Bi-level Optimization for hyperparameters in Nonnegative Matrix Factorizations
arxiv(2022)
摘要
Hyperparameters (HPs) Optimization in machine learning algorithms represents an open problem with a direct impact on algorithm performances and on the knowledge extraction process from data. Matrix Decompositions (MDs) has recently gained more attention in data science as mathematical techniques able to capture latent information embedded in large datasets. MDs can be formalized as penalized optimization problems in which the tuning of the penalization HPs represents an issue. Current literature panorama does not provide any general framework addressing optimally conditions for the best configuration of HPs. In this work, we focus on the role the HPs play in the penalized Nonnegative Matrix Factorizations (NMF) context and on the importance of their correct selection. Using a bi-level optimization approach, HPs selection is considered from an optimization point of view and their choice is directly incorporated in the unsupervised algorithm as a part of the updating process. Either theorems of existence and convergence of numerical solutions, under appropriate assumptions, are studied and a novel algorithm, namely AltBi-Alternate bi-level Algorithm, is proposed to tune the penalization HP in NMF problems.
更多查看译文
AI 理解论文
溯源树
样例
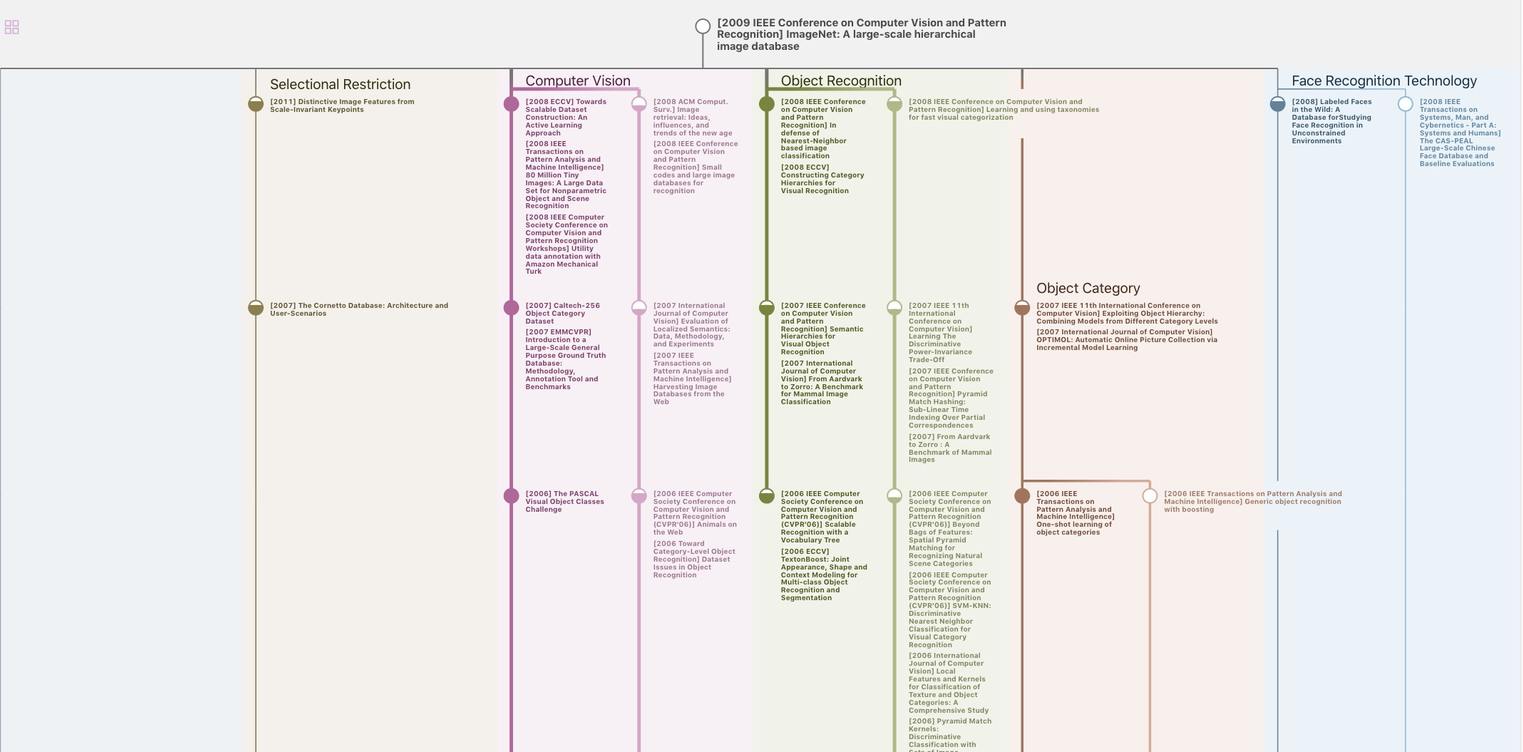
生成溯源树,研究论文发展脉络
Chat Paper
正在生成论文摘要