DeLA-Drug: A Deep Learning Algorithm for Automated Design of Druglike Analogues
JOURNAL OF CHEMICAL INFORMATION AND MODELING(2022)
摘要
In this paper, we present a deep learning algorithmfor automated design of druglike analogues (DeLA-Drug), arecurrent neural network (RNN) model composed of two longshort-term memory (LSTM) layers and conceived for data-drivengeneration of similar-to-bioactive compounds. DeLA-Drug cap-tures the syntax of SMILES strings of more than 1 millioncompounds belonging to the ChEMBL28 database and, byemploying a new strategy called sampling with substitutions(SWS), generates molecules starting from a single user-definedquery compound. Remarkably, the algorithm preserves druglike-ness and synthetic accessibility of the known bioactive compoundspresent in the ChEMBL28 repository. The absence of any time-demandingfine-tuning procedure enables DeLA-Drug to perform a fast generation of focused libraries for further high-throughputscreening and makes it a suitable tool for performingde novodesign even in low-data regimes. To provide a concrete idea of itsapplicability, DeLA-Drug was applied to the cannabinoid receptor subtype 2 (CB2R), a known target involved in differentpathological conditions such as cancer and neurodegeneration. DeLA-Drug, available as a free web platform (http://www.ba.ic.cnr.it/softwareic/deladrugportal/), can help medicinal chemists interested in generating analogues of compounds already available intheir laboratories and, for this reason, good candidates for an easy and low-cost synthesis.
更多查看译文
AI 理解论文
溯源树
样例
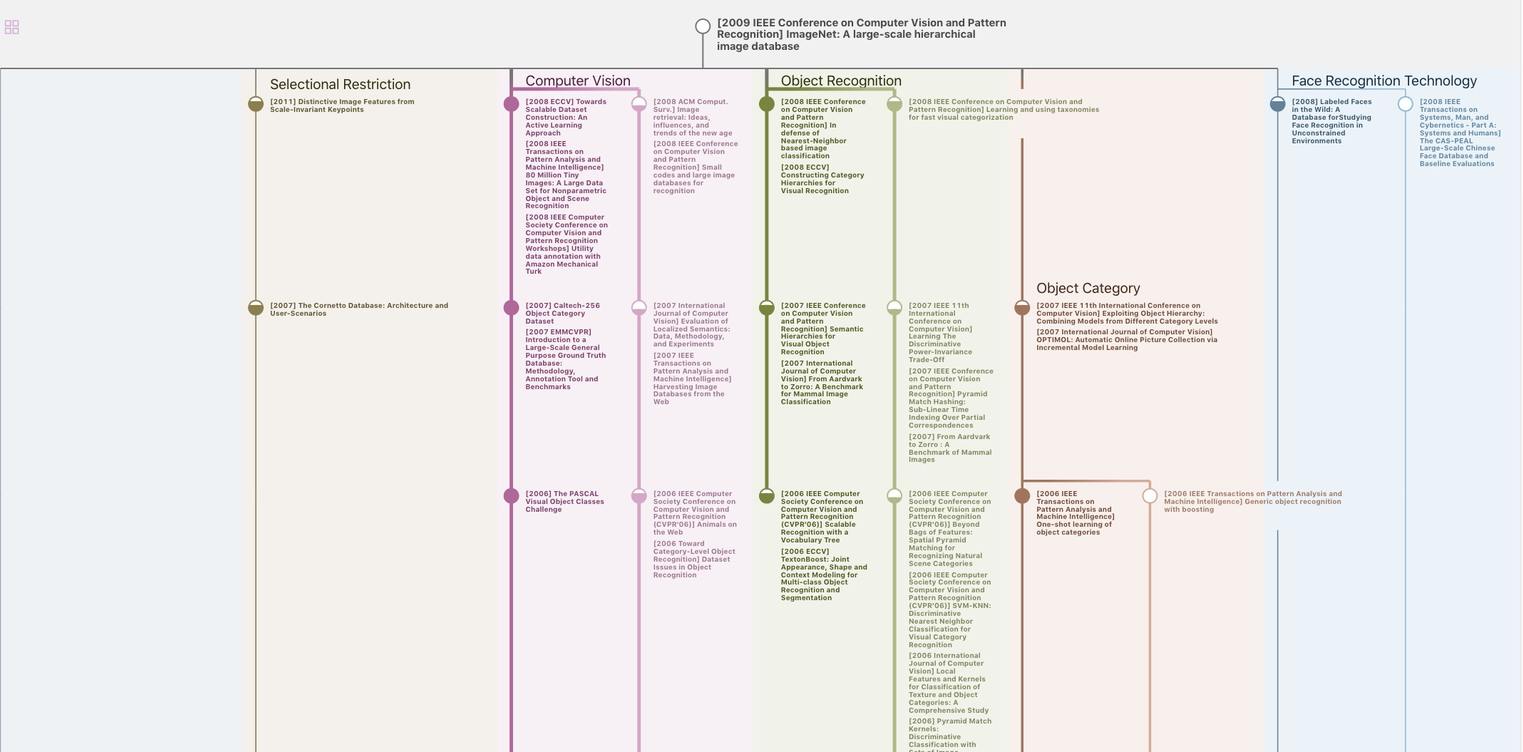
生成溯源树,研究论文发展脉络
Chat Paper
正在生成论文摘要