Machine-Learning Based Objective Function Selection for Community Detection
Cyber Security, Cryptology, and Machine Learning(2022)
摘要
NECTAR, a Node-centric ovErlapping Community deTection AlgoRithm, presented by Cohen et al., chooses dynamically between two objective functions which to optimize, based on the network on which it is invoked. It was shown that this approach outperforms six state-of-the-art algorithms for overlapping community detection. In this work, we present NECTAR-ML, an extension of the NECTAR algorithm that uses a machine-learning based model for automating the selection of the objective function, trained and evaluated on a dataset of 15,755 synthetic and 7 real-world networks. Our analysis shows that in approximately 90% of the cases our model was able to successfully select the correct objective function. We conducted a competitive analysis of NECTAR and NECTAR-ML. NECTAR-ML was shown to significantly outperform NECTAR’s ability to select the best objective function. We also conducted a competitive analysis of NECTAR-ML and two additional state-of-the-art multi-objective evolutionary community detection algorithms. NECTAR-ML outperformed both algorithms in terms of average detection quality. Multi-objective evolutionary algorithms are considered to be the most popular approach to solve multi-objective optimization problems and the fact that NECTAR-ML significantly outperforms them demonstrates the effectiveness of ML-based objective function selection.
更多查看译文
关键词
Community detection, Complex networks, Machine learning, Overlapping community detection, Supervised learning
AI 理解论文
溯源树
样例
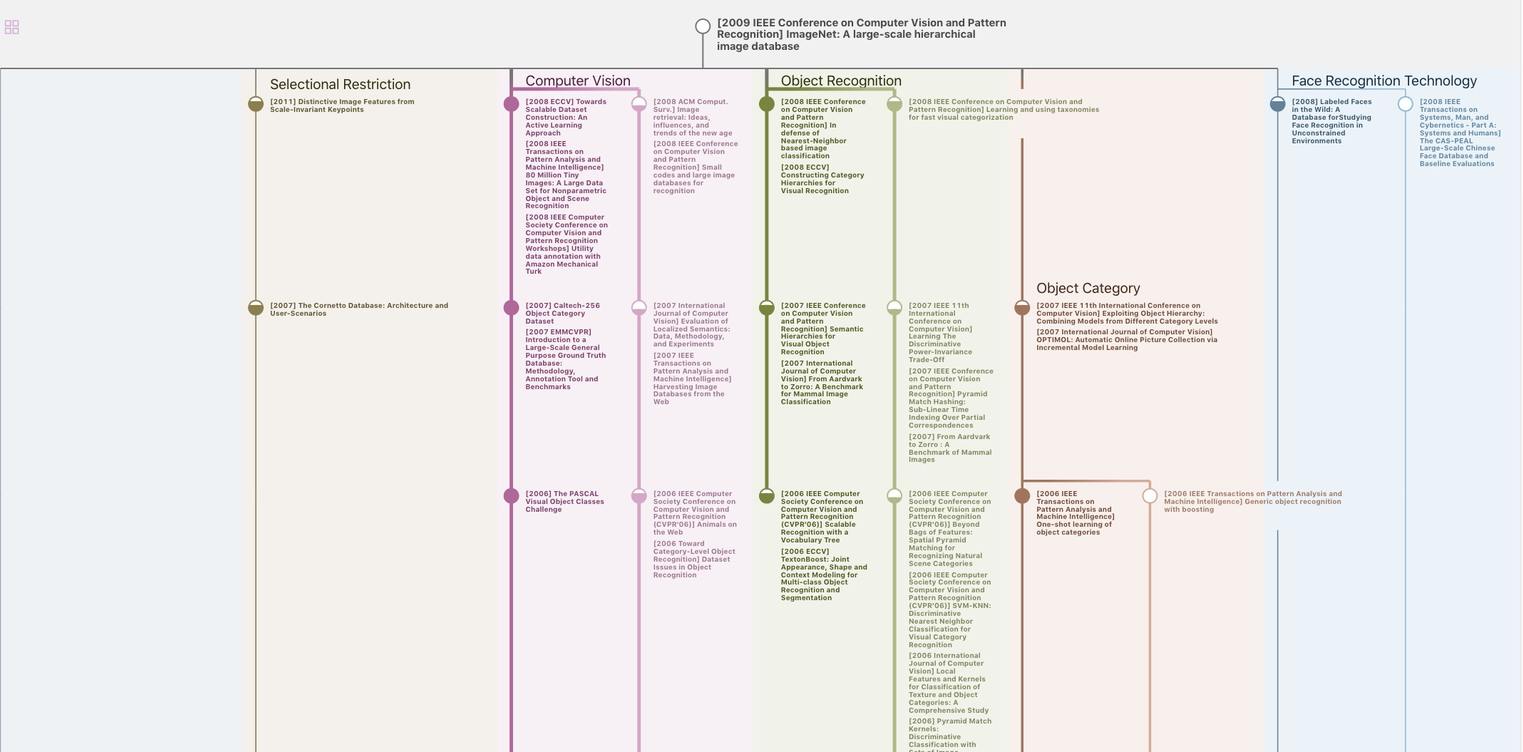
生成溯源树,研究论文发展脉络
Chat Paper
正在生成论文摘要