An Untrained One-layer Convolutional Network-based Method for Line Spectral Estimation.
ACSCC(2021)
摘要
Many signals of practical interest can be represented as a sparse linear combination of elements from some dictionary, such as spectrally sparse signals. In recent years, denoising such signals has been heavily studied in line spectral estimation. Motivated by recent work on the Deep Image Prior, we propose a very simple convolutional network with only one layer to denoise a spectrally sparse signal from its noisy uniform time samples. In particular, we fit the one-layer convolutional network to the noisy measurements via gradient descent with zero initialization and early stopping. Unlike other deep learning-based methods proposed for line spectral estimation, we emphasize that there is no need to train any network in our proposed method. We also provide theoretical analysis to explain why the proposed method works well in signal denoising. Moreover, simulations indicate that our approach significantly outperforms the atomic norm minimization-based method in both accuracy and efficiency for signals with only low-frequency components.
更多查看译文
关键词
Line spectral estimation,spectrally sparse signals,convolutional neural network,signal denoising,gradient descent
AI 理解论文
溯源树
样例
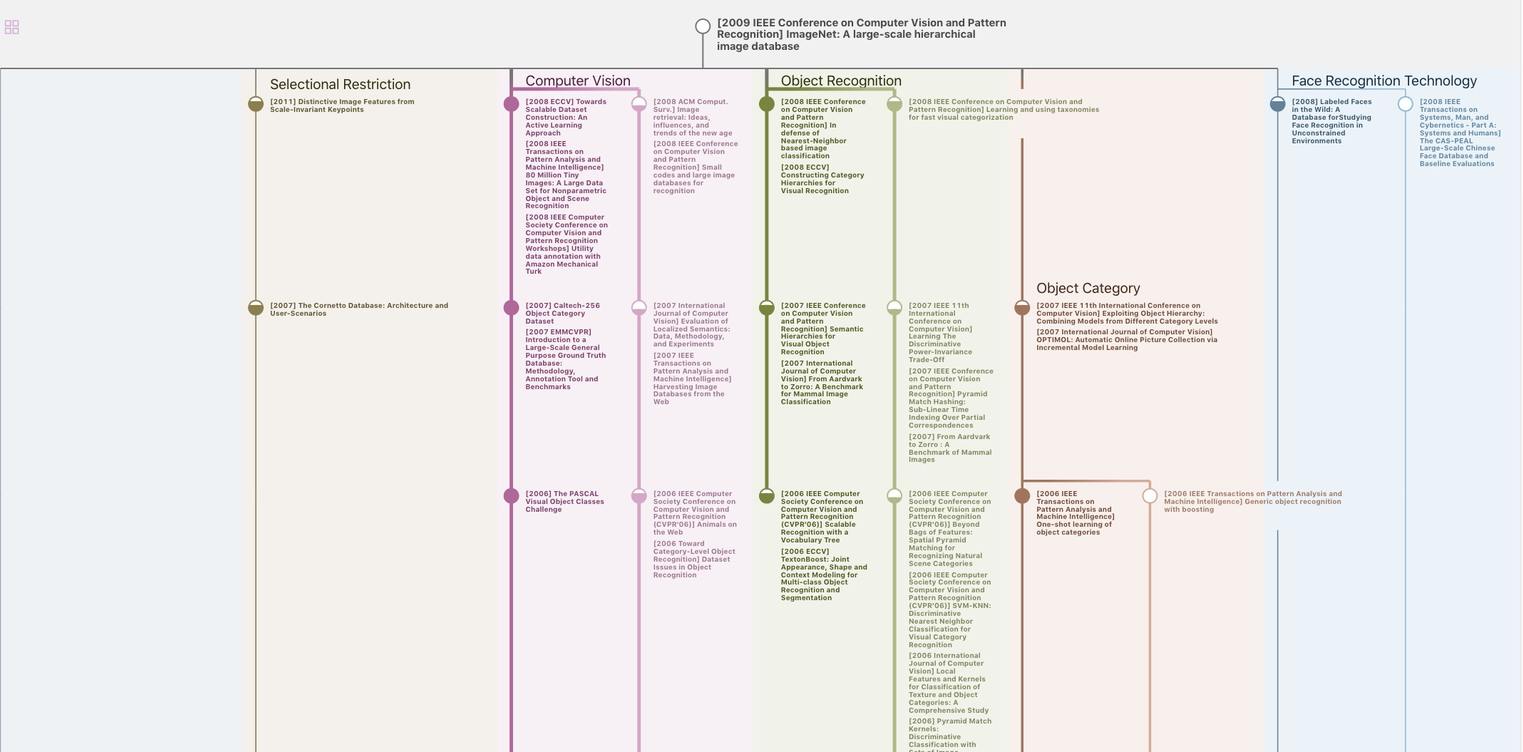
生成溯源树,研究论文发展脉络
Chat Paper
正在生成论文摘要