Representation Bias in Data: A Survey on Identification and Resolution Techniques
arxiv(2023)
摘要
Data-driven algorithms are only as good as the data they work with, while datasets, especially social data, often fail to represent minorities adequately. Representation Bias in data can happen due to various reasons, ranging from historical discrimination to selection and sampling biases in the data acquisition and preparation methods. Given that "bias in, bias out," one cannot expect AI-based solutions to have equitable outcomes for societal applications, without addressing issues such as representation bias. While there has been extensive study of fairness in machine learning models, including several review papers, bias in the data has been less studied. This article reviews the literature on identifying and resolving representation bias as a feature of a dataset, independent of how consumed later. The scope of this survey is bounded to structured (tabular) and unstructured (e.g., image, text, graph) data. It presents taxonomies to categorize the studied techniques based on multiple design dimensions and provides a side-by-side comparison of their properties. There is still a long way to fully address representation bias issues in data. The authors hope that this survey motivates researchers to approach these challenges in the future by observing existing work within their respective domains.
更多查看译文
关键词
Responsible data science,fairness in machine learning,data equity systems,data-centric AI,AI-ready data
AI 理解论文
溯源树
样例
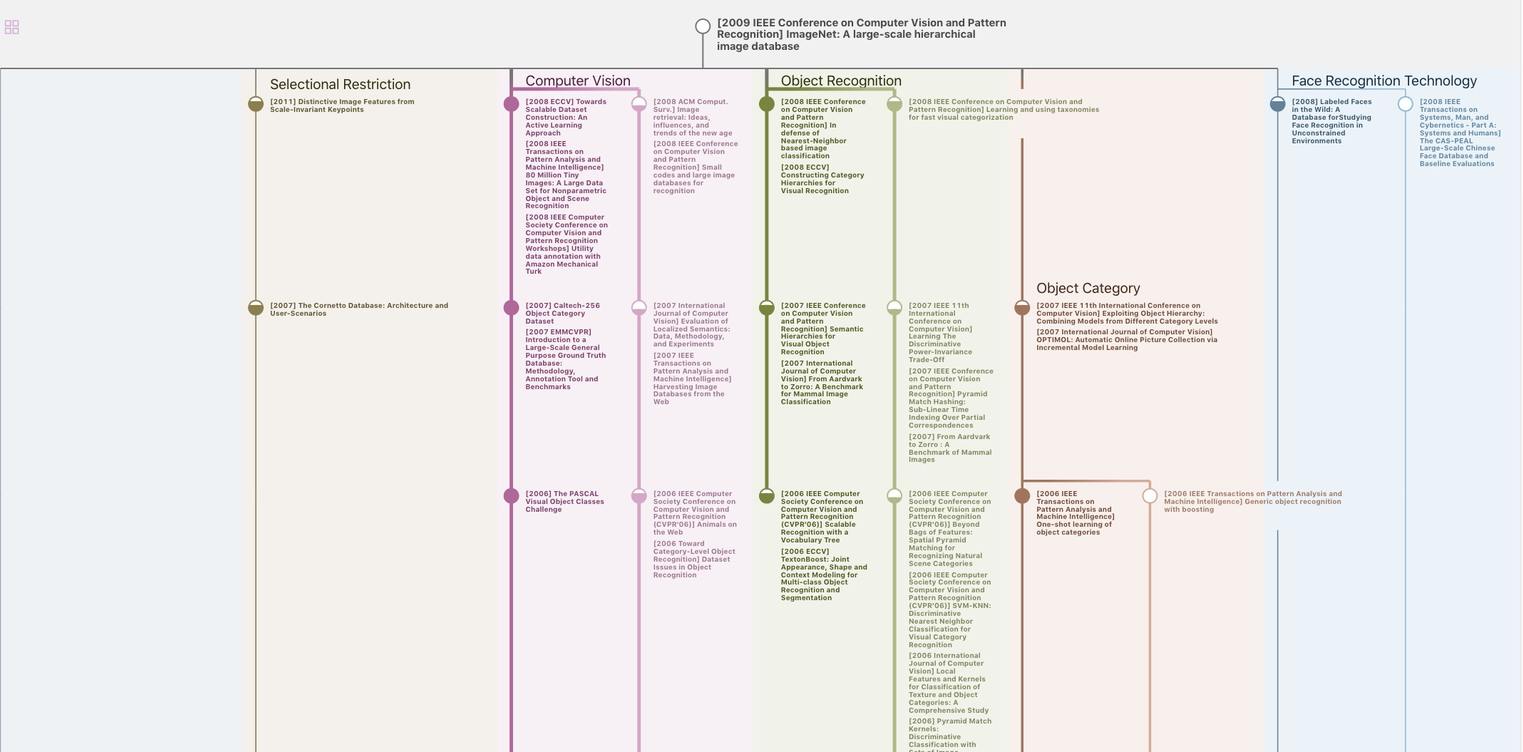
生成溯源树,研究论文发展脉络
Chat Paper
正在生成论文摘要