Task-guided Disentangled Tuning for Pretrained Language Models
FINDINGS OF THE ASSOCIATION FOR COMPUTATIONAL LINGUISTICS (ACL 2022)(2022)
摘要
Pretrained language models (PLMs) trained on large-scale unlabeled corpus are typically finetuned on task-specific downstream datasets, which have produced state-of-the-art results on various NLP tasks. However, the data discrepancy issue in domain and scale makes finetuning fail to efficiently capture task-specific patterns, especially in the low data regime. To address this issue, we propose Task-guided Disentangled Tuning (TDT) for PLMs, which enhances the generalization of representations by disentangling task-relevant signals from the entangled representations. For a given task, we introduce a learnable confidence model to detect indicative guidance from context, and further propose a disentangled regularization to mitigate the over-reliance problem. Experimental results on GLUE and CLUE benchmarks show that TDT gives consistently better results than fine-tuning with different PLMs, and extensive analysis demonstrates the effectiveness and robustness of our method. Code is available at https://github.com/lemon0830/TDT.
更多查看译文
关键词
pretrained language models,disentangled tuning,task-guided
AI 理解论文
溯源树
样例
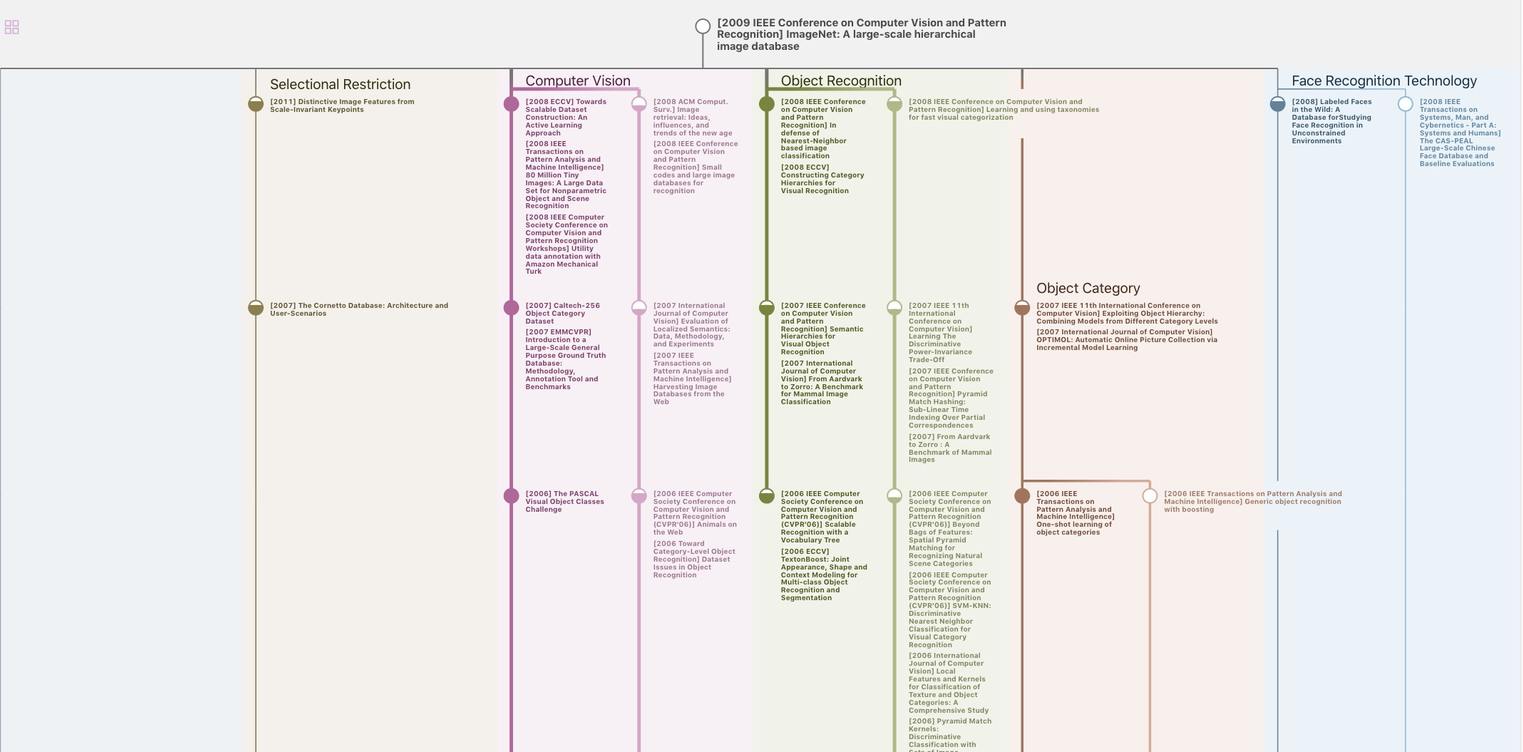
生成溯源树,研究论文发展脉络
Chat Paper
正在生成论文摘要