Stochastic Gauss–Newton Algorithms for Online PCA
arxiv(2023)
摘要
In this paper, we propose a stochastic Gauss–Newton (SGN) algorithm to study the online principal component analysis (OPCA) problem, which is formulated by using the symmetric low-rank product model for dominant eigenspace calculation. Compared with existing OPCA solvers, SGN is of improved robustness with respect to the varying input data and algorithm parameters. In addition, turning to an evaluation of data stream based on approximated objective functions, we develop a new adaptive stepsize strategy for SGN which requires no priori knowledge of the input data, and numerically illustrate its comparable performance with SGN adopting the manaully-tuned diminishing stepsize. Without assuming the eigengap to be positive, we also establish the global and optimal convergence rate of SGN with the specified stepsize using the diffusion approximation theory.
更多查看译文
关键词
Online principal component analysis, Symmetric low-rank product, Gauss–Newton method, Adaptive stepsize
AI 理解论文
溯源树
样例
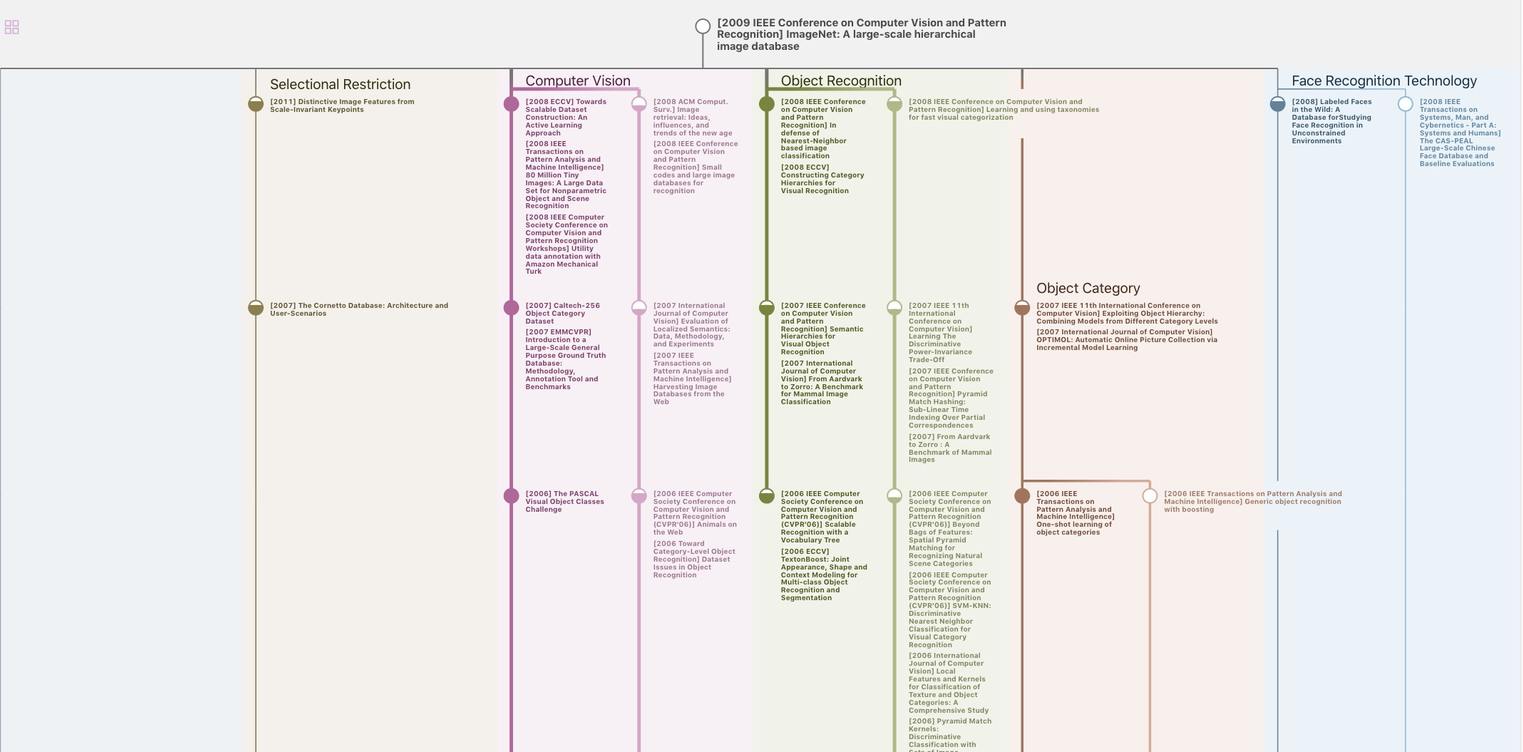
生成溯源树,研究论文发展脉络
Chat Paper
正在生成论文摘要