One-Step Weighting to Generalize and Transport Treatment Effect Estimates to a Target Population
AMERICAN STATISTICIAN(2023)
摘要
The problems of generalization and transportation of treatment effect estimates from a study sample to a target population are central to empirical research and statistical methodology. In both randomized experiments and observational studies, weighting methods are often used with this objective. Traditional methods construct the weights by separately modeling the treatment assignment and study selection probabilities and then multiplying functions (e.g., inverses) of their estimates. In this work, we provide a justification and an implementation for weighting in a single step. We show a formal connection between this one-step method and inverse probability and inverse odds weighting. We demonstrate that the resulting estimator for the target average treatment effect is consistent, asymptotically Normal, multiply robust, and semiparametrically efficient. We evaluate the performance of the one-step estimator in a simulation study. We illustrate its use in a case study on the effects of physician racial diversity on preventive healthcare utilization among Black men in California. We provide R code implementing the methodology.
更多查看译文
关键词
Causal inference,Generalization,Observational studies,Randomized experiments,Transportation,Weighting methods
AI 理解论文
溯源树
样例
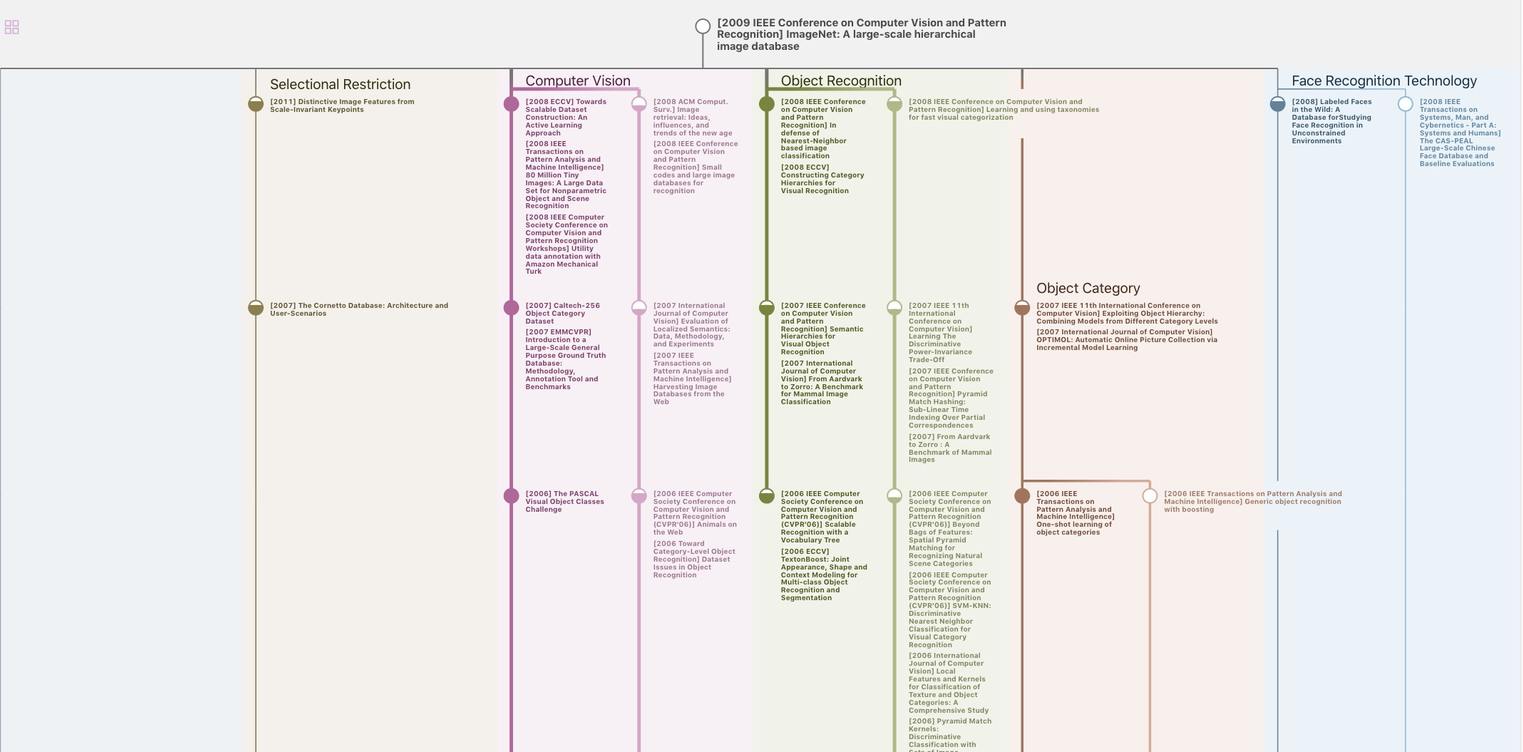
生成溯源树,研究论文发展脉络
Chat Paper
正在生成论文摘要