Development and validation of multivariable machine learning algorithms to predict risk of cancer in symptomatic patients referred urgently from primary care: a diagnostic accuracy study
BMJ OPEN(2022)
摘要
Objectives To develop and validate tests to assess the risk of any cancer for patients referred to the NHS Urgent Suspected Cancer (2-week wait, 2WW) clinical pathways. Setting Primary and secondary care, one participating regional centre. Participants Retrospective analysis of data from 371 799 consecutive 2WW referrals in the Leeds region from 2011 to 2019. The development cohort was composed of 224 669 consecutive patients with an urgent suspected cancer referral in Leeds between January 2011 and December 2016. The diagnostic algorithms developed were then externally validated on a similar consecutive sample of 147 130 patients (between January 2017 and December 2019). All such patients over the age of 18 with a minimum set of blood counts and biochemistry measurements available were included in the cohort. Primary and secondary outcome measures sensitivity, specificity, negative predictive value, positive predictive value, Receiver Operating Characteristic (ROC) curve Area Under Curve (AUC), calibration curves Results We present results for two clinical use-cases. In use-case 1, the algorithms identify 20% of patients who do not have cancer and may not need an urgent 2WW referral. In use-case 2, they identify 90% of cancer cases with a high probability of cancer that could be prioritised for review. Conclusions Combining a panel of widely available blood markers produces effective blood tests for cancer for NHS 2WW patients. The tests are affordable, and can be deployed rapidly to any NHS pathology laboratory with no additional hardware requirements.
更多查看译文
关键词
health informatics, oncology, statistics & research methods
AI 理解论文
溯源树
样例
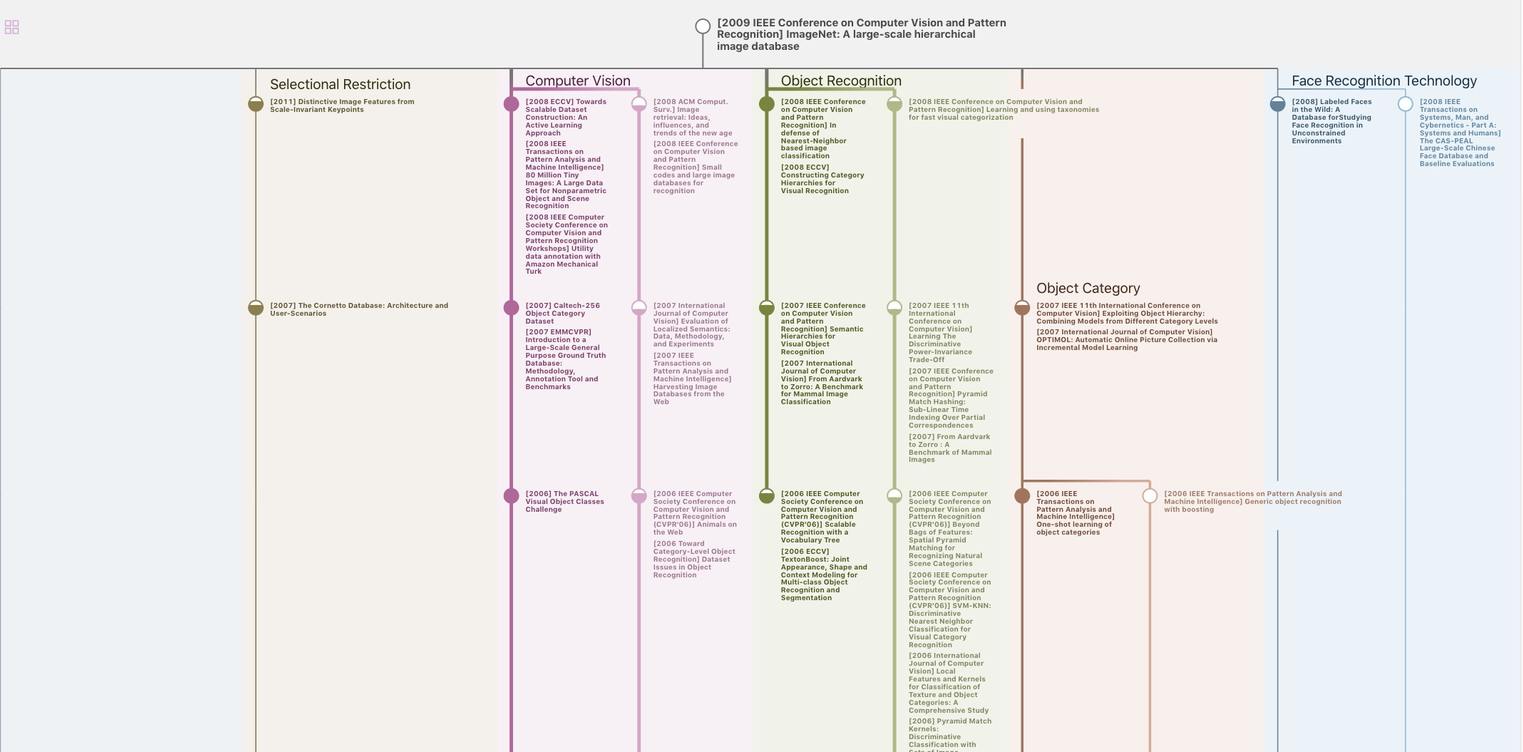
生成溯源树,研究论文发展脉络
Chat Paper
正在生成论文摘要