A Linearly Convergent Douglas-Rachford Splitting Solver for Markovian Information-Theoretic Optimization Problems
arxiv(2022)
摘要
In this work, we propose solving the Information bottleneck (IB) and Privacy Funnel (PF) problems with Douglas-Rachford Splitting methods (DRS). We study a general Markovian information-theoretic Lagrangian that includes IB and PF into a unified framework. We prove the linear convergence of the proposed solvers using the Kurdyka-{\L}ojasiewicz inequality. Moreover, our analysis is beyond IB and PF and applies to any convex-weakly convex pair objectives. Based on the results, we develop two types of linearly convergent IB solvers, with one improves the performance of convergence over existing solvers while the other can be independent to the relevance-compression trade-off. Moreover, our results apply to PF, yielding a new class of linearly convergent PF solvers. Empirically, the proposed IB solvers IB obtain solutions that are comparable to the Blahut-Arimoto-based benchmark and is convergent for a wider range of the penalty coefficient than existing solvers. For PF, our non-greedy solvers can characterize the privacy-utility trade-off better than the clustering-based greedy solvers.
更多查看译文
关键词
Mutual information,information bottleneck (IB),privacy funnel (PF),source coding,convergence,iterative algorithm,optimization methods,gradient methods,Lagrangian functions
AI 理解论文
溯源树
样例
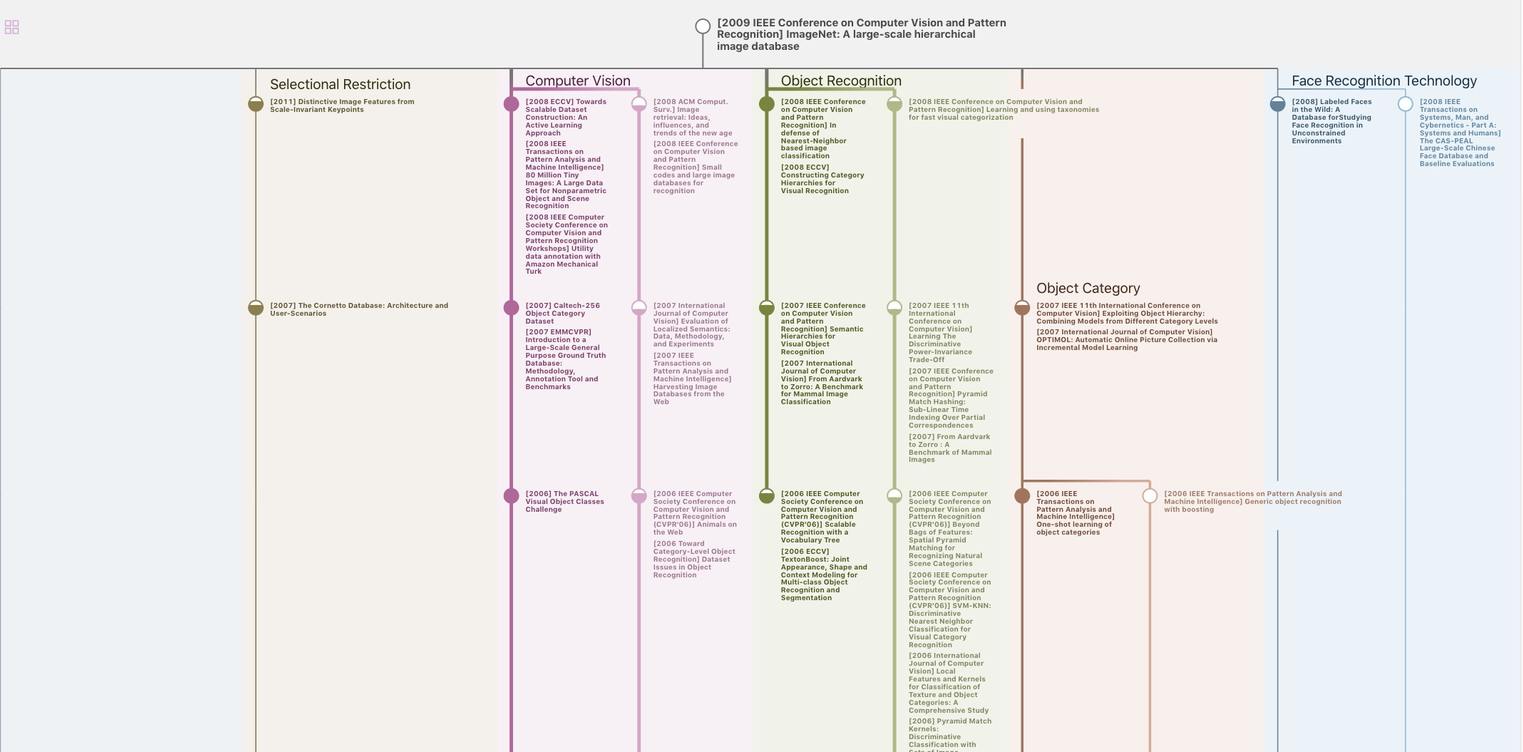
生成溯源树,研究论文发展脉络
Chat Paper
正在生成论文摘要