Robust model predictive control with polytopic model uncertainty through System Level Synthesis
AUTOMATICA(2024)
摘要
We propose a robust model predictive control (MPC) method for discrete -time linear systems with polytopic model uncertainty and additive disturbances. Optimizing over linear time -varying (LTV) state feedback controllers has been successfully used for robust MPC when only additive disturbances are present. However, it is challenging to design LTV state feedback controllers in the face of model uncertainty whose effects are difficult to bound. To address this issue, we propose a novel approach to over -approximate the effects of both model uncertainty and additive disturbances by a filtered additive disturbance signal. Using the System Level Synthesis framework, we jointly search for robust LTV state feedback controllers and the bounds on the effects of uncertainty online, which allows us to reduce the conservatism and minimize an upper bound on the worst -case cost in robust MPC. We provide a comprehensive numerical comparison of our method and representative robust MPC methods from the literature. Numerical examples demonstrate that our proposed method can significantly reduce the conservatism over a wide range of uncertainty parameters with comparable computational effort as the baseline methods. (c) 2023 Published by Elsevier Ltd.
更多查看译文
关键词
Robust model predictive control,System Level Synthesis,Robust control
AI 理解论文
溯源树
样例
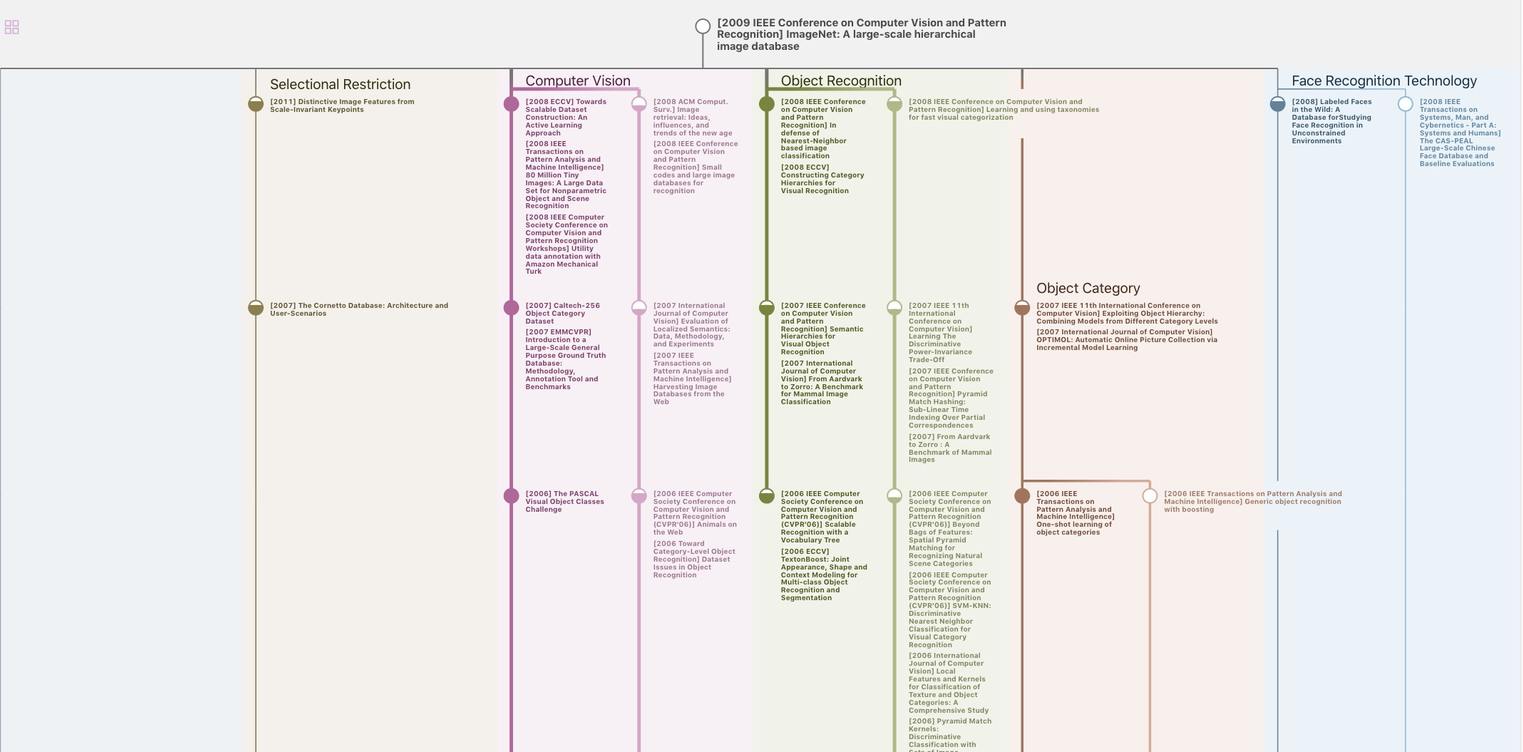
生成溯源树,研究论文发展脉络
Chat Paper
正在生成论文摘要