CancerBERT: a cancer domain-specific language model for extracting breast cancer phenotypes from electronic health records
JOURNAL OF THE AMERICAN MEDICAL INFORMATICS ASSOCIATION(2022)
摘要
Objective Accurate extraction of breast cancer patients' phenotypes is important for clinical decision support and clinical research. This study developed and evaluated cancer domain pretrained CancerBERT models for extracting breast cancer phenotypes from clinical texts. We also investigated the effect of customized cancer-related vocabulary on the performance of CancerBERT models. Materials and Methods A cancer-related corpus of breast cancer patients was extracted from the electronic health records of a local hospital. We annotated named entities in 200 pathology reports and 50 clinical notes for 8 cancer phenotypes for fine-tuning and evaluation. We kept pretraining the BlueBERT model on the cancer corpus with expanded vocabularies (using both term frequency-based and manually reviewed methods) to obtain CancerBERT models. The CancerBERT models were evaluated and compared with other baseline models on the cancer phenotype extraction task. Results All CancerBERT models outperformed all other models on the cancer phenotyping NER task. Both CancerBERT models with customized vocabularies outperformed the CancerBERT with the original BERT vocabulary. The CancerBERT model with manually reviewed customized vocabulary achieved the best performance with macro F1 scores equal to 0.876 (95% CI, 0.873-0.879) and 0.904 (95% CI, 0.902-0.906) for exact match and lenient match, respectively. Conclusions The CancerBERT models were developed to extract the cancer phenotypes in clinical notes and pathology reports. The results validated that using customized vocabulary may further improve the performances of domain specific BERT models in clinical NLP tasks. The CancerBERT models developed in the study would further help clinical decision support.
更多查看译文
关键词
natural language processing,CancerBERT,cancer phenotyping,electronic health record,name entity recognition
AI 理解论文
溯源树
样例
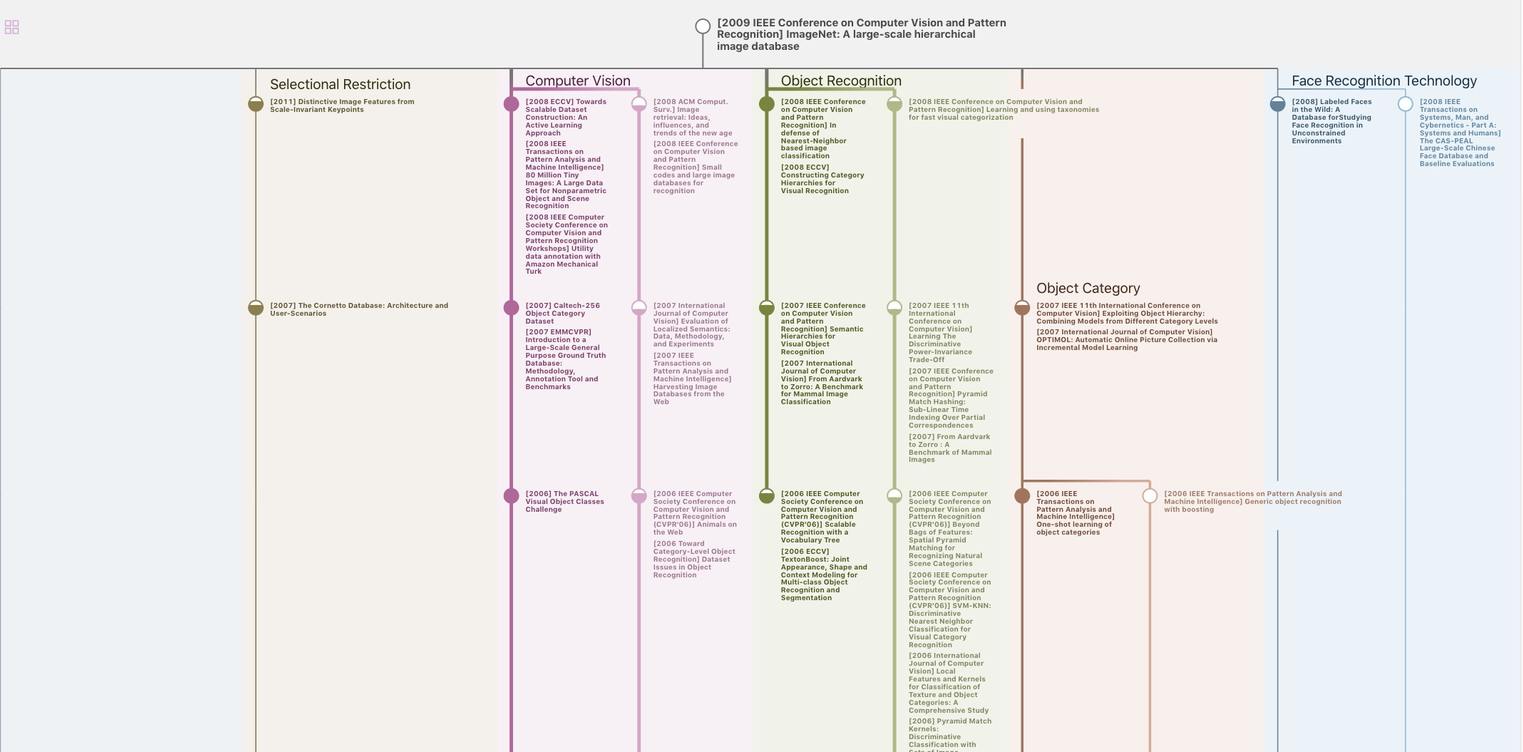
生成溯源树,研究论文发展脉络
Chat Paper
正在生成论文摘要