Detection and Analysis of Attention Errors in Sequence-to-Sequence Text-to-Speech.
Interspeech(2021)
摘要
Sequence-to-sequence speech synthesis models are notorious for gross errors such as skipping and repetition, commonly associated with failures in the attention mechanism. While a lot has been done to improve attention and decrease errors, this paper focuses instead on automatic error detection and analysis. We evaluated three objective metrics against error detection scores collected by human listening. All metrics were derived from the synthesised attention matrix alone and do not require a reference signal, relying on the expectation that errors occur when attention is dispersed or insufficient. Using one of this metrics as an analysis tool, we observed that gross errors are more likely to occur in longer sentences and in sentences with punctuation marks that indicate pause or break. We also found that mechanisms such as forcibly incremented attention have the potential for decreasing gross errors but to the detriment of naturalness. The results of the error detection evaluation revealed that two of the evaluated metrics were able to detect errors with a relatively high success rate, obtaining F-scores of up to 0.89 and 0.96.
更多查看译文
关键词
speech synthesis,attention,sequence-tosequence modelling
AI 理解论文
溯源树
样例
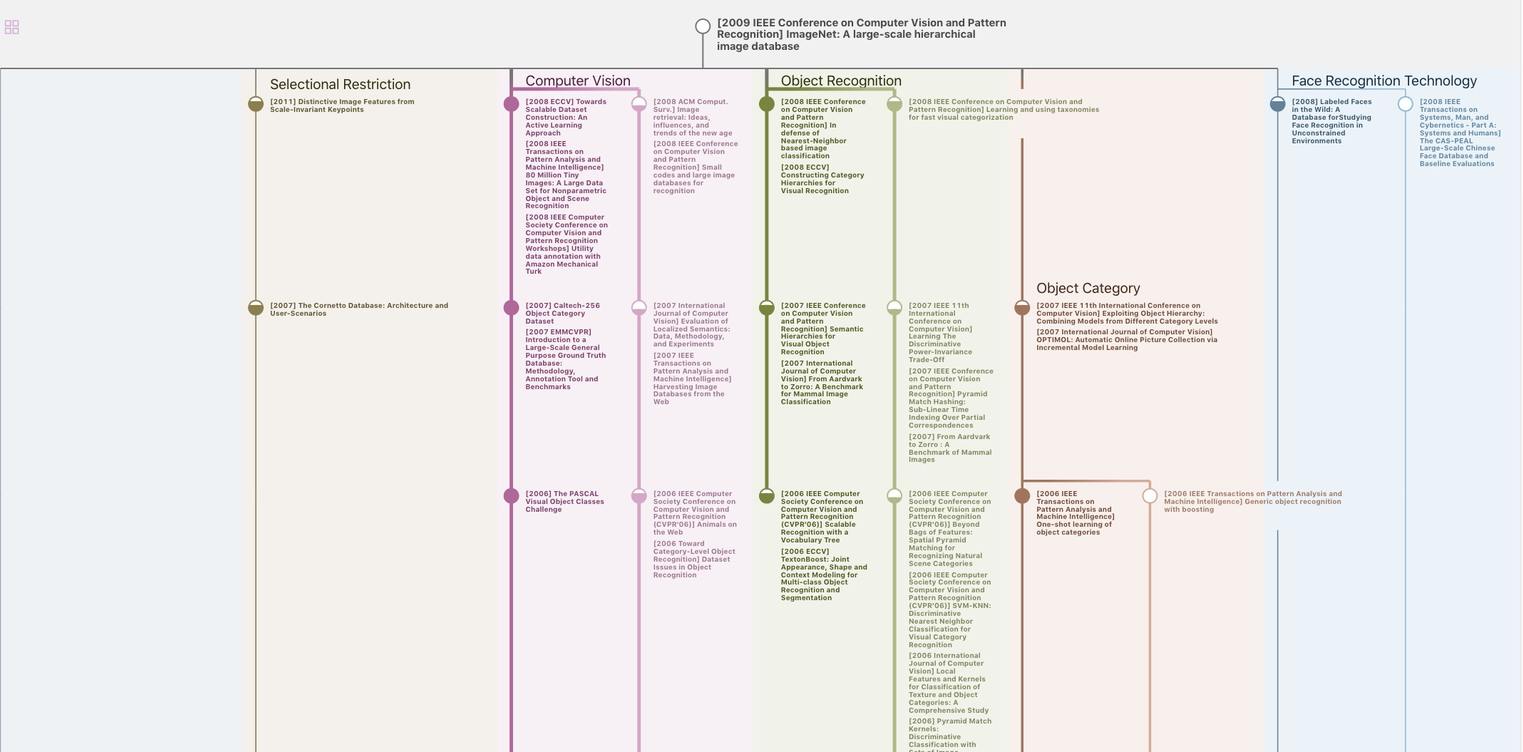
生成溯源树,研究论文发展脉络
Chat Paper
正在生成论文摘要