Semantic Segmentation (U-Net) of Archaeological Features in Airborne Laser Scanning-Example of the Bialowieza Forest
REMOTE SENSING(2022)
摘要
Airborne Laser Scanning (ALS) technology can be used to identify features of terrain relief in forested areas, possibly leading to the discovery of previously unknown archaeological monuments. Spatial interpretation of numerous objects with various shapes and sizes is a difficult challenge for archaeologists. Mapping structures with multiple elements whose area can exceed dozens of hectares, such as ancient agricultural field systems, is very time-consuming. These archaeological sites are composed of a large number of embanked fields, which together form a recognizable spatial pattern. Image classification and segmentation, as well as object recognition, are the most important tasks for deep learning neural networks (DLNN) and therefore they can be used for automatic recognition of archaeological monuments. In this study, a U-Net neural network was implemented to perform semantic segmentation of the ALS-derived data including (1) archaeological, (2) natural and (3) modern features in the Polish part of the Bialowieza Forest. The performance of the U-Net segmentation model was evaluated by measuring the pixel-wise similarity between ground truth and predicted segmentation masks. After 83 epochs, The Dice-Sorensen coefficient (F1 score) and the Intersect Over Union (IoU) metrics were 0.58 and 0.5, respectively. The IoU metric reached a value of 0.41, 0.62 and 0.62 for the ancient field system banks, ancient field system plots and burial mounds, respectively. The results of the U-Net deep learning model proved very useful in semantic segmentation of images derived from ALS data.
更多查看译文
关键词
deep neural networks,convolutional neural network,U-Net,ancient field systems,Celtic fields,Polish part of the Bialowieza Forest,UNESCO World Heritage Site,LiDAR,ALS,DTM
AI 理解论文
溯源树
样例
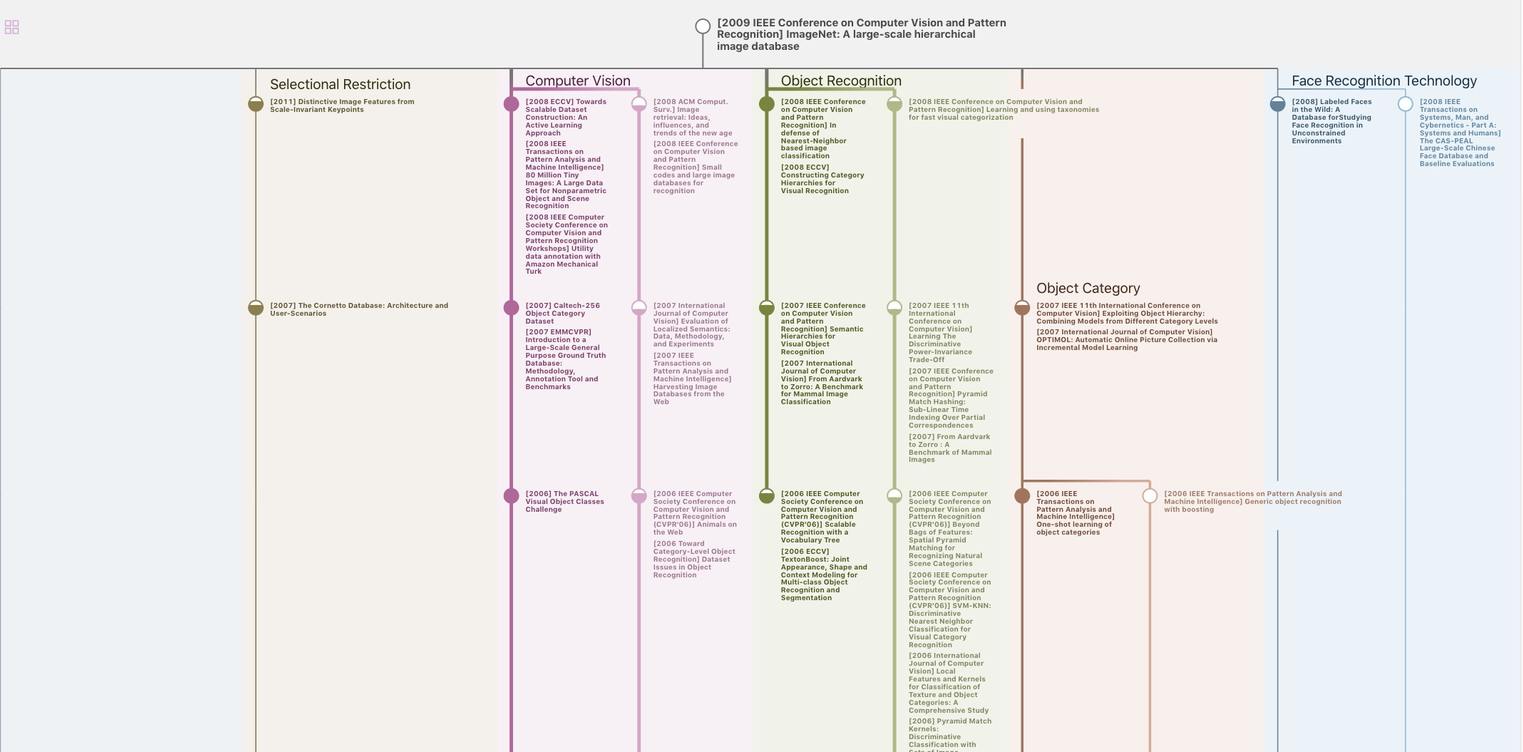
生成溯源树,研究论文发展脉络
Chat Paper
正在生成论文摘要