A population-based game-theoretic optimizer for the minimum weighted vertex cover
APPLIED SOFT COMPUTING(2022)
摘要
Toward higher solution efficiency and faster computation, this paper addresses the minimum weighted vertex cover (MWVC) problem by introducing game theory into iterated optimization and proposing a population-based game-theoretic optimizer (PGTO). A group of candidate solutions are iterated through the specially designed procedures of swarm evolution (SE), learning in games (LIG), and local search (LS) successively. Within the framework of potential game theory, we prove that LIG computes in finite time Nash equilibria that represent vertex cover solutions where no redundant nodes exist. Moreover, theoretical analysis is presented that by exchanging the actions of certain neighbours, LS is capable of generating better results upon the input Nash equilibrium. Through intensive numerical experiments, we show that while enlarging the population size could boost the global objective, a mutation probability between 0.05 and 0.2 is more likely to provide the best performance. Comparison experiments against the state of the art demonstrate the superiority of the presented methodology, both in terms of solution quality and computation speed. (C) 2021 Elsevier B.V. All rights reserved.
更多查看译文
关键词
Minimum weighted vertex cover (MWVC), Combinatorial optimization, Learning in games, Nash equilibrium, Exploration and exploitation
AI 理解论文
溯源树
样例
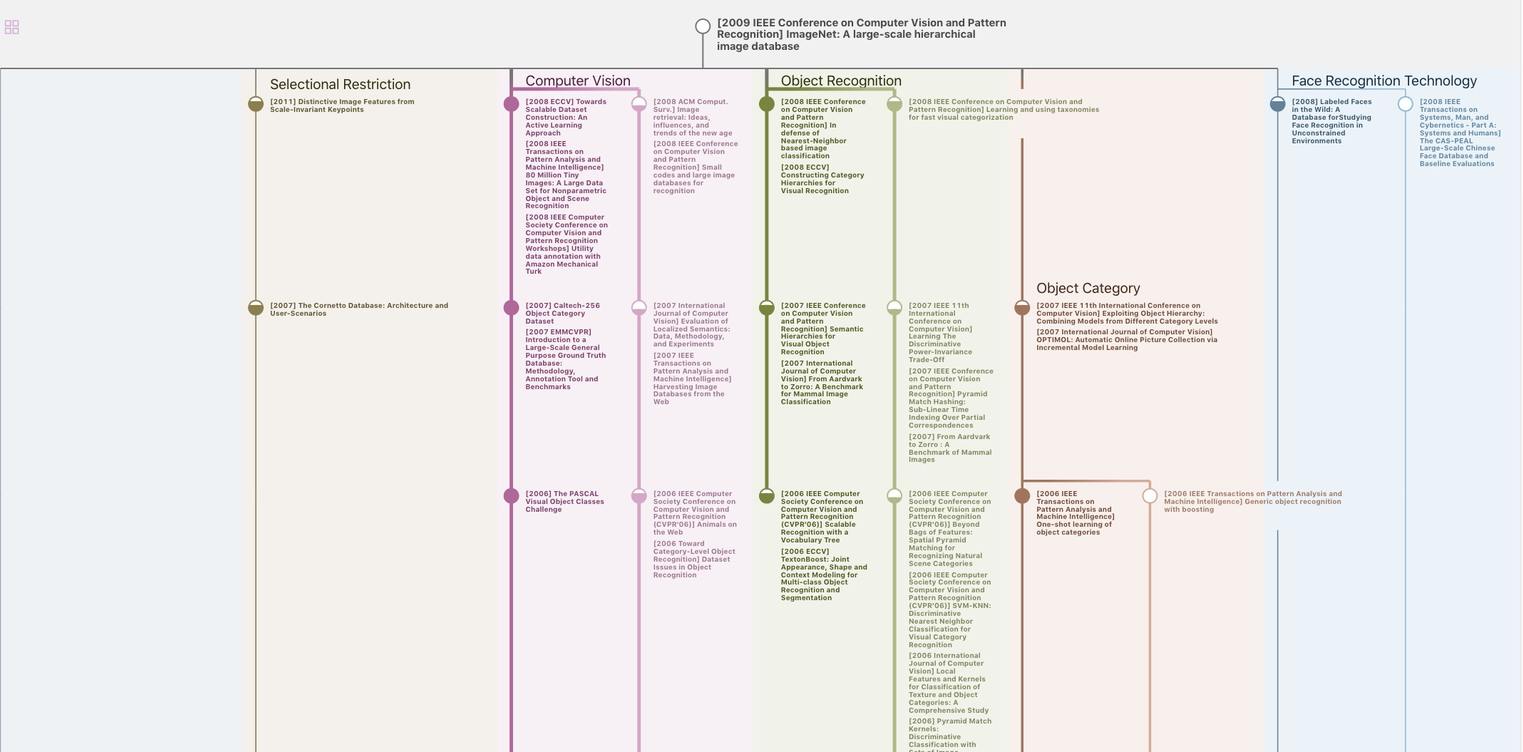
生成溯源树,研究论文发展脉络
Chat Paper
正在生成论文摘要