Importance Sampling Placement in Off-Policy Temporal-Difference Methods
arxiv(2022)
摘要
A central challenge to applying many off-policy reinforcement learning algorithms to real world problems is the variance introduced by importance sampling. In off-policy learning, the agent learns about a different policy than the one being executed. To account for the difference importance sampling ratios are often used, but can increase variance in the algorithms and reduce the rate of learning. Several variations of importance sampling have been proposed to reduce variance, with per-decision importance sampling being the most popular. However, the update rules for most off-policy algorithms in the literature depart from per-decision importance sampling in a subtle way; they correct the entire TD error instead of just the TD target. In this work, we show how this slight change can be interpreted as a control variate for the TD target, reducing variance and improving performance. Experiments over a wide range of algorithms show this subtle modification results in improved performance.
更多查看译文
关键词
sampling,importance,off-policy,temporal-difference
AI 理解论文
溯源树
样例
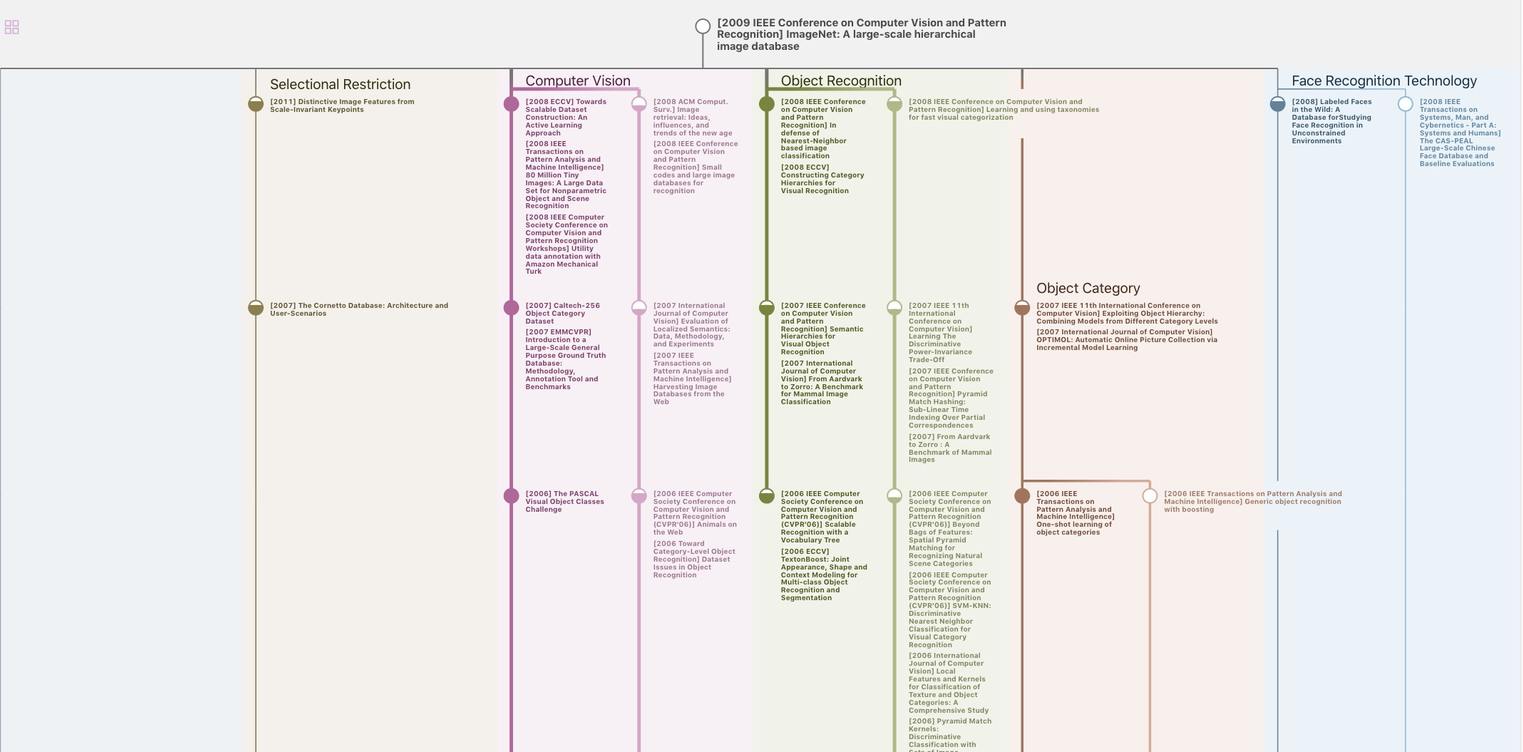
生成溯源树,研究论文发展脉络
Chat Paper
正在生成论文摘要