Acceleration control strategy for aero-engines based on model-free deep reinforcement learning method
Aerospace Science and Technology(2022)
摘要
Deep reinforcement learning has emerged as a powerful control method, especially for the complex nonlinear system such as aeroengine control system, due to its strong representation ability and capability of learning from data measurements. This paper presents a novel control strategy based on deep reinforcement learning to speed up the acceleration process of aero-engine. The actor-critic framework is adopted, where the actor neural network aims to find the optimal control policy and the critic network aims to evaluate the current control policy. The deep deterministic policy gradient algorithm is used to update the parameters of the neural networks. In addition, a complementary integrator is introduced to eliminate the steady-state error caused by the approximation error of the deep neural networks, and a momentum term is introduced to set limits for the input of the control system, thereby suppressing the overrun during the early learning and exploration processes. The numerical simulations show that the controller with this new control strategy can cope with different flight conditions, and significantly speed up the acceleration process of aero-engine.
更多查看译文
关键词
Reinforcement learning,Neural network,Aero-engine control,Nonlinear system
AI 理解论文
溯源树
样例
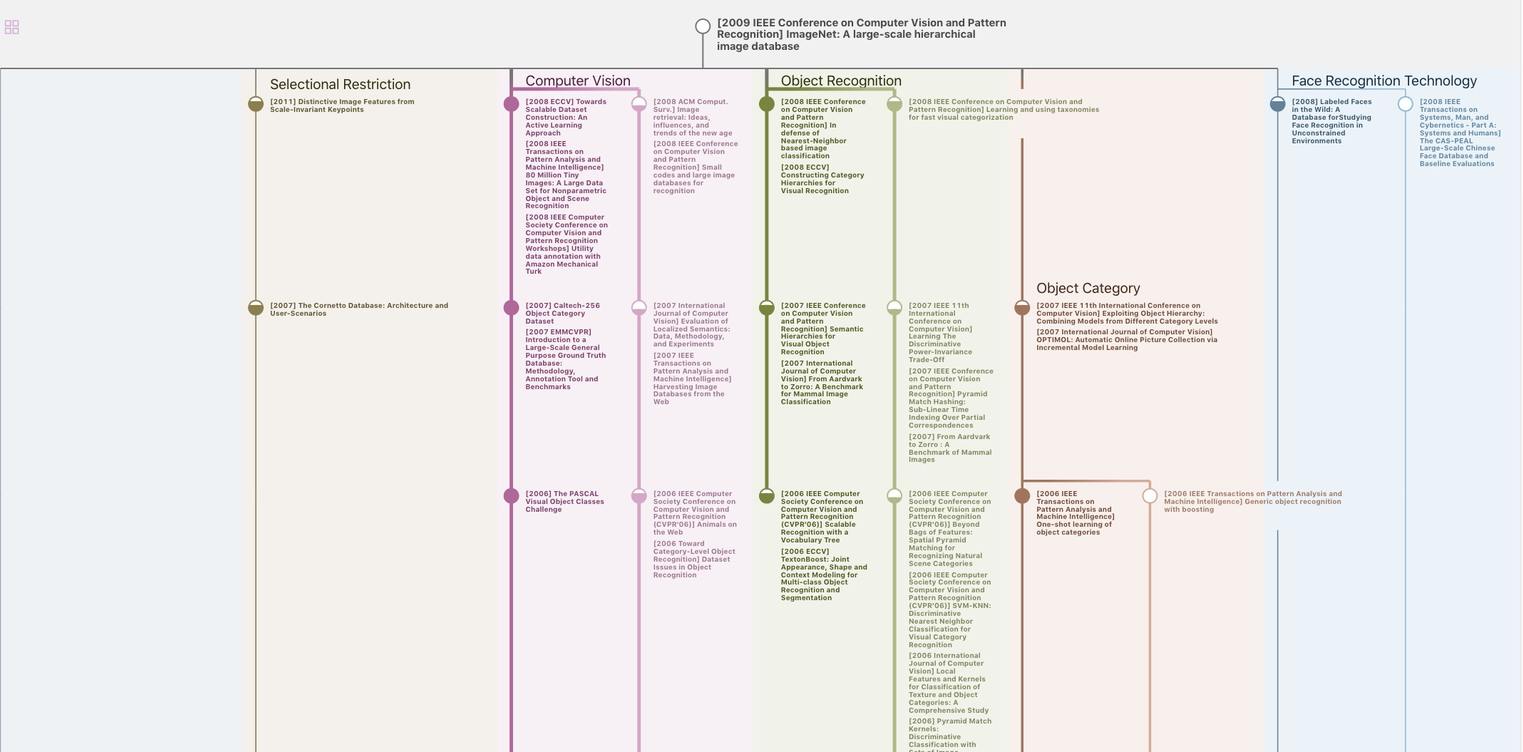
生成溯源树,研究论文发展脉络
Chat Paper
正在生成论文摘要