A Robust and Reliable Point Cloud Recognition Network Under Rigid Transformation
IEEE TRANSACTIONS ON INSTRUMENTATION AND MEASUREMENT(2022)
摘要
Point cloud recognition is an essential task in industrial robotics and autonomous driving. Recently, several point cloud processing models have achieved state-of-the-art performances. However, these methods lack rotation robustness, and their performances degrade severely under random rotations, failing to extend to real-world scenarios with varying orientations. To this end, we propose a method named self-contour-based transformation (SCT), which can be flexibly integrated into various existing point cloud recognition models against arbitrary rotations. SCT provides efficient rotation and translation invariance by introducing contour-aware transformation (CAT), which linearly transforms the Cartesian coordinates of points to translation and rotation-invariant representations. We prove that CAT is a rotation- and translation-invariant transformation based on the theoretical analysis. Furthermore, the frame alignment module is proposed to enhance the discriminative feature extraction by capturing contours and transforming self-contour-based frames into intraclass frames. Extensive experimental results show that SCT outperforms the state-of-the-art approaches under arbitrary rotations in effectiveness and efficiency on synthetic and real-world benchmarks. Furthermore, the robustness and generality evaluations indicate that SCT is robust and is applicable to various point cloud processing models, which highlights the superiority of SCT in industrial applications.
更多查看译文
关键词
Point cloud compression, Feature extraction, Three-dimensional displays, Convolution, Robustness, Task analysis, Perturbation methods, 3-D point clouds, classification, robustness, rotation and translation invariance, segmentation
AI 理解论文
溯源树
样例
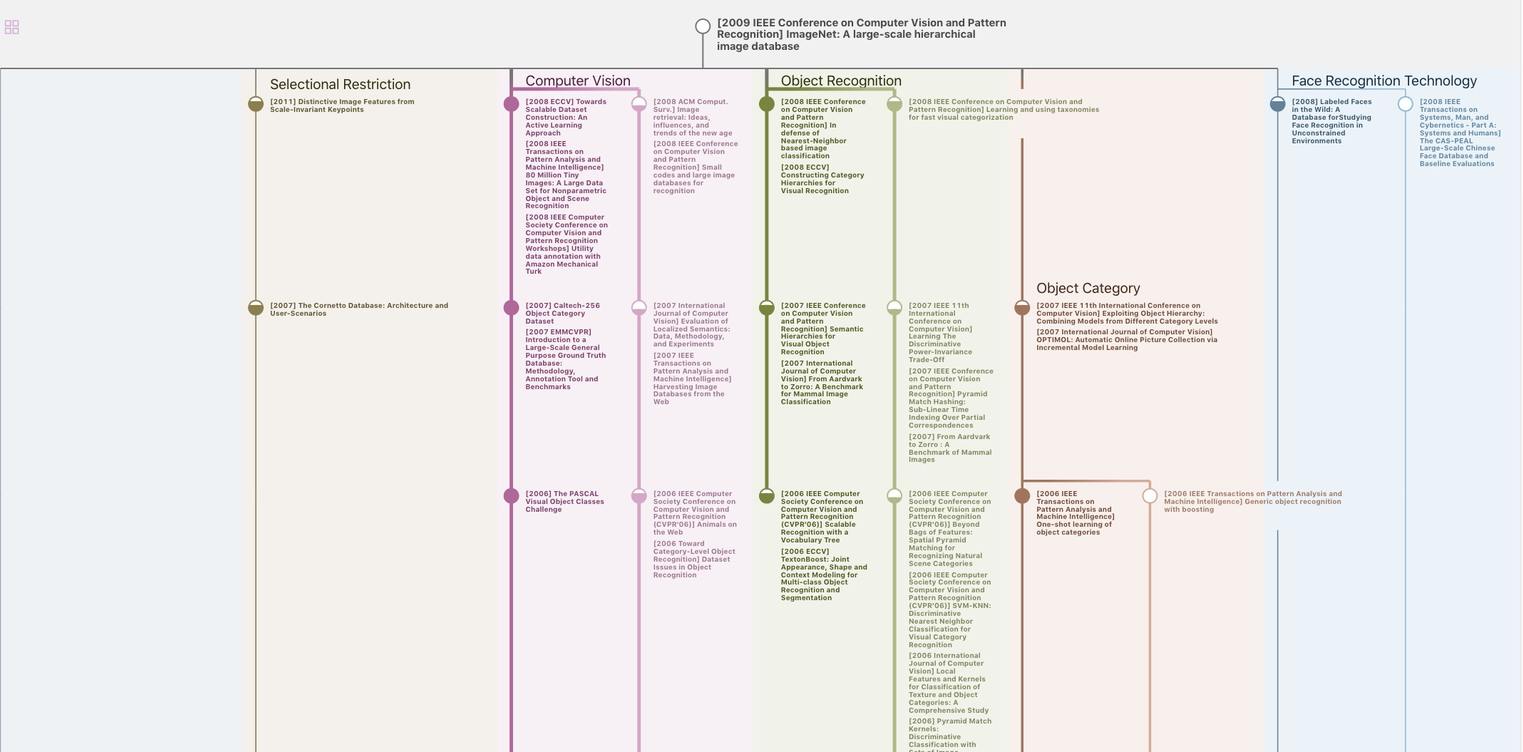
生成溯源树,研究论文发展脉络
Chat Paper
正在生成论文摘要