Unsupervised Denoising Feature Learning for Classification of Corrupted Images
BIG DATA RESEARCH(2022)
摘要
Numerous corrupted or noisy images are generated owing to the problems in sampling and transmission. Facing the category of high-dimensional image data, directly operating like classification is inefficient and the accuracy is normally not ideal. Consequently, dimensionality reduction is a critical process. After dimensionality reduction by classical algorithms, the classification efficiency can be improved. However, the problem of low accuracy still exists. In this paper, an unsupervised denoising feature learning model is proposed to learn a discriminative feature representation from corrupted images. First, the relationship between initial data and low-dimensional feature representation is explored. We introduce several classical dimensionality reduction algorithms. Second, the working mechanism of denoising feature learning model is proved. Due to its structural characteristics, denoising model can extract robust features from partially destroyed data to represent the original data. It demonstrates proposed model can extract essential information from noisy image to decrease reconstruction error. Finally, extensive experiments demonstrate that the features representation obtained by the proposed method outperforms those obtained by the existing classical dimensionality reduction algorithms. (C) 2021 Elsevier Inc. All rights reserved.
更多查看译文
关键词
Dimensionality reduction, Image classification, Corrupted data, Feature representation, Denoising learning
AI 理解论文
溯源树
样例
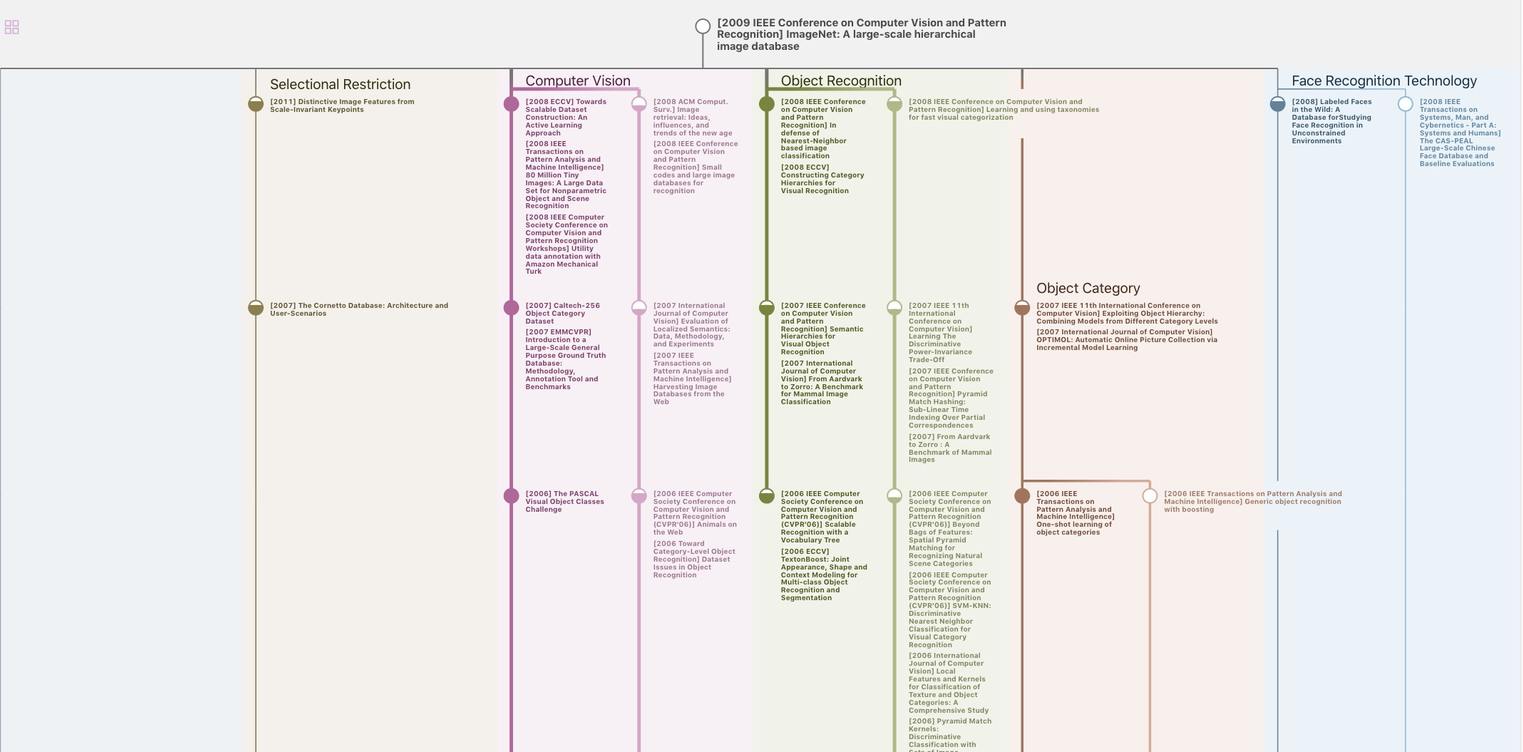
生成溯源树,研究论文发展脉络
Chat Paper
正在生成论文摘要