recommendations not only help users compare items related to a given item, but also play a major role in bringing traffic to individual items, thus deciding the exposure that different items receive. With a growing number of people depending on such platforms to earn their livelihood, it is important to understand whether different items are receiving their desired exposure. To this end, our experiments on multiple real-world RIR datasets reveal that the existing RIR algorithms often result in very skewed exposure distribution of items, and the quality of items is not a plausible explanation for such skew in exposure. To mitigate this exposure bias, we introduce multiple flexible interventions (FaiRIR) in the RIR pipeline. We instantiate these mechanisms with two well-known algorithms for constructing related item recommendations -- rating-SVD and item2vec -- and show on real-world data that our mechanisms allow for a fine-grained control on the exposure distribution, often at a small or no cost in terms of recommendation quality, measured in terms of relatedness and user satisfaction.
更多查看译文
关键词
Exposure bias,fair related item recommendation (FaiRIR),related item recommendation (RIR),two-sided platforms
AI 理解论文
溯源树
样例
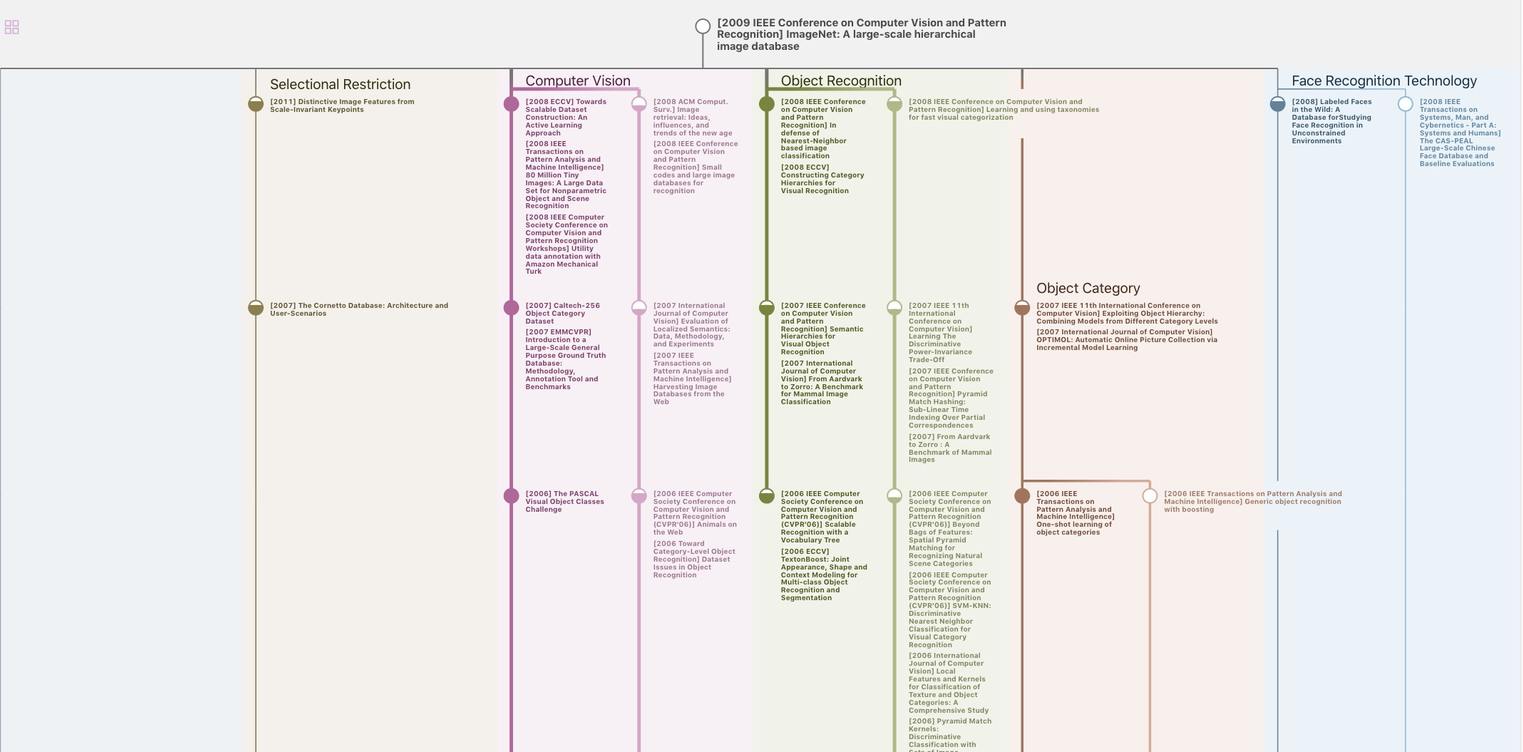
生成溯源树,研究论文发展脉络
Chat Paper
正在生成论文摘要