TFA-Net: A Deep Learning-Based Time-Frequency Analysis Tool
IEEE transactions on neural networks and learning systems(2023)
摘要
Recently, synchrosqueenzing transform (SST)-based time-frequency analysis (TFA) methods have been developed for achieving the highly concentrated TF representation (TFR). However, SST-based methods suffer from two drawbacks. The first one is that the TFRs are unsatisfactory when dealing with the multicomponent signals, the instantaneous frequencies (IFs) of which are closely adjacent or intersected. Besides, the exhaustive adjustment of window length is required for SST-based methods to obtain the optimal TFR. To tackle these problems, in this article, we first analyze the concentration of TFRs for SST-based methods. A deep learning (DL)-based end-to-end replacement scheme for SST-based methods, named TFA-Net, is then proposed, which learns complete basis functions to obtain various TF characteristics of time series. The 2-D filter kernels are subsequently used for energy concentration. Different from the two-step SST-based methods where the TF transform and energy concentration are separated, the proposed end-to-end architecture makes the basis functions used for extracting TF features more beneficial to energy concentration. The comprehensive numerical experiments are conducted to demonstrate the effectiveness of the TFA-Net. The applications of the proposed method to real-world vital signs, undersea voices and micro-Doppler signatures show its great potential in analyzing nonstationary signals.
更多查看译文
关键词
Transforms,Time-frequency analysis,Time-domain analysis,Trajectory,Feature extraction,Kernel,Frequency modulation,Deep learning (DL),micro-Doppler signatures,signal processing,time-frequency analysis (TFA),vital signs
AI 理解论文
溯源树
样例
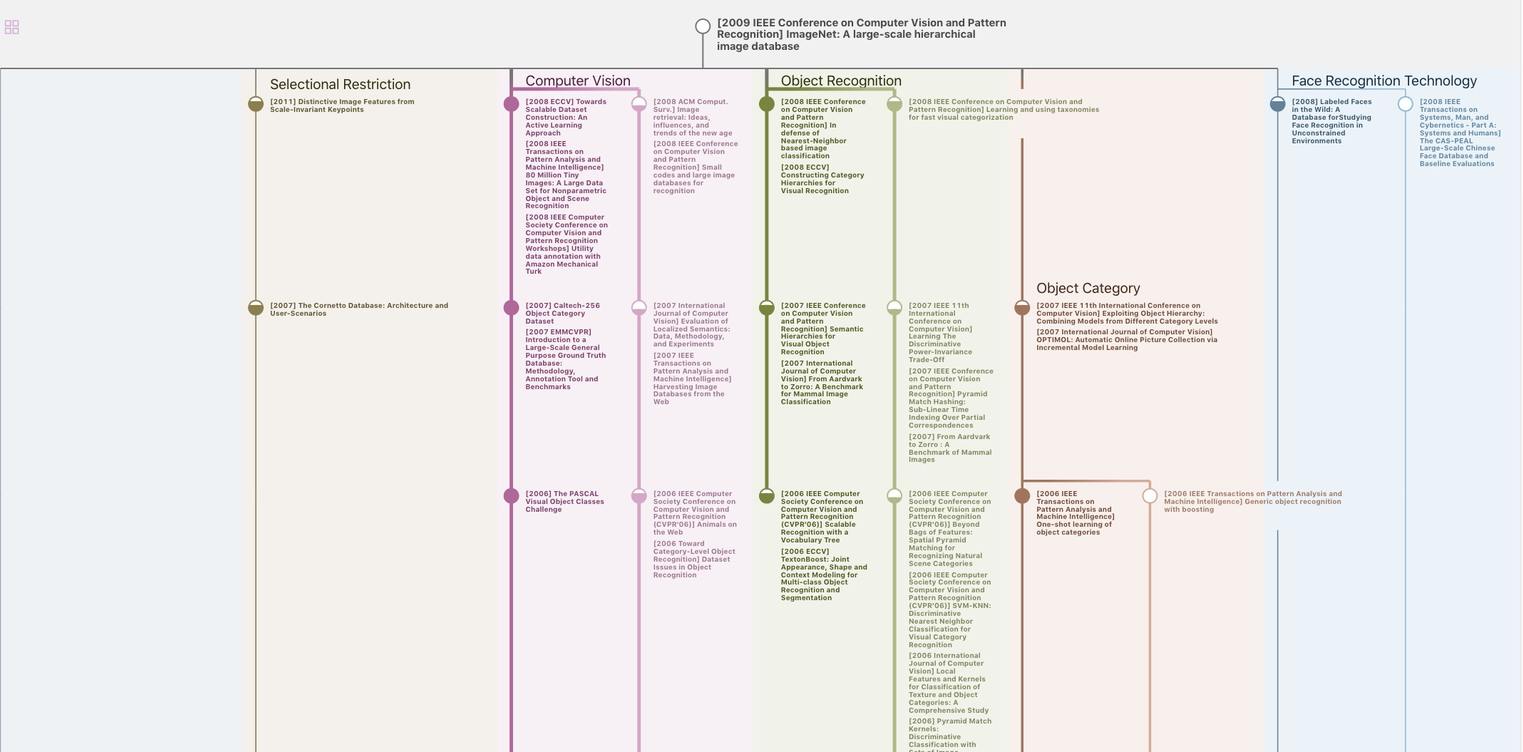
生成溯源树,研究论文发展脉络
Chat Paper
正在生成论文摘要