Deep neural models for color classification and color constancy.
Journal of vision(2022)
摘要
Color constancy is our ability to perceive constant colors across varying illuminations. Here, we trained deep neural networks to be color constant and evaluated their performance with varying cues. Inputs to the networks consisted of two-dimensional images of simulated cone excitations derived from three-dimensional (3D) rendered scenes of 2,115 different 3D shapes, with spectral reflectances of 1,600 different Munsell chips, illuminated under 278 different natural illuminations. The models were trained to classify the reflectance of the objects. Testing was done with four new illuminations with equally spaced CIEL*a*b* chromaticities, two along the daylight locus and two orthogonal to it. High levels of color constancy were achieved with different deep neural networks, and constancy was higher along the daylight locus. When gradually removing cues from the scene, constancy decreased. Both ResNets and classical ConvNets of varying degrees of complexity performed well. However, DeepCC, our simplest sequential convolutional network, represented colors along the three color dimensions of human color vision, while ResNets showed a more complex representation.
更多查看译文
AI 理解论文
溯源树
样例
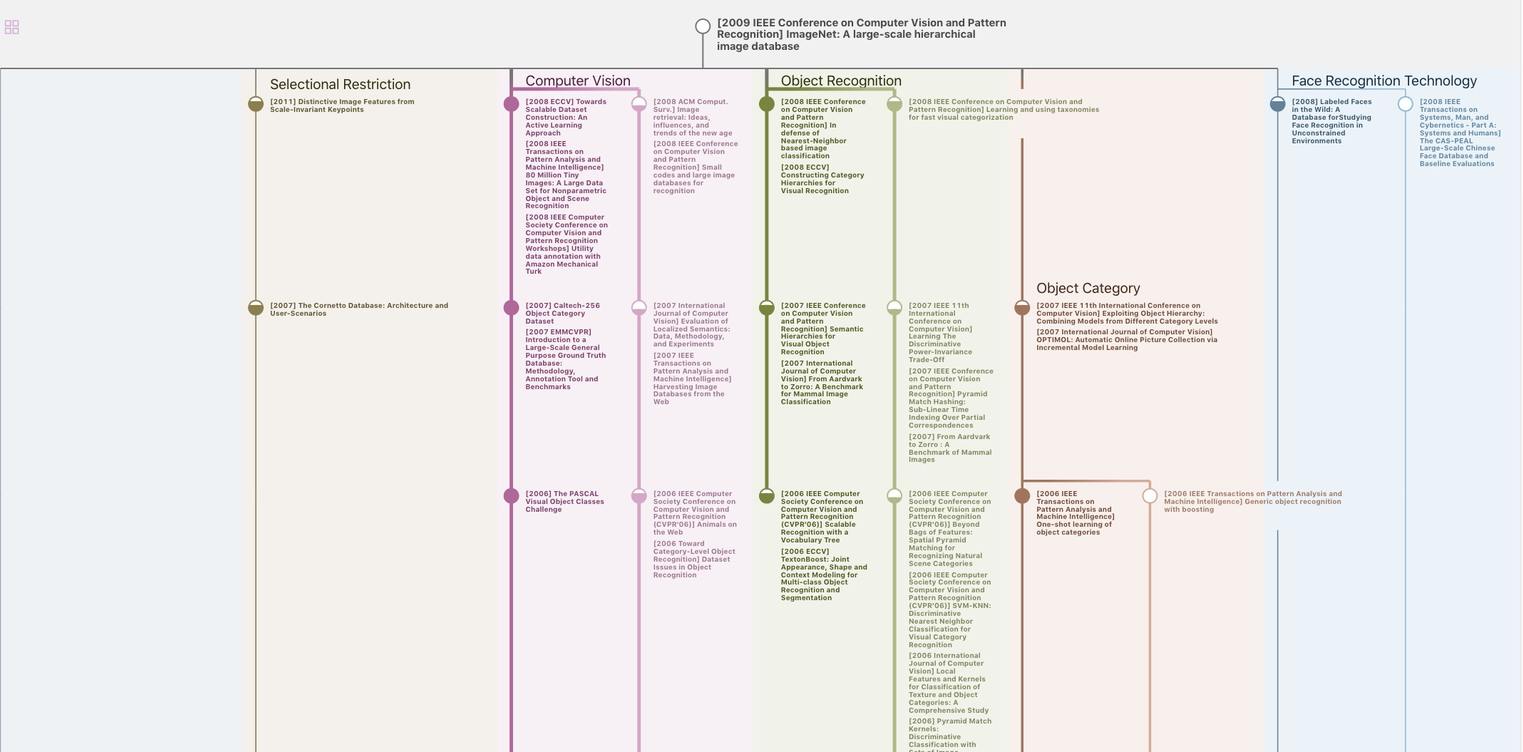
生成溯源树,研究论文发展脉络
Chat Paper
正在生成论文摘要