FedVLN: Privacy-Preserving Federated Vision-and-Language Navigation.
European Conference on Computer Vision(2022)
摘要
Data privacy is a central problem for embodied agents that can perceive the environment, communicate with humans, and act in the real world. While helping humans complete tasks, the agent may observe and process sensitive information of users, such as house environments, human activities, etc. In this work, we introduce privacy-preserving embodied agent learning for the task of Vision-and-Language Navigation (VLN), where an embodied agent navigates house environments by following natural language instructions. We view each house environment as a local client, which shares nothing other than local updates with the cloud server and other clients, and propose a novel Federated Vision-and-Language Navigation (FedVLN) framework to protect data privacy during both training and pre-exploration. Particularly, we propose a decentralized federated training strategy to limit the data of each client to its local model training and a federated pre-exploration method to do partial model aggregation to improve model generalizability to unseen environments. Extensive results on R2R and RxR datasets show that, decentralized federated training achieve comparable results with centralized training while protecting seen environment privacy, and federated pre-exploration significantly outperforms centralized pre-exploration while preserving unseen environment privacy. Code is available at https://github.com/eric-ai-lab/FedVLN.
更多查看译文
关键词
Privacy-preserving Embodied AI,Vision-and-language navigation,Federated learning
AI 理解论文
溯源树
样例
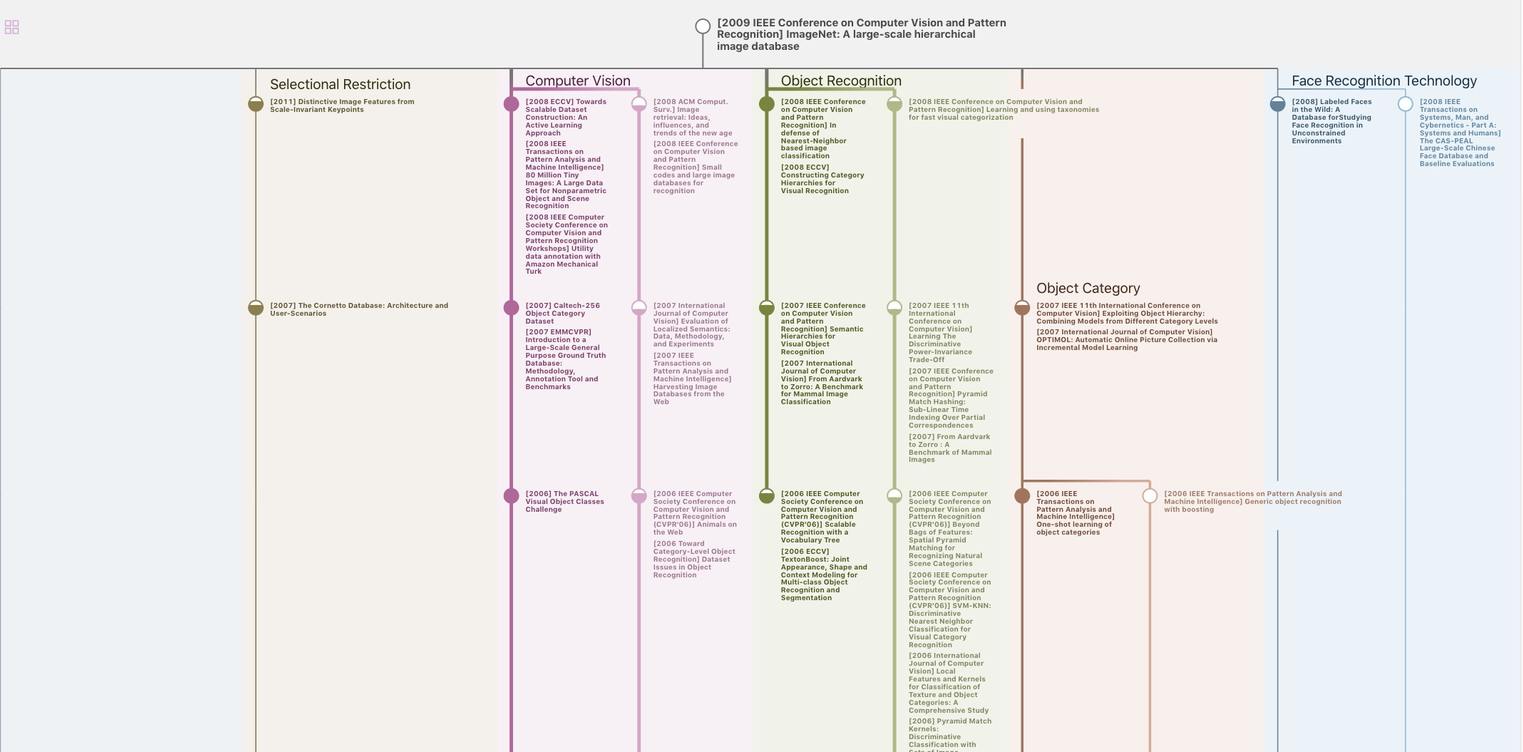
生成溯源树,研究论文发展脉络
Chat Paper
正在生成论文摘要