On the Expressive Power of Message-Passing Neural Networks as Global Feature Map Transformers.
International Symposium on Foundations of Information and Knowledge Systems (FoIKS)(2022)
摘要
We investigate the power of message-passing neural networks (MPNNs) in their capacity to transform the numerical features stored in the nodes of their input graphs. Our focus is on global expressive power, uniformly over all input graphs, or over graphs of bounded degree with features from a bounded domain. Accordingly, we introduce the notion of a global feature map transformer (GFMT). As a yardstick for expressiveness, we use a basic language for GFMTs, which we call MPLang. Every MPNN can be expressed in MPLang, and our results clarify to which extent the converse inclusion holds. We consider exact versus approximate expressiveness; the use of arbitrary activation functions; and the case where only the ReLU activation function is allowed.
更多查看译文
关键词
Closure under concatenation,Semiring provenance semantics for modal logic,Query languages for numerical data
AI 理解论文
溯源树
样例
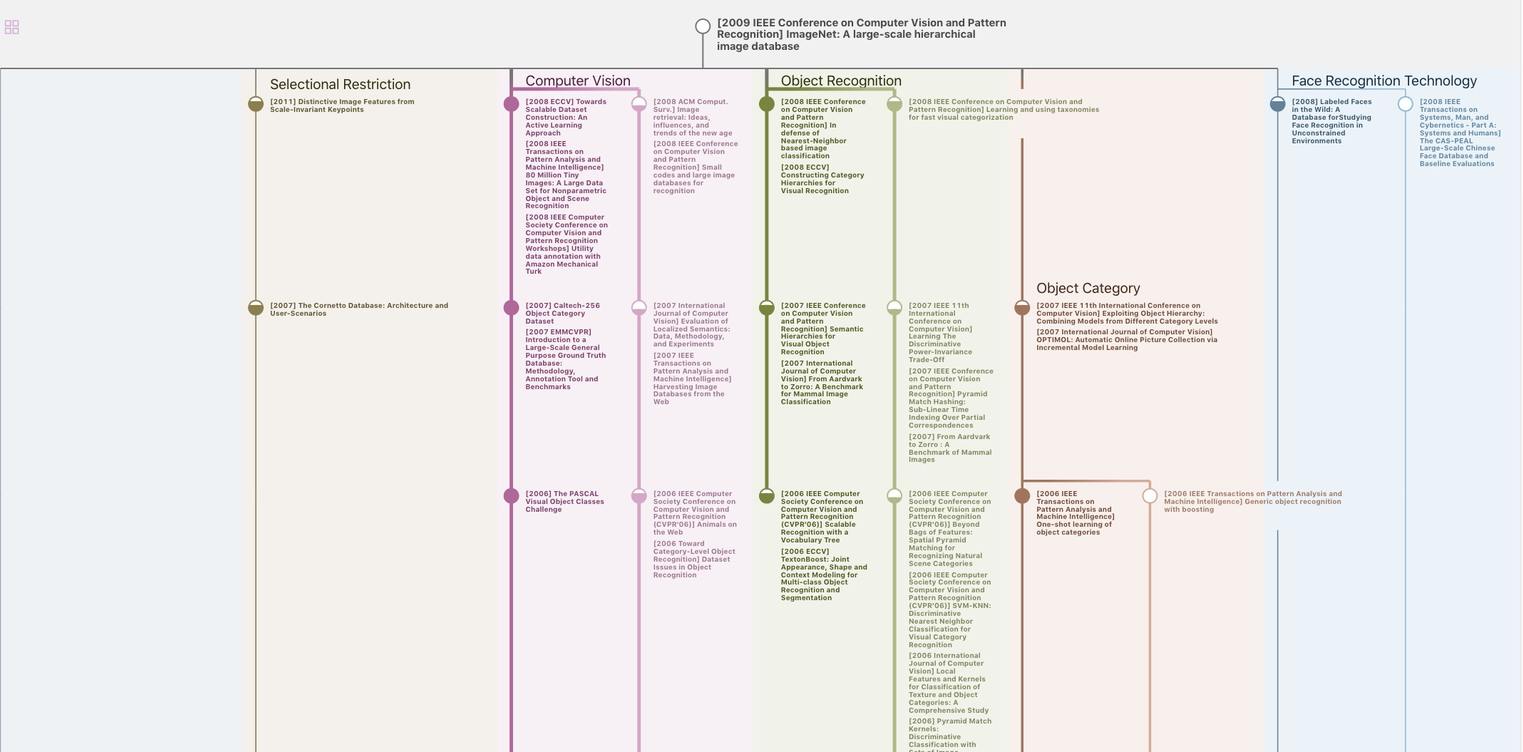
生成溯源树,研究论文发展脉络
Chat Paper
正在生成论文摘要