Embedding Semantic Anchors to Guide Topic Models on Short Text Corpora
BIG DATA RESEARCH(2022)
摘要
Documents on the social media platform Twitter are formulated in short and simple style, instead of being written extensively and elaborately. Further, the core message of a post is often encoded into characteristic phrases called hashtags. These hashtags illustrate the semantics of a post or tie it to a specific topic. In this paper, we propose multiple approaches of using hashtags and their surrounding texts to improve topic modeling of short texts. We use transfer learning by applying a pre-trained word embedding of hashtags to derive preliminary topics. These function as supervising information, or seed topics and are passed to Archetypal LDA (A-LDA), a recent variant of Latent Dirichlet Allocation. We demonstrate the effectiveness of our approach using a large corpus of posts exemplarily on Twitter. Our approaches improve the topic model's qualities in terms of various quantitative metrics. Moreover, the presented algorithms used to extract seed topics can be utilized as form of lightweight topic model by themselves. Hence, our approaches create additional analytical opportunities and can help to gain a more detailed understanding of what people are talking about on social media. By using big data in terms of millions of tweets for preprocessing and fine-tuning, we enable the classification algorithm to produce topics that are very coherent to the reader. (C) 2021 Elsevier Inc. All rights reserved.
更多查看译文
关键词
Topic modeling, Short text, Word embedding, Transfer learning, Big data
AI 理解论文
溯源树
样例
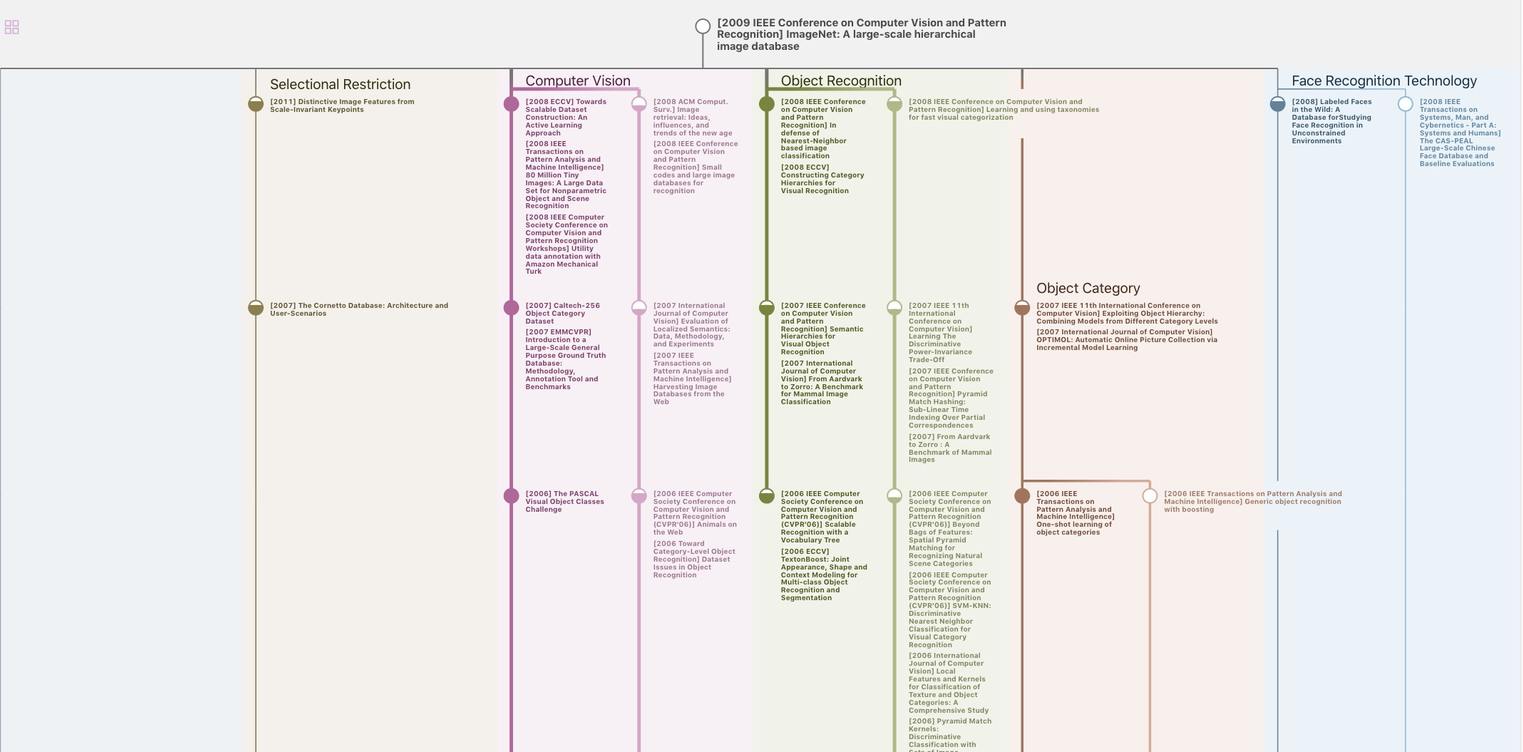
生成溯源树,研究论文发展脉络
Chat Paper
正在生成论文摘要