Modelling the galaxy-halo connection with semi-recurrent neural networks
arxiv(2022)
摘要
We present an artificial neural network design in which past and present-day properties of dark matter halos and their local environment are used to predict time-resolved star formation histories and stellar metallicity histories of central and satellite galaxies. Using data from the IllustrisTNG simulations, we train a TensorFlow-based neural network with two inputs: a standard layer with static properties of the dark matter halo, such as halo mass and starting time; and a recurrent layer with variables such as overdensity and halo mass accretion rate, evaluated at multiple time steps from $0 \leq z \lesssim 20$. The model successfully reproduces key features of the galaxy halo connection, such as the stellar-to-halo mass relation, downsizing, and colour bimodality, for both central and satellite galaxies. We identify mass accretion history as crucial in determining the geometry of the star formation history and trends with halo mass such as downsizing, while environmental variables are important indicators of chemical enrichment. We use these outputs to compute optical spectral energy distributions, and find that they are well matched to the equivalent results in IllustrisTNG, recovering observational statistics such as colour bimodality and mass-magnitude diagrams.
更多查看译文
关键词
galaxies: evolution,galaxies: formation,galaxies: haloes,galaxies: star formation
AI 理解论文
溯源树
样例
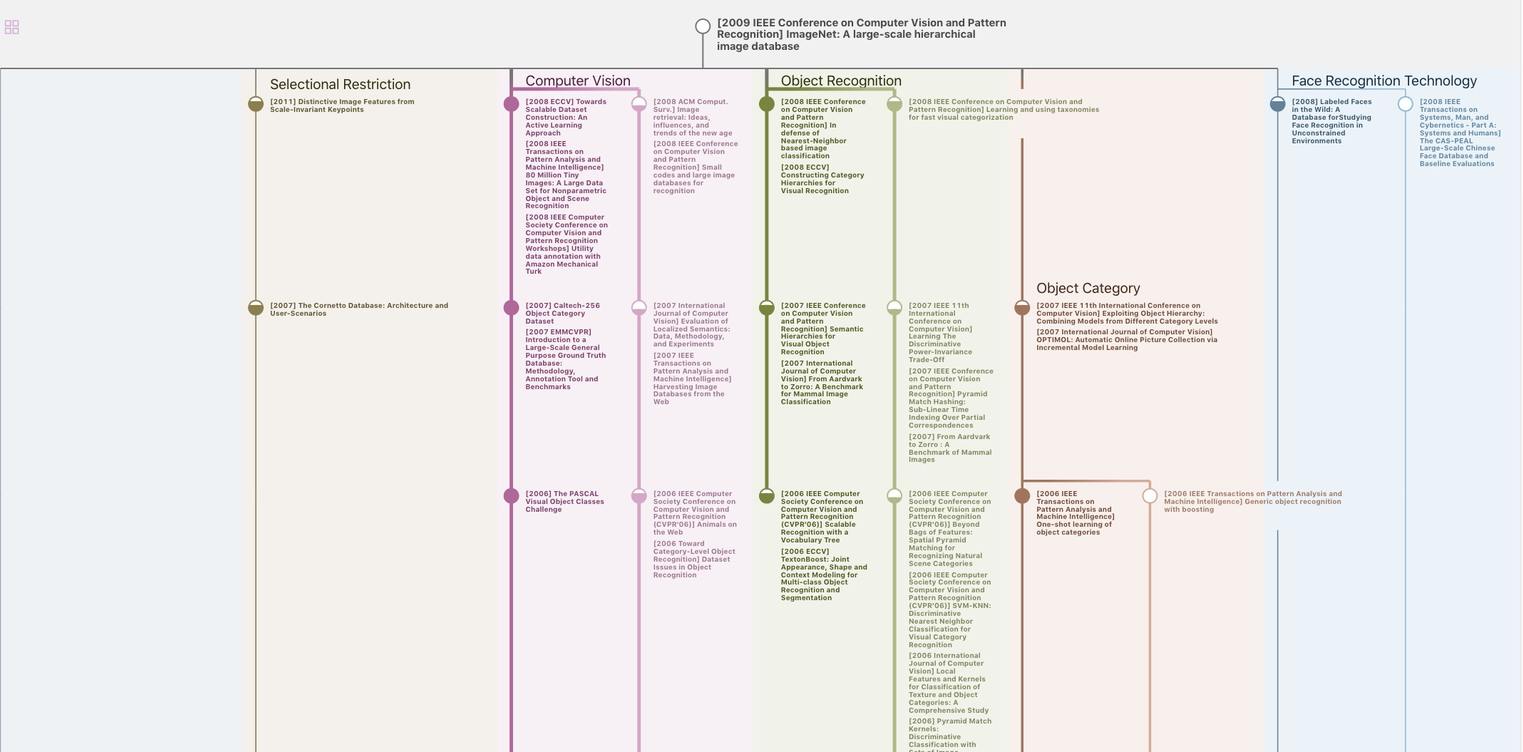
生成溯源树,研究论文发展脉络
Chat Paper
正在生成论文摘要