Open-Vocabulary One-Stage Detection with Hierarchical Visual-Language Knowledge Distillation
IEEE Conference on Computer Vision and Pattern Recognition(2022)
摘要
Open- vocabulary object detection aims to detect novel object categories beyond the training set. The advanced open- vocabulary two-stage detectors employ instance-level visual-to- visual knowledge distillation to align the visual space of the detector with the semantic space of the Pre-trained Visual-Language Model (PVLM). However, in the more efficient one-stage detector, the absence of class-agnostic object proposals hinders the knowledge distil-lation on unseen objects, leading to severe performance degradation. In this paper, we propose a hierarchical visual-language knowledge distillation method, i.e., Hi-erKD, for open-vocabulary one-stage detection. Specifi-cally, a global-level knowledge distillation is explored to transfer the knowledge of unseen categories from the PVLM to the detector. Moreover, we combine the proposed global-level knowledge distillation and the common instance-level knowledge distillation to learn the knowledge of seen and unseen categories simultaneously. Extensive experiments on MS-COCO show that our method significantly surpasses the previous best one-stage detector with 11.9% and 6.7% AP50 gains under the zero-shot detection and generalized zero-shot detection settings, and reduces the AP 50 performance gap from 14% to 7.3% compared to the best two-stage detector. Code will be released at this url 1 1 https://qithub.com/menqqiDyanqqe/HierKD.
更多查看译文
关键词
Recognition: detection,categorization,retrieval, Vision + language
AI 理解论文
溯源树
样例
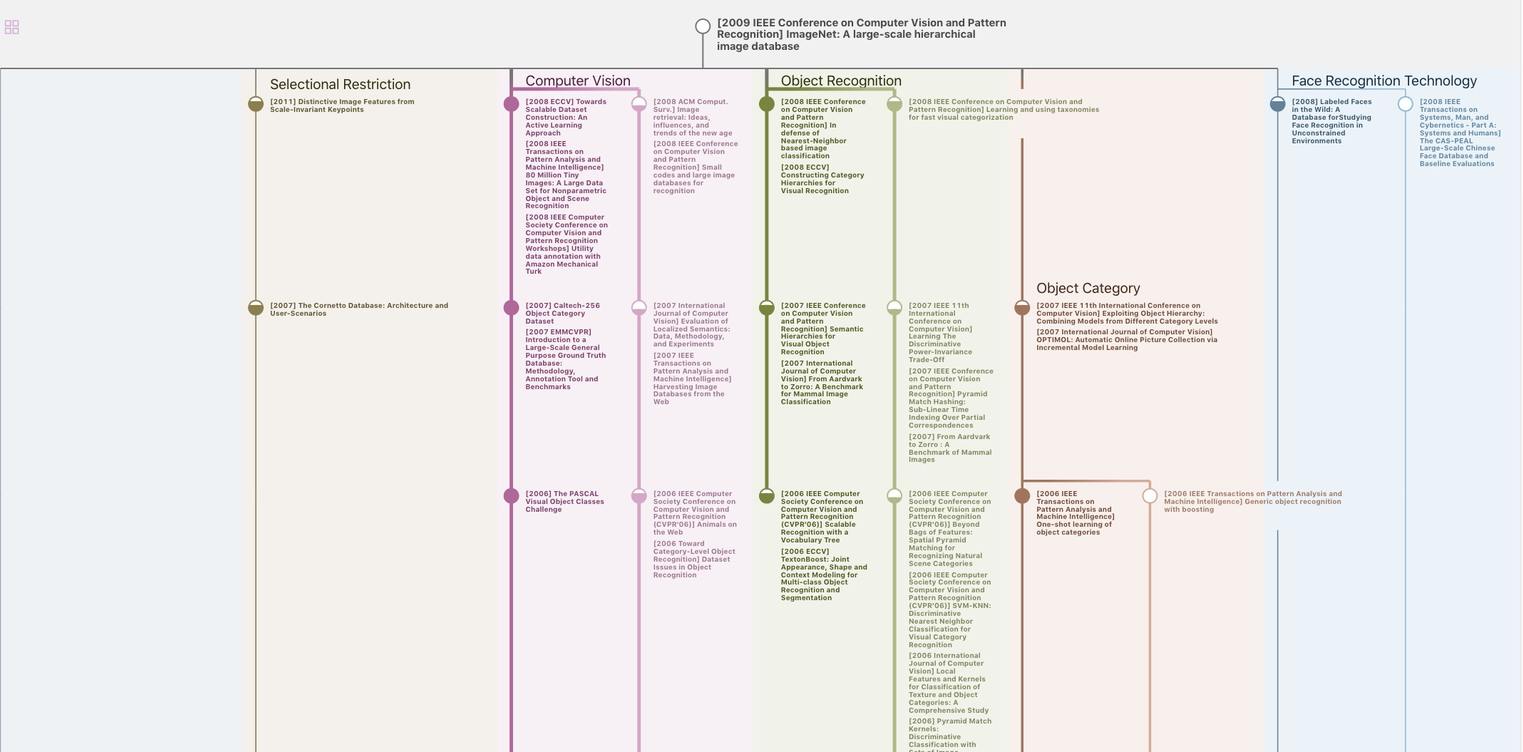
生成溯源树,研究论文发展脉络
Chat Paper
正在生成论文摘要