A comparative study of Deep Learning architectures for Classification of Natural and Human-made Sea Events in SAR images
Discover Artificial Intelligence(2022)
摘要
Sea monitoring is essential for a better understanding of its dynamics and to measure the impact of human activities. In this context, remote sensing plays an important role by providing satellite imagery every day, even in critical climate conditions, for the detection of sea events with a potential risk to the environment. The present work proposes a comparative study of Deep Learning architectures for classification of natural and man-made sea events using SAR imagery. The evaluated architectures comprises models based on convolutional networks, inception blocks, and attention modules. Two datasets are employed for this purpose: the first one encompasses a series of natural events (geophysical phenomena), while the second describes a real oil spill scenario in the Gulf of Mexico from 2018 to 2021. As a result, through experimental analysis, it is demonstrated how the Xception and Deep Attention sampling architectures obtained the highest performance metrics, presenting Recall values of 94.2% and 87.4% for the classification of natural and human-made events, respectively.
更多查看译文
关键词
Deep learning,Remote sensing,Maritime events classification,Sentinel-1A,Convolutional neural networks
AI 理解论文
溯源树
样例
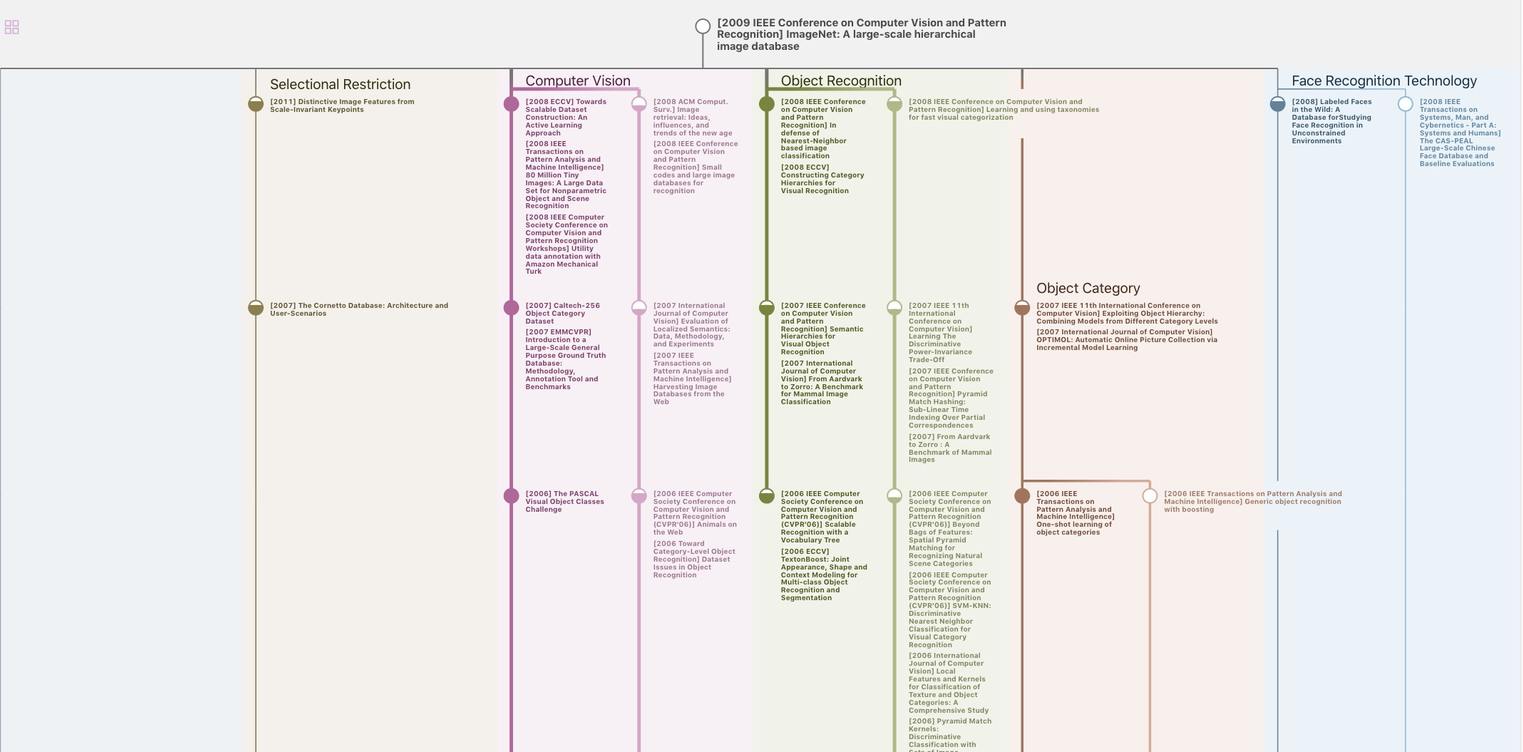
生成溯源树,研究论文发展脉络
Chat Paper
正在生成论文摘要