Clozer: Adaptable Data Augmentation for Cloze-style Reading Comprehension
PROCEEDINGS OF THE 7TH WORKSHOP ON REPRESENTATION LEARNING FOR NLP(2022)
Abstract
Task-adaptive pre-training (TAPT) alleviates the lack of labelled data and provides performance lift by adapting unlabelled data to downstream task. Unfortunately, existing adaptations mainly involve deterministic rules that cannot generalize well. Here, we propose Clozer, a sequence-tagging based doze answer extraction method used in TAPT that is extendable for adaptation on any doze-style machine reading comprehension (MRC) downstream tasks. We experiment on multiple-choice dozestyle MRC tasks, and show that Clozer performs significantly better compared to the oracle and state-of-the-art in escalating TAPT effectiveness in lifting model performance, and prove that Clozer is able to recognize the gold answers independently of any heuristics.
MoreTranslated text
AI Read Science
Must-Reading Tree
Example
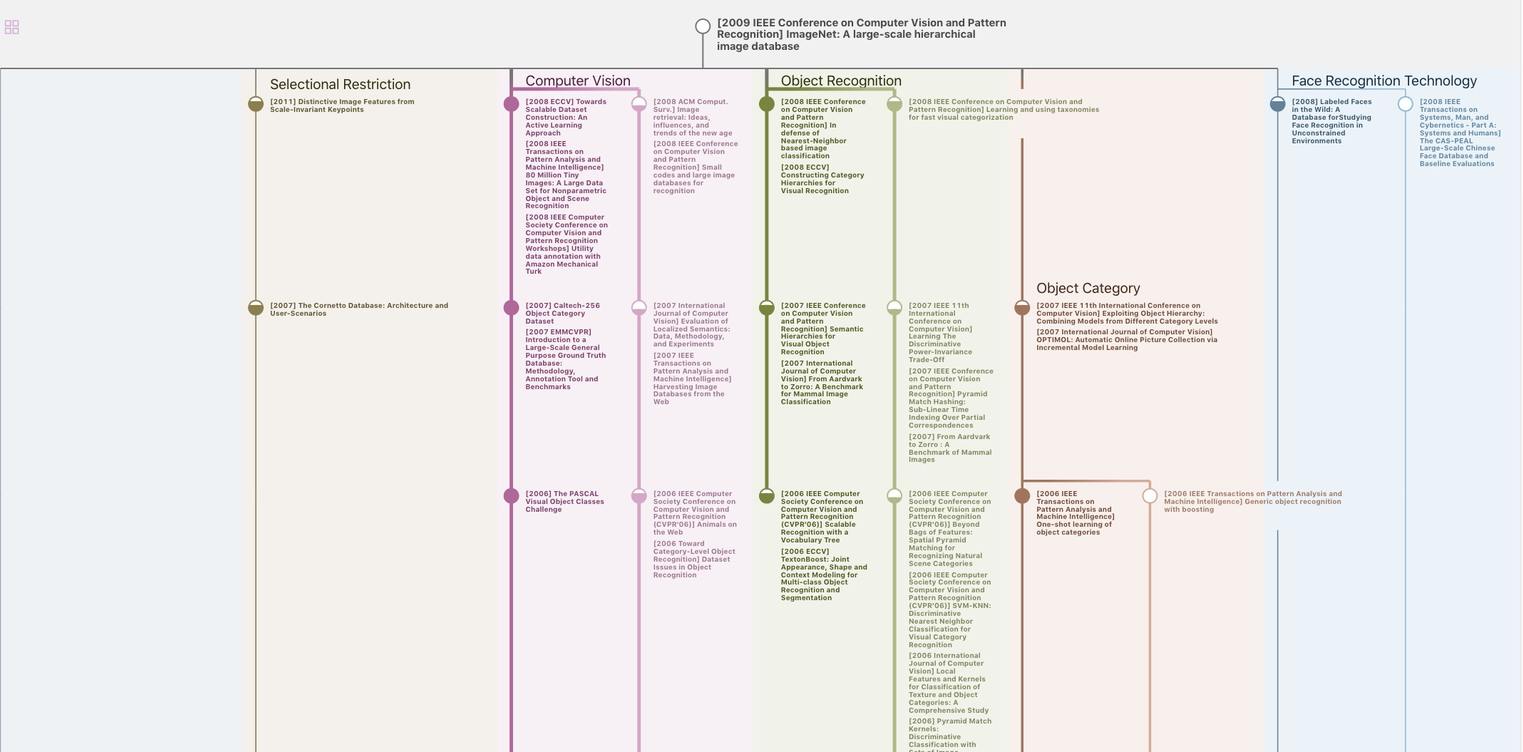
Generate MRT to find the research sequence of this paper
Chat Paper
Summary is being generated by the instructions you defined